# Partial-Computation-Offloading-For-MEC
Optimization of delay for partial computing task offloading based on deep reinforcement learning
(基于深度强化学习的部分计算任务卸载延迟优化)
<img src="resources/fig1.png"/>
This repository provides the official Tensorflow implementation for the following paper:
**Intelligent Delay-Aware Partial Computing Task Offloading for Multi-User Industrial Internet of Things through Edge Computing**
> ***Abstract***: The development of Industrial Internet of Things (IIoT) and Industry 4.0 has completely changed the traditional manufacturing industry. Intelligent IIoT technology usually involves a large number of intensive computing tasks. Resource-constrained IIoT devices often cannot meet the real-time requirements of these tasks. As a promising paradigm, Mobile Edge Computing (MEC) system migrates the computation intensive tasks from resource-constrained IIoT devices to nearby MEC servers, thereby obtaining lower delay and energy consumption. However, considering the varying channel conditions as well as the distinct delay requirements for various computing tasks, it is challenging to coordinate the computing task offloading among multiple users. In this paper, we propose an autonomous partial offloading system for delay sensitive computation tasks in multi-user IIoT MEC systems. Our goal is to provide offloading services with minimum delay for better Quality of Service (QoS). Enlighten by the recent advancement of Reinforcement Learning (RL), we propose two RL based offloading strategies to automatically optimize the delay performance. Specifically, we first implement Q-learning algorithm to provide a discrete partial offloading decision. Then, to further optimize the system performance with more flexible task offloading, the offloading decisions are given as continuous based on Deep Deterministic Policy Gradient (DDPG). The simulation results show that, the Q-learning scheme reduces the delay by 23%, and the DDPG scheme reduces the delay by 30%.
## Method
The overall architecture of our method:
<img src="resources/fig2.png"/>
## Citation
If you find our work helpful to your research, please cite our paper:
```
@article{deng2021intelligent,
title={Intelligent Delay-Aware Partial Computing Task Offloading for Multi-User Industrial Internet of Things through Edge Computing},
author={Deng, Xiaoheng and Yin, Jian and Guan, Peiyuan and Xiong, Neal N and Zhang, Lan and Mumtaz, Shahid},
journal={IEEE Internet of Things Journal},
year={2021},
publisher={IEEE}
}
```

程序员张小妍
- 粉丝: 1w+
- 资源: 3704
最新资源
- SoundManager.java
- 永磁同步电机参数调整与预测控制模块:时变工况下的参数不确定性与匹配策略,永磁同步电机参数调整与预测控制模块:时变工况下的参数不确定性与匹配策略,永磁同步电机参数不确定 参数变化 参数失配 参数摄动模块
- C++基础入门.pdf
- 软考-中级-网络工程师-知识点总结.docx
- ,《基于速度同步线性调频小波变换(VSLCT)的时频分析技术研究与应用》,速度同步线性调频小波变(VSLCT)VSLCT是当前线性变的扩展版本 它能有效地缓解拖尾效应,并能动态地响应条件变化提供所需
- ROS工程认知-ROS文件系统结构与编译系统详解
- 两极式三相光伏逆变并网仿真:基于MPPT算法与双环PI调节的LCL型三相逆变系统仿真模型,三相光伏并网系统仿真模型:研究Boost变换器MPPT算法与三相两电平LCL型逆变器的SVPWM调制及控制策略
- ROS环境中C++节点的构建与多节点协调运行机制探讨
- ROS通讯机制-话题通信详解及实例:构建发布/订阅模式实现异步通信
- 优化后的PFC2D颗粒离散元数值模拟试验合集:直剪、单轴与双轴压缩并行高效运行代码集,优化后PFC2D颗粒离散元数值模拟试验合集:高效单直剪与单双轴压缩并行运行代码集,该模型是一个PFC2D颗粒离散元
- 第五次课程服务组实验1:基于仿真的机器人及其应用环境
- 【business-jxd】
- 第6次课 ROS服务.docx
- Abaqus铁路轨道建模及车轨耦合动力响应分析:车轨地基相互作用与弹簧批量施加研究,Abaqus铁路轨道建模及车轨耦合动力响应分析:车轨地基相互作用与弹簧批量施加研究,Abaqus铁路轨道建模,车轨耦
- PCAN-Basic-Windows.zip
- 从0到1的网络安全专家之路 二进制安全和Web安全.zip
资源上传下载、课程学习等过程中有任何疑问或建议,欢迎提出宝贵意见哦~我们会及时处理!
点击此处反馈


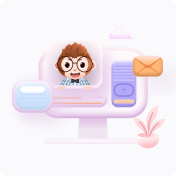