#!/usr/bin/python3.7
# -*- coding: utf-8 -*-
# @Time : 2020/10/20 15:12
# @Author : dly
# @File : Main.py
# @Desc :
import pandas as pd
import numpy as np
import os
import seaborn as sns
import matplotlib.pyplot as plt
from sklearn import metrics
from sklearn.model_selection import KFold, train_test_split, cross_val_score
import lightgbm as lgb
from bayes_opt import BayesianOptimization
# 调整数据类型,减少内存占用
def reduce_mem_usage(df):
start_mem = df.memory_usage().sum() / 1024 ** 2
print('Memory usage of df is {:.2f} MB'.format(start_mem))
for col in df.columns:
col_type = df[col].dtype
if col_type != object:
c_min = df[col].min()
c_max = df[col].max()
if str(col_type)[:3] == 'int':
if c_min > np.iinfo(np.int8).min and c_max < np.iinfo(np.int8).max:
df[col] = df[col].astype(np.int8)
elif c_min > np.iinfo(np.int16).min and c_max < np.iinfo(np.int16).max:
df[col] = df[col].astype(np.int16)
elif c_min > np.iinfo(np.int32).min and c_max < np.iinfo(np.int32).max:
df[col] = df[col].astype(np.int32)
elif c_min > np.iinfo(np.int64).min and c_max < np.iinfo(np.int64).max:
df[col] = df[col].astype(np.int64)
else:
if c_min > np.finfo(np.float16).min and c_max < np.finfo(np.float16).max:
df[col] = df[col].astype(np.float16)
elif c_min > np.finfo(np.float32).min and c_max < np.finfo(np.float32).max:
df[col] = df[col].astype(np.float32)
else:
df[col] = df[col].astype(np.float64)
else:
df[col] = df[col].astype('category')
end_mem = df.memory_usage().sum() / 1024 ** 2
print('Memory usage of df is {:.2f} MB'.format(end_mem))
print('Decreased by {:.1f}%'.format(100 * (start_mem - end_mem) / start_mem))
return df
# 读取数据
data_path = 'C:/Users/Dooooooooo21/Desktop/project/FOUNDATION/'
train_all = pd.read_csv(data_path + 'train.csv')
test = pd.read_csv(data_path + 'testA.csv')
train_all = reduce_mem_usage(train_all)
test = reduce_mem_usage(test)
y_train = train_all['isDefault']
X_train = train_all.drop(['id', 'issueDate', 'isDefault', 'policyCode'], axis=1)
X_test = test.drop(['id', 'issueDate', 'policyCode'], axis=1)
def ori_lgb():
# 5折交叉验证
folds = 5
seed = 1001
kf = KFold(n_splits=folds, shuffle=True, random_state=seed)
cv_scores = []
for i, (train_index, valid_index) in enumerate(kf.split(X_train, y_train)):
print('*' * 20 + str(i + 1) + '*' * 20)
X_train_split, X_val, y_train_split, y_val = train_test_split(X_train, y_train, test_size=0.2)
train_matrix = lgb.Dataset(X_train_split, label=y_train_split)
valid_matrix = lgb.Dataset(X_val, y_val)
params = {
'boosting_type': 'gbdt',
'objective': 'binary',
'metric': 'auc',
'learning_rate': 0.01,
'num_leaves': 14,
'max_depth': 19,
'min_data_in_leaf': 37,
'min_child_weight': 1.6,
'bagging_fraction': 0.98,
'feature_fraction': 0.69,
'bagging_freq': 96,
'reg_lambda': 9,
'reg_alpha': 7,
'min_split_gain': 0.4,
'nthread': 8,
'seed': 2020,
}
model = lgb.train(params, train_set=train_matrix, valid_sets=valid_matrix, num_boost_round=14269,
verbose_eval=1000,
early_stopping_rounds=200)
val_pre_lgb = model.predict(X_val, num_iteration=model.best_iteration)
cv_scores.append(metrics.roc_auc_score(y_val, val_pre_lgb))
print(cv_scores)
print("lgb_scotrainre_list:{}".format(cv_scores))
print("lgb_score_mean:{}".format(np.mean(cv_scores)))
print("lgb_score_std:{}".format(np.std(cv_scores)))
def plot_roc(y_val, val_pre_lgb):
fpr, tpr, th = metrics.roc_curve(y_val, val_pre_lgb)
roc_auc = metrics.auc(fpr, tpr)
print('未调参lgb单模型在验证机上的AUC: {}'.format(roc_auc))
# 画roc
plt.figure(figsize=(8, 8))
plt.title('val roc')
plt.plot(fpr, tpr, 'b', label='Val auc = %0.4f' % roc_auc)
plt.xlim(0, 1)
plt.ylim(0, 1)
plt.legend(loc='best')
plt.xlabel('false positive rate')
plt.ylabel('true positive rate')
# 画对角线
plt.plot([0, 1], [0, 1], 'r--')
plt.show()
def rf_cv_lgb(num_leaves, max_depth, bagging_fraction, feature_fraction, bagging_freq, min_data_in_leaf,
min_child_weight, min_split_gain, reg_lambda, reg_alpha):
model_lgb = lgb.LGBMClassifier(boosting_type='gbdt', objective='binary', metrics='auc', learning_rate=0.1,
n_estimators=5000, num_leaves=int(num_leaves), max_depth=int(max_depth),
bagging_fraction=round(bagging_fraction, 2),
feature_fraction=round(feature_fraction, 2),
bagging_freq=int(bagging_freq), min_data_in_leaf=int(min_data_in_leaf),
min_child_weight=min_child_weight, min_split_gain=min_split_gain,
reg_lambda=reg_lambda, reg_alpha=reg_alpha, n_jobs=8)
val = cross_val_score(model_lgb, X_train_split, y_train_split, cv=5, scoring='roc_auc').mean()
return val
# 贝叶斯调参
def bayes():
bayes_lgb = BayesianOptimization(rf_cv_lgb, {
'num_leaves': (10, 200),
'max_depth': (3, 20),
'bagging_fraction': (0.5, 1.0),
'feature_fraction': (0.5, 1.0),
'bagging_freq': (0, 100),
'min_data_in_leaf': (10, 100),
'min_child_weight': (0, 10),
'min_split_gain': (0.0, 1.0),
'reg_alpha': (0.0, 10),
'reg_lambda': (0.0, 10),
})
bayes_lgb.maximize(n_iter=10)
# 最优参数
print(bayes_lgb.max)
# 确定最优的迭代次数
def it():
base_params_lgb = {'boosting_type': 'gbdt',
'objective': 'binary',
'metric': 'auc',
'learning_rate': 0.01,
'num_leaves': 200,
'max_depth': 3,
'min_data_in_leaf': 56,
'min_child_weight': 9.63,
'bagging_fraction': 1.0,
'feature_fraction': 1.0,
'bagging_freq': 100,
'reg_lambda': 10,
'reg_alpha': 1,
'min_split_gain': 1.0,
'nthread': 8,
'seed': 2020,
'verbose': -1, }
cv_result_lgb = lgb.cv(train_set=train_matrix, early_stopping_rounds=1000, num_boost_round=20000, nfold=5,
stratified=True, shuffle=True, params=base_params_lgb, metrics='auc', seed=0)
print('迭代次数{}'.format(len(cv_result_lgb['auc-mean'])))
print('最终模型的AUC为{}'.format(max(cv_result_lgb['auc-mean'])))
# 最终训练模型
X_train_split, X_val, y_train_split, y_val = train_test_split(X_train, y_train, test_size=0.2)
train_matrix = lgb.Dataset(X_train_split, label=y_train_split)
valid_matrix = lgb.Dataset(X_val, y_val)
params = {
'boosting_type': 'gbdt',
'objective': 'binary',
'metric': 'auc',
'learning_rate': 0.01,
'num_leaves': 14,
'max_depth': 19,
'min_data_in_leaf': 37,
'min_child_weight': 1.6,
'bagging_fraction': 0.98,
'feature_fraction': 0.69,
'bagging_freq': 96,
'reg_lambda': 9,
'reg_alpha': 7,
'min_split_gain': 0.4,
'nthread': 8,
'seed': 2020,
}
model = lgb.train(params, train_set=train_matrix, num_boost_round=3300,
verbose_eval=1000,
valid_sets=val
没有合适的资源?快使用搜索试试~ 我知道了~
温馨提示
课程大作业基于python实现的金融风控贷款违约预测源码(可参考借鉴).zip 个人经导师指导并认可通过的98分大作业设计项目,主要针对计算机相关专业的正在做课程设计、期末大作业的学生和需要项目实战练习的学习者。个人经导师指导并认可通过的98分大作业设计项目,主要针对计算机相关专业的正在做课程设计、期末大作业的学生和需要项目实战练习的学习者。个人经导师指导并认可通过的98分大作业设计项目,主要针对计算机相关专业的正在做课程设计、期末大作业的学生和需要项目实战练习的学习者。个人经导师指导并认可通过的98分大作业设计项目,主要针对计算机相关专业的正在做课程设计、期末大作业的学生和需要项目实战练习的学习者。个人经导师指导并认可通过的98分大作业设计项目,主要针对计算机相关专业的正在做课程设计、期末大作业的学生和需要项目实战练习的学习者。个人经导师指导并认可通过的98分大作业设计项目,主要针对计算机相关专业的正在做课程设计、期末大作业的学生和需要项目实战练习的学习者。个人经导师指导并认可通过的98分大作业设计项目,主要针对计算机相关专业的正在做课程设计、期末大作业的学生和需要项目实战练习
资源推荐
资源详情
资源评论
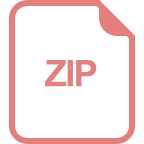
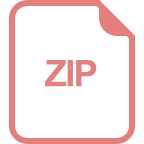
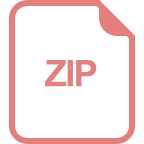
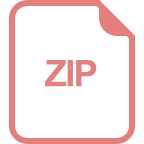
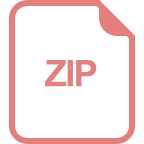
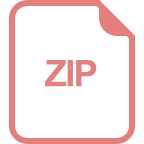
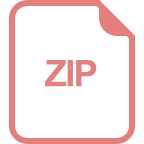
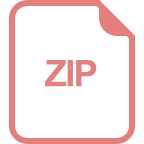
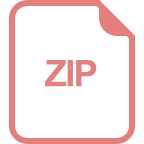
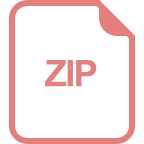
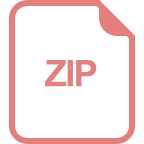
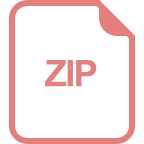
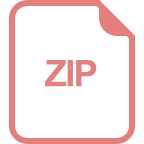
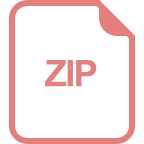
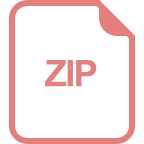
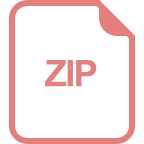
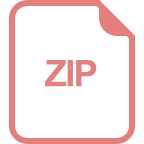
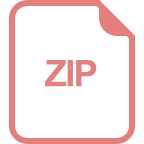
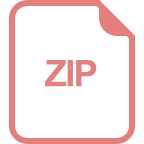
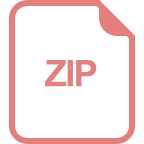
收起资源包目录


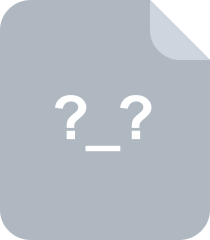
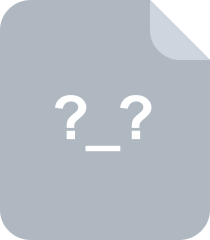
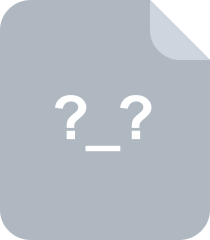
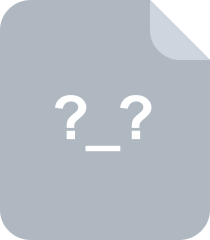
共 4 条
- 1
资源评论
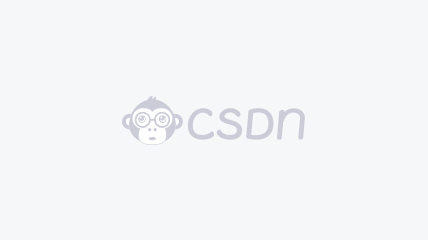

程序员张小妍
- 粉丝: 1w+
- 资源: 3252
上传资源 快速赚钱
我的内容管理 展开
我的资源 快来上传第一个资源
我的收益
登录查看自己的收益我的积分 登录查看自己的积分
我的C币 登录后查看C币余额
我的收藏
我的下载
下载帮助

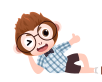
最新资源
- 全球干旱数据集【自校准帕尔默干旱程度指数scPDSI】-190101-202312-0.5x0.5
- 基于Python实现的VAE(变分自编码器)训练算法源代码+使用说明
- 全球干旱数据集【标准化降水蒸发指数SPEI-12】-190101-202312-0.5x0.5
- C语言小游戏-五子棋-详细代码可运行
- 全球干旱数据集【标准化降水蒸发指数SPEI-03】-190101-202312-0.5x0.5
- spring boot aop记录修改前后的值demo
- 全球干旱数据集【标准化降水蒸发指数SPEI-01】-190101-202312-0.5x0.5
- ActiveReports
- vgbvdsbnjkbfnb
- effsefefeffsfwfse
资源上传下载、课程学习等过程中有任何疑问或建议,欢迎提出宝贵意见哦~我们会及时处理!
点击此处反馈


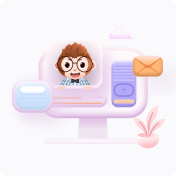
安全验证
文档复制为VIP权益,开通VIP直接复制
