"""
#-*-coding:utf-8-*-
# @anthor: wangyu a beginner programmer, striving to be the strongest.
# @date: 2021/7/20 14:48
"""
import torch.nn as nn
import torch
class BasicBlock(nn.Module):
# layer18/layer34
expansion = 1 # 对应各层的卷积核的个数是否改变,对于18和34层网络,相邻两层卷积核个数均为64
def __init__(self, in_channel, out_channel, stride=1, downsample=None):
"""
初始化函数
:param in_channel: 输入特征矩阵的深度
:param out_channel:输出特征矩阵的深度(卷积核的个数)
:param stride: 步长
:param downsample: 下采样,对应虚线处的残差结构,1x1的卷积层
"""
super(BasicBlock, self).__init__()
self.conv1 = nn.Conv2d(in_channels=in_channel,
out_channels=out_channel,
kernel_size=3, # 卷积核的大小
stride=stride,
padding=1, # 填充步长
bias=False,) # 偏置,使用BatchNormalization时不用偏置
self.bn1 = nn.BatchNorm2d(out_channel)
self.relu = nn.ReLU()
self.conv2 = nn.Conv2d(in_channels=out_channel,
out_channels=out_channel,
kernel_size=3,
stride=1,
padding=1,
bias=False)
self.bn2 = nn.BatchNorm2d(out_channel)
self.downsample = downsample
def forward(self, x):
identity = x # 捷径输出值
if self.downsample is not None:
identity = self.downsample(x) # 有下采样函数,捷径输出值为下采样输出值
out = self.conv1(x)
out = self.bn1(out)
out = self.relu(out)
out = self.conv2(out)
out = self.bn2(out)
out += identity
out = self.relu(out)
return out
class Bottleneck(nn.Module):
"""
注意:原论文中,在虚线残差结构的主分支上,第一个1x1卷积层的步距是2,第二个3x3卷积层步距是1。
但在pytorch官方实现过程中是第一个1x1卷积层的步距是1,第二个3x3卷积层步距是2,
这么做的好处是能够在top1上提升大概0.5%的准确率。
可参考Resnet v1.5 https://ngc.nvidia.com/catalog/model-scripts/nvidia:resnet_50_v1_5_for_pytorch
"""
expansion = 4
def __init__(self, in_channel, out_channel, stride=1, downsample=None,
groups=1, width_per_group=64):
super(Bottleneck, self).__init__()
width = int(out_channel * (width_per_group / 64.)) * groups
self.conv1 = nn.Conv2d(in_channels=in_channel, out_channels=width,
kernel_size=1, stride=1, bias=False) # squeeze channels
self.bn1 = nn.BatchNorm2d(width)
# -----------------------------------------
self.conv2 = nn.Conv2d(in_channels=width, out_channels=width, groups=groups,
kernel_size=3, stride=stride, bias=False, padding=1)
self.bn2 = nn.BatchNorm2d(width)
# -----------------------------------------
self.conv3 = nn.Conv2d(in_channels=width, out_channels=out_channel*self.expansion,
kernel_size=1, stride=1, bias=False) # unsqueeze channels
self.bn3 = nn.BatchNorm2d(out_channel*self.expansion)
self.relu = nn.ReLU(inplace=True)
self.downsample = downsample
def forward(self, x):
identity = x
if self.downsample is not None:
identity = self.downsample(x)
out = self.conv1(x)
out = self.bn1(out)
out = self.relu(out)
out = self.conv2(out)
out = self.bn2(out)
out = self.relu(out)
out = self.conv3(out)
out = self.bn3(out)
out += identity
out = self.relu(out)
return out
class ResNet(nn.Module):
def __init__(self, block, blocks_num, num_classes=1000, include_top=True):
"""
:param block: BasicBlock(18, 34), Bottleneck(50, 101, 152)
:param blocks_num: 残差网络的个数
:param num_classes: 分类个数
:param include_top: 便于扩展网络
:param groups:
:param width_per_group:
"""
super(ResNet, self).__init__()
self.include_top = include_top
self.in_channel = 64
# self.groups = groups
# self.width_per_group = width_per_group
self.conv1 = nn.Conv2d(3, self.in_channel, kernel_size=7, stride=2,
padding=3, bias=False)
self.bn1 = nn.BatchNorm2d(self.in_channel)
self.relu = nn.ReLU(inplace=True)
self.maxpool = nn.MaxPool2d(kernel_size=3, stride=2, padding=1)
self.layer1 = self._make_layer(block, 64, blocks_num[0])
self.layer2 = self._make_layer(block, 128, blocks_num[1], stride=2)
self.layer3 = self._make_layer(block, 256, blocks_num[2], stride=2)
self.layer4 = self._make_layer(block, 512, blocks_num[3], stride=2)
if self.include_top:
self.avgpool = nn.AdaptiveAvgPool2d((1, 1)) # output size = (1, 1)
self.fc = nn.Linear(512 * block.expansion, num_classes)
for m in self.modules():
if isinstance(m, nn.Conv2d):
nn.init.kaiming_normal_(m.weight, mode='fan_out', nonlinearity='relu')
def _make_layer(self, block, channel, block_num, stride=1):
"""
:param block:
:param channel: 残差结构中卷积核的个数
:param block_num: 残差结构的个数
:param stride:
:return:
"""
downsample = None
if stride != 1 or self.in_channel != channel * block.expansion:
downsample = nn.Sequential(
nn.Conv2d(self.in_channel, channel * block.expansion, kernel_size=1, stride=stride, bias=False),
nn.BatchNorm2d(channel * block.expansion))
layers = []
layers.append(block(self.in_channel,
channel,
downsample=downsample,
stride=stride))
self.in_channel = channel * block.expansion
for _ in range(1, block_num):
layers.append(block(self.in_channel,
channel))
return nn.Sequential(*layers)
def forward(self, x):
x = self.conv1(x)
x = self.bn1(x)
x = self.relu(x)
x = self.maxpool(x)
x = self.layer1(x)
x = self.layer2(x)
x = self.layer3(x)
x = self.layer4(x)
if self.include_top:
x = self.avgpool(x)
x = torch.flatten(x, 1)
x = self.fc(x)
return x
def resnet34(num_classes=1000, include_top=True):
# https://download.pytorch.org/models/resnet34-333f7ec4.pth
return ResNet(BasicBlock, [3, 4, 6, 3], num_classes=num_classes, include_top=include_top)
def resnet50(num_classes=1000, include_top=True):
# https://download.pytorch.org/models/resnet50-19c8e357.pth
return ResNet(Bottleneck, [3, 4, 6, 3], num_classes=num_classes, include_top=include_top)
def resnet101(num_classes=1000, include_top=True):
# https://download.pytorch.org/models/resnet101-5d3b4d8f.pth
return ResNet(Bottleneck, [3, 4, 23, 3], num_classes=num_classes, include_top=include_top)
def resnext50_32x4d(num_classes=1000, include_top=True):
# https://download.pytorch.org/models/resnext50_32x4d-7cdf4587.pth
groups = 32
width_per_group = 4
return ResNet(Bottleneck, [3, 4, 6, 3],
num_classes=num_classes,
include_top=include_top,
groups=groups,
width_per_group=width_per_group)
def resnext101_32x8d(num_classes=1000, include_top=True

程序员张小妍
- 粉丝: 1w+
- 资源: 3474
最新资源
- 8bit逐次逼近型SAR ADC电路设计成品 入门时期的第三款sarADC,适合新手学习等 包括电路文件和详细设计文档 smic0.18工艺,单端结构,3.3V供电 整体采样率500k,可实现基
- 操作系统实验 ucorelab4内核线程管理
- 脉冲注入法,持续注入,启动低速运行过程中注入,电感法,ipd,力矩保持,无霍尔无感方案,媲美有霍尔效果 bldc控制器方案,无刷电机 提供源码,原理图
- Matlab Simulink#直驱永磁风电机组并网仿真模型 基于永磁直驱式风机并网仿真模型 采用背靠背双PWM变流器,先整流,再逆变 不仅实现电机侧的有功、无功功率的解耦控制和转速调节,而且能实
- 157389节奏盒子地狱模式第三阶段7.apk
- 操作系统实验ucore lab3
- DG储能选址定容模型matlab 程序采用改进粒子群算法,考虑时序性得到分布式和储能的选址定容模型,程序运行可靠 这段程序是一个改进的粒子群算法,主要用于解决电力系统中的优化问题 下面我将对程序进行详
- final_work_job1(1).sql
- 区块链与联邦学习结合:FedChain项目详细复现指南
- 西门子S7 和 S7 Plus 协议开发示例
资源上传下载、课程学习等过程中有任何疑问或建议,欢迎提出宝贵意见哦~我们会及时处理!
点击此处反馈


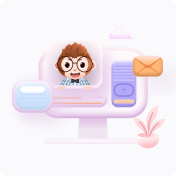