## Interactive Image Generation via Generative Adversarial Networks
<img src='pics/demo.gif' width=320>
<img src='pics/demo_teaser.jpg' width=800>
Given a few user strokes, our system could produce photo-realistic samples that best satisfy the user edits in real-time. Our system is based on deep generative models such as Generative Adversarial Networks ([GAN](https://arxiv.org/abs/1406.2661)) and [DCGAN](https://github.com/Newmu/dcgan_code). The system serves the following two purposes:
* An intelligent drawing interface for automatically generating images inspired by the color and shape of the brush strokes.
* An interactive visual debugging tool for understanding and visualizing deep generative models. By interacting with the generative model, a developer can understand what visual content the model can produce, as well as the limitation of the model.
Please cite our paper if you find this code useful in your research. (Contact: Jun-Yan Zhu, junyanz at mit dot edu)
## Getting started
* Install the python libraries. (See [Requirements](#requirements)).
* Download the code
* Download the model. (See `Model Zoo` for details):
``` bash
bash ./models/scripts/download_dcgan_model.sh outdoor_64
```
* Run the python script:
``` bash
THEANO_FLAGS='device=gpu0, floatX=float32, nvcc.fastmath=True' python iGAN_main.py --model_name outdoor_64
```
## Requirements
The code is written in Python2 and requires the following 3rd party libraries:
* numpy
* [OpenCV](http://opencv.org/)
```bash
sudo apt-get install python-opencv
```
* [Theano](https://github.com/Theano/Theano)
```bash
sudo pip install --upgrade --no-deps git+git://github.com/Theano/Theano.git
```
* [PyQt4](https://wiki.python.org/moin/PyQt4): more details on Qt installation can be found [here](http://www.saltycrane.com/blog/2008/01/how-to-install-pyqt4-on-ubuntu-linux/)
```bash
sudo apt-get install python-qt4
```
* [Qdarkstyle](https://github.com/ColinDuquesnoy/QDarkStyleSheet)
```bash
sudo pip install qdarkstyle
```
* [dominate](https://github.com/Knio/dominate)
```bash
sudo pip install dominate
```
* GPU + CUDA + cuDNN:
The code is tested on GTX Titan X + CUDA 7.5 + cuDNN 5. Here are the tutorials on how to install [CUDA](http://www.r-tutor.com/gpu-computing/cuda-installation/cuda7.5-ubuntu) and [cuDNN](http://askubuntu.com/questions/767269/how-can-i-install-cudnn-on-ubuntu-16-04). A decent GPU is required to run the system in real-time. [**Warning**] If you run the program on a GPU server, you need to use remote desktop software (e.g., VNC), which may introduce display artifacts and latency problem.
## Python3
For `Python3` users, you need to replace `pip` with `pip3`:
* PyQt4 with Python3:
``` bash
sudo apt-get install python3-pyqt4
```
* OpenCV3 with Python3: see the installation [instruction](http://www.pyimagesearch.com/2015/07/20/install-opencv-3-0-and-python-3-4-on-ubuntu/).
## Interface:
<img src='pics/ui_intro.jpg' width=800>
#### Layout
* Drawing Pad: This is the main window of our interface. A user can apply different edits via our brush tools, and the system will display the generated image. Check/Uncheck `Edits` button to display/hide user edits.
* Candidate Results: a display showing thumbnails of all the candidate results (e.g., different modes) that fits the user edits. A user can click a mode (highlighted by a green rectangle), and the drawing pad will show this result.
* Brush Tools: `Coloring Brush` for changing the color of a specific region; `Sketching brush` for outlining the shape. `Warping brush` for modifying the shape more explicitly.
* Slider Bar: drag the slider bar to explore the interpolation sequence between the initial result (i.e., randomly generated image) and the current result (e.g., image that satisfies the user edits).
* Control Panel: `Play`: play the interpolation sequence; `Fix`: use the current result as additional constraints for further editing `Restart`: restart the system; `Save`: save the result to a webpage. `Edits`: Check the box if you would like to show the edits on top of the generated image.
#### User interaction
* `Coloring Brush`: right-click to select a color; hold left click to paint; scroll the mouse wheel to adjust the width of the brush.
* `Sketching Brush`: hold left-click to sketch the shape.
* `Warping Brush`: We recommend you first use coloring and sketching before the warping brush. Right-click to select a square region; hold left click to drag the region; scroll the mouse wheel to adjust the size of the square region.
* Shortcuts: P for `Play`, F for `Fix`, R for `Restart`; S for `Save`; E for `Edits`; Q for quitting the program.
* Tooltips: when you move the cursor over a button, the system will display the tooltip of the button.
## Model Zoo:
Download the Theano DCGAN model (e.g., outdoor_64). Before using our system, please check out the random real images vs. DCGAN generated samples to see which kind of images that a model can produce.
``` bash
bash ./models/scripts/download_dcgan_model.sh outdoor_64
```
* [ourdoor_64.dcgan_theano](http://efrosgans.eecs.berkeley.edu/iGAN/models/theano_dcgan/outdoor_64.dcgan_theano) (64x64): trained on 150K landscape images from MIT [Places](http://places.csail.mit.edu/) dataset [[Real](http://efrosgans.eecs.berkeley.edu/iGAN/samples/outdoor_64_real.png) vs. [DCGAN](http://efrosgans.eecs.berkeley.edu/iGAN/samples/outdoor_64_dcgan.png)].
* [church_64.dcgan_theano](http://efrosgans.eecs.berkeley.edu/iGAN/models/theano_dcgan/church_64.dcgan_theano) (64x64): trained on 126k church images from the [LSUN](http://lsun.cs.princeton.edu/2016/) challenge [[Real](http://efrosgans.eecs.berkeley.edu/iGAN/samples/church_64_real.png) vs. [DCGAN](http://efrosgans.eecs.berkeley.edu/iGAN/samples/church_64_dcgan.png)].
* [handbag_64.dcgan_theano](http://efrosgans.eecs.berkeley.edu/iGAN/models/theano_dcgan/handbag_64.dcgan_theano) (64x64): trained on 137K handbag images downloaded from Amazon [[Real](http://efrosgans.eecs.berkeley.edu/iGAN/samples/handbag_64_real.png) vs. [DCGAN](http://efrosgans.eecs.berkeley.edu/iGAN/samples/handbag_64_dcgan.png)].
* [shoes_64.dcgan_theano](http://efrosgans.eecs.berkeley.edu/iGAN/models/theano_dcgan/shoes_64.dcgan_theano) (64x64): trained on 50K shoes images collected by [Yu and Grauman](http://vision.cs.utexas.edu/projects/finegrained/utzap50k/) [[Real](http://efrosgans.eecs.berkeley.edu/iGAN/samples/shoes_64_real.png) vs. [DCGAN](http://efrosgans.eecs.berkeley.edu/iGAN/samples/shoes_64_dcgan.png)].
* [hed_shoes_64.dcgan_theano](http://efrosgans.eecs.berkeley.edu/iGAN/models/theano_dcgan/hed_shoes_64.dcgan_theano) (64x64): trained on 50K shoes sketches (computed by [HED](https://github.com/s9xie/hed)) [[Real](http://efrosgans.eecs.berkeley.edu/iGAN/samples/hed_shoes_64_real.png) vs. [DCGAN](http://efrosgans.eecs.berkeley.edu/iGAN/samples/hed_shoes_64_dcgan.png)]. (Use this model with `--shadow` flag)
We provide a simple script to generate samples from a pre-trained DCGAN model. You can run this script to test if Theano, CUDA, cuDNN are configured properly before running our interface.
```bash
THEANO_FLAGS='device=gpu0, floatX=float32, nvcc.fastmath=True' python generate_samples.py --model_name outdoor_64 --output_image outdoor_64_dcgan.png
```
## Command line arguments:
Type `python iGAN_main.py --help` for a complete list of the arguments. Here we discuss some important arguments:
* `--model_name`: the name of the model (e.g., outdoor_64, shoes_64, etc.)
* `--model_type`: currently only supports dcgan_theano.
* `--model_file`: the file that stores the generative model; If not specified, `model_file='./models/%s.%s' % (model_name, model_type)`
* `--top_k`: the number of the candidate results being displayed
* `--average`: show an average image in the main window. Inspired by [AverageExplorer](https://www.cs.cmu.edu/~junyanz/projects/averageExplorer/), average image is a weighted average of multiple generated results, with the weights reflecting user-indi

__AtYou__
- 粉丝: 3513
- 资源: 2177
最新资源
- 苹果手机外壳贴麦拉机组装流道(sw16可编辑+工程图+bom)全套技术资料100%好用.zip
- MATLAB 实现基于PCNN(脉冲耦合神经网络)进行时间序列预测模型的项目详细实例(含完整的程序,GUI设计和代码详解)
- MATLAB 实现基于麻雀搜索算法(SSA)进行时间序列预测模型的项目详细实例(含完整的程序,GUI设计和代码详解)
- 基于改进剪枝算法的接触熟虾图像分割技术及其质量测量应用
- 音乐爬虫python源码分享
- MATLAB 实现基于移动平均模型(MA)进行时间序列预测模型的项目详细实例(含完整的程序,GUI设计和代码详解)
- Matlab实现基于NNMF+DBO+K-Medoids的数据聚类可视化的详细项目实例(含完整的程序,GUI设计和代码详解)
- 基于SSM的球鞋交易管理平台论文+Java-HTML+球鞋交易平台+毕设-课设均可
- 计算机视觉中高效曲线检测算法的研究与应用-随机化Hough变换的新方法实现线条、圆及椭圆识别
- Matlab基于ALO-SVR蚁狮优化支持向量回归的锂离子电池剩余寿命预测的详细项目实例(含完整的程序,GUI设计和代码详解)
- Matlab实现BiTCN双向时间卷积神经网络多变量时间序列预测的详细项目实例(含完整的程序,GUI设计和代码详解)
- 医学图像中基于判别广义霍夫变换的目标定位方法研究与应用
- 华强北悦虎耳机刷机固件包
- Matlab实现RIME-BP霜冰算法优化BP神经网络多变量回归预测的详细项目实例(含完整的程序,GUI设计和代码详解)
- 桌面作图软件CAD字体库
- MATLAB 实现基于VMD(变分模态分解)进行时间序列预测模型的项目详细实例(含完整的程序,GUI设计和代码详解)
资源上传下载、课程学习等过程中有任何疑问或建议,欢迎提出宝贵意见哦~我们会及时处理!
点击此处反馈


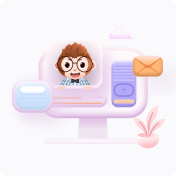