# TF Keras YOLOv4/v3/v2 Modelset
[](LICENSE)
## Introduction
A general YOLOv4/v3/v2 object detection pipeline inherited from [keras-yolo3-Mobilenet](https://github.com/Adamdad/keras-YOLOv3-mobilenet)/[keras-yolo3](https://github.com/qqwweee/keras-yolo3) and [YAD2K](https://github.com/allanzelener/YAD2K). Implement with tf.keras, including data collection/annotation, model training/tuning, model evaluation and on device deployment. Support different architecture and different technologies:
#### Backbone
- [x] CSPDarknet53
- [x] Darknet53/Tiny Darknet
- [x] Darknet19
- [x] MobilenetV1
- [x] MobilenetV2
- [x] MobilenetV3(Large/Small)
- [x] PeleeNet ([paper](https://arxiv.org/abs/1804.06882))
- [x] GhostNet ([paper](https://arxiv.org/abs/1911.11907))
- [x] EfficientNet
- [x] Xception
- [x] VGG16
#### Head
- [x] YOLOv4 (Lite)
- [x] Tiny YOLOv4 (Lite, no-SPP, unofficial)
- [x] YOLOv3 (Lite, SPP)
- [x] YOLOv3 Nano ([paper](https://arxiv.org/abs/1910.01271)) (unofficial)
- [x] Tiny YOLOv3 (Lite)
- [x] YOLOv2 (Lite)
- [x] Tiny YOLOv2 (Lite)
#### Loss
- [x] YOLOv3 loss
- [x] YOLOv2 loss
- [x] Binary focal classification loss
- [x] Softmax focal classification loss
- [x] GIoU localization loss
- [x] DIoU localization loss ([paper](https://arxiv.org/abs/1911.08287))
- [x] Binary focal loss for objectness (experimental)
- [x] Label smoothing for classification loss
#### Postprocess
- [x] Numpy YOLOv3/v2 postprocess implementation
- [x] TFLite/MNN C++ YOLOv3/v2 postprocess implementation
- [x] tf.keras batch-wise YOLOv3/v2 postprocess layer
- [x] DIoU-NMS bounding box postprocess (numpy/C++)
- [x] SoftNMS bounding box postprocess (numpy)
- [x] Eliminate grid sensitivity (numpy/C++, from [YOLOv4](https://arxiv.org/abs/2004.10934))
- [x] WBF(Weighted-Boxes-Fusion) bounding box postprocess (numpy) ([paper](https://arxiv.org/abs/1910.13302))
- [x] Cluster NMS family (Fast/Matrix/SPM/Weighted) bounding box postprocess (numpy) ([paper](https://arxiv.org/abs/2005.03572))
#### Train tech
- [x] Transfer training from imagenet
- [x] Singlescale image input training
- [x] Multiscale image input training
- [x] Dynamic learning rate decay (Cosine/Exponential/Polynomial/PiecewiseConstant)
- [x] Weights Average policy for optimizer (EMA/SWA/Lookahead, valid for TF-2.x with tfa)
- [x] Mosaic data augmentation (from [YOLOv4](https://arxiv.org/abs/2004.10934))
- [x] GridMask data augmentation ([paper](https://arxiv.org/abs/2001.04086))
- [x] Multi anchors for single GT (from [YOLOv4](https://arxiv.org/abs/2004.10934))
- [x] Pruned model training (only valid for TF 1.x)
- [x] Multi-GPU training with SyncBatchNorm support (valid for TF-2.2 and later)
#### On-device deployment
- [x] Tensorflow-Lite Float32/UInt8 model inference
- [x] MNN Float32/UInt8 model inference
## Quick Start
1. Install requirements on Ubuntu 16.04/18.04:
```
# apt install python3-opencv
# pip install Cython
# pip install -r requirements.txt
```
2. Download Related Darknet/YOLOv2/v3/v4 weights from [YOLO website](http://pjreddie.com/darknet/yolo/) and [AlexeyAB/darknet](https://github.com/AlexeyAB/darknet).
3. Convert the Darknet YOLO model to a Keras model.
4. Run YOLO detection on your image or video, default using Tiny YOLOv3 model.
```
# wget -O weights/darknet53.conv.74.weights https://pjreddie.com/media/files/darknet53.conv.74
# wget -O weights/darknet19_448.conv.23.weights https://pjreddie.com/media/files/darknet19_448.conv.23
# wget -O weights/yolov3.weights https://pjreddie.com/media/files/yolov3.weights
# wget -O weights/yolov3-tiny.weights https://pjreddie.com/media/files/yolov3-tiny.weights
# wget -O weights/yolov3-spp.weights https://pjreddie.com/media/files/yolov3-spp.weights
# wget -O weights/yolov2.weights http://pjreddie.com/media/files/yolo.weights
# wget -O weights/yolov2-voc.weights http://pjreddie.com/media/files/yolo-voc.weights
# wget -O weights/yolov2-tiny.weights https://pjreddie.com/media/files/yolov2-tiny.weights
# wget -O weights/yolov2-tiny-voc.weights https://pjreddie.com/media/files/yolov2-tiny-voc.weights
### manually download csdarknet53-omega_final.weights from https://drive.google.com/open?id=18jCwaL4SJ-jOvXrZNGHJ5yz44g9zi8Hm
# wget -O weights/yolov4.weights https://github.com/AlexeyAB/darknet/releases/download/darknet_yolo_v3_optimal/yolov4.weights
# python tools/model_converter/convert.py cfg/yolov3.cfg weights/yolov3.weights weights/yolov3.h5
# python tools/model_converter/convert.py cfg/yolov3-tiny.cfg weights/yolov3-tiny.weights weights/yolov3-tiny.h5
# python tools/model_converter/convert.py cfg/yolov3-spp.cfg weights/yolov3-spp.weights weights/yolov3-spp.h5
# python tools/model_converter/convert.py cfg/yolov2.cfg weights/yolov2.weights weights/yolov2.h5
# python tools/model_converter/convert.py cfg/yolov2-voc.cfg weights/yolov2-voc.weights weights/yolov2-voc.h5
# python tools/model_converter/convert.py cfg/yolov2-tiny.cfg weights/yolov2-tiny.weights weights/yolov2-tiny.h5
# python tools/model_converter/convert.py cfg/yolov2-tiny-voc.cfg weights/yolov2-tiny-voc.weights weights/yolov2-tiny-voc.h5
# python tools/model_converter/convert.py cfg/darknet53.cfg weights/darknet53.conv.74.weights weights/darknet53.h5
# python tools/model_converter/convert.py cfg/darknet19_448_body.cfg weights/darknet19_448.conv.23.weights weights/darknet19.h5
# python tools/model_converter/convert.py cfg/csdarknet53-omega.cfg weights/csdarknet53-omega_final.weights weights/cspdarknet53.h5
### make sure to reorder output tensors for YOLOv4 cfg and weights file
# python tools/model_converter/convert.py --yolo4_reorder cfg/yolov4.cfg weights/yolov4.weights weights/yolov4.h5
### Scaled YOLOv4
### manually download yolov4-csp.weights from https://drive.google.com/file/d/1NQwz47cW0NUgy7L3_xOKaNEfLoQuq3EL/view?usp=sharing
# python tools/model_converter/convert.py --yolo4_reorder cfg/yolov4-csp_fixed.cfg weights/yolov4-csp.weights weights/scaled-yolov4-csp.h5
### Yolo-Fastest
# wget -O weights/yolo-fastest.weights https://github.com/dog-qiuqiu/Yolo-Fastest/raw/master/ModelZoo/yolo-fastest-1.0_coco/yolo-fastest.weights
# wget -O weights/yolo-fastest-xl.weights https://github.com/dog-qiuqiu/Yolo-Fastest/raw/master/ModelZoo/yolo-fastest-1.0_coco/yolo-fastest-xl.weights
# python tools/model_converter/convert.py cfg/yolo-fastest.cfg weights/yolo-fastest.weights weights/yolo-fastest.h5
# python tools/model_converter/convert.py cfg/yolo-fastest-xl.cfg weights/yolo-fastest-xl.weights weights/yolo-fastest-xl.h5
# python yolo.py --image
# python yolo.py --input=<your video file>
```
For other model, just do in a similar way, but specify different model type, weights path and anchor path with `--model_type`, `--weights_path` and `--anchors_path`.
Image detection sample:
<p align="center">
<img src="assets/dog_inference.jpg">
<img src="assets/kite_inference.jpg">
</p>
## Guide of train/evaluate/demo
### Train
1. Generate train/val/test annotation file and class names file.
Data annotation file format:
* One row for one image in annotation file;
* Row format: `image_file_path box1 box2 ... boxN`;
* Box format: `x_min,y_min,x_max,y_max,class_id` (no space).
* Here is an example:
```
path/to/img1.jpg 50,100,150,200,0 30,50,200,120,3
path/to/img2.jpg 120,300,250,600,2
...
```
1. For VOC style dataset, you can use [voc_annotation.py](https://github.com/david8862/keras-YOLOv3-model-set/blob/master/tools/dataset_converter/voc_annotation.py) to convert original dataset to our annotation file:
```
# cd tools/dataset_converter/ && python voc_annotation.py -h
usage: voc_annotation.py [-h] [--dataset_path DATASET_PATH] [--year YEAR]
[--set SET] [--output_path OUTPUT_PATH]
[--classes_path CLASSES_PATH] [--include_difficult]
[--include_no_obj]
convert PascalVOC
没有合适的资源?快使用搜索试试~ 我知道了~
这是一个基于yolo-fastest模型的小车,主控是art-pi开发板,使用了rt thread操作系统 该小车能够识别特定种
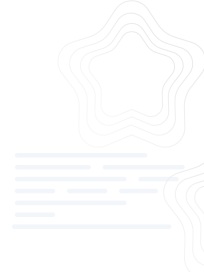
共2000个文件
h:815个
c:733个
html:188个

需积分: 5 0 下载量 21 浏览量
2024-06-04
09:43:44
上传
评论
收藏 669.7MB ZIP 举报
温馨提示
这是一个基于yolo-fastest模型的小车,主控是art-pi开发板,使用了rt thread操作系统。该小车能够识别特定种类的垃圾,并使用机械臂将其拾取并放置在垃圾筐内 演示效果 Demo 1 demo1存在一些问题: 如小车有时对不准,距离目标过远时容易走偏,车两侧的铝板使小车在旋转时不够稳定等等。 Demo 2 demo2对demo1中的问题进行一系列的改进: 对于小车对不准的问题,我们采用了在距离目标一定距离时进行第二次PID调整,这样使得小车积累一定误差后能将误差清零。在视频中, 可以看到小车在一定距离时有一明显停顿,这就是在进行第二次PID调整。 对于车身不稳,我们改进了机械结构,采用一个篮子并改变了摄像头和机械臂的位置,以及机械臂的初始状态,使得小车质量分布更集中。
资源推荐
资源详情
资源评论
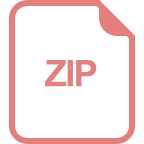
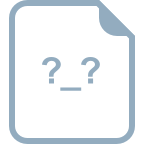
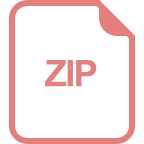
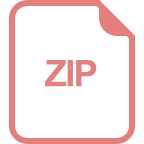
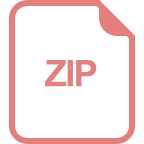
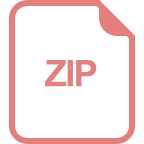
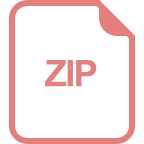
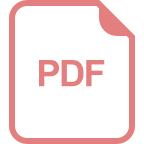
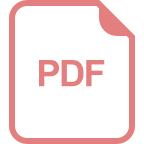
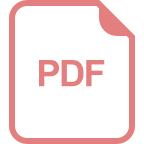
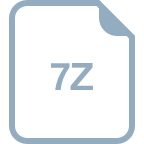
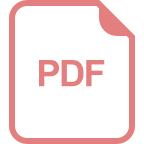
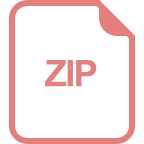
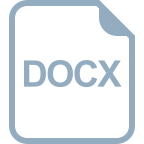
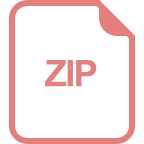
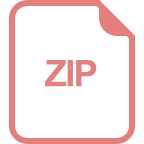
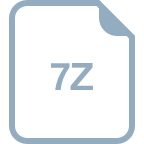
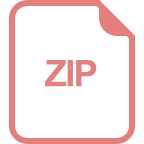
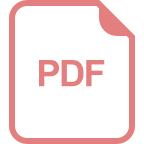
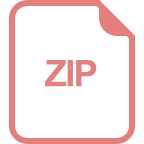
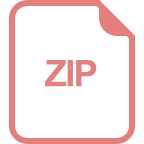
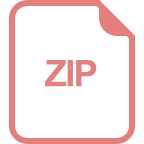
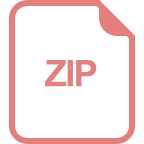
收起资源包目录

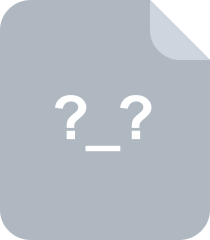
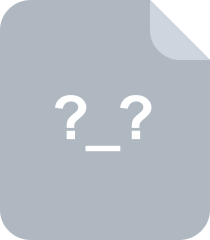
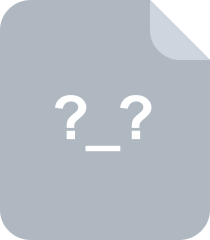
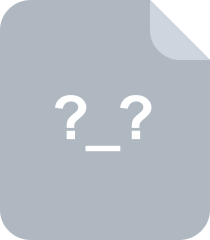
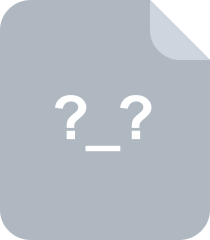
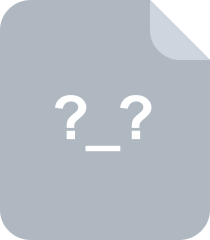
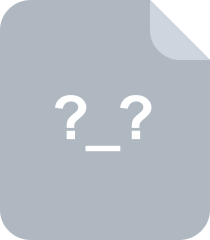
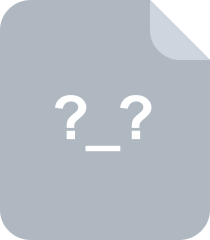
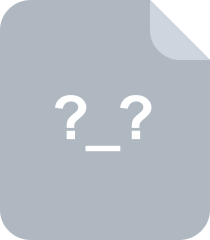
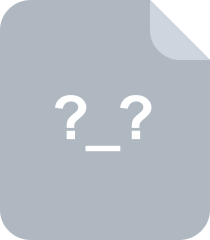
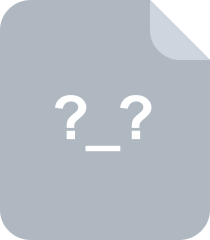
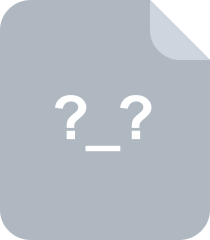
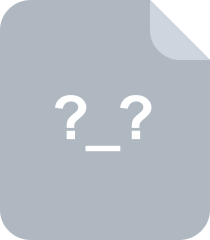
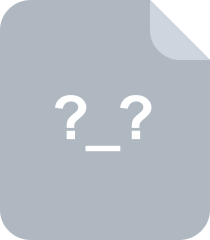
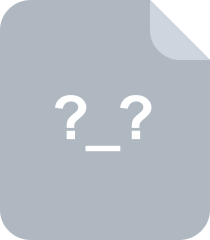
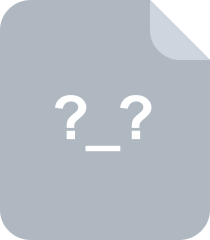
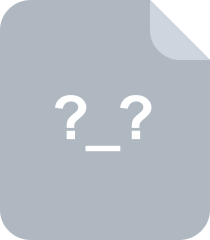
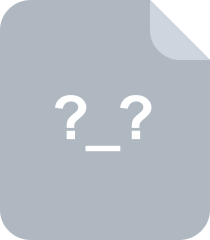
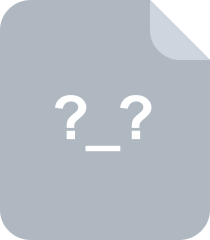
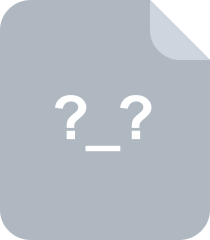
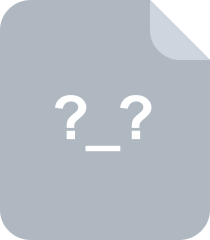
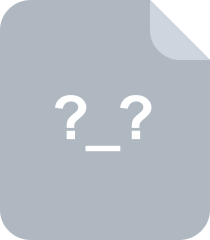
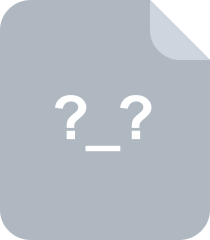
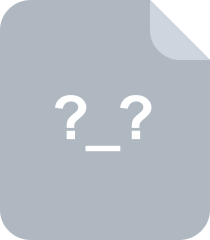
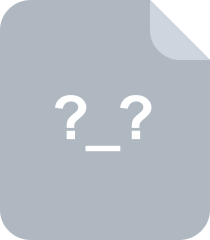
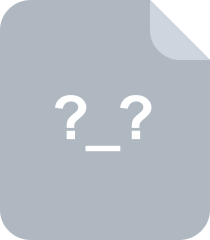
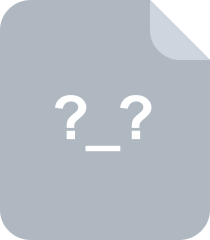
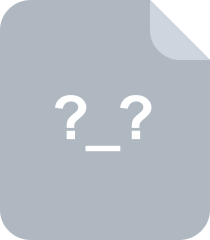
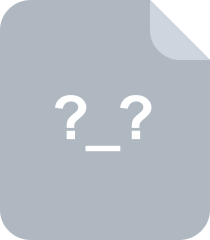
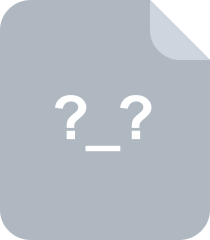
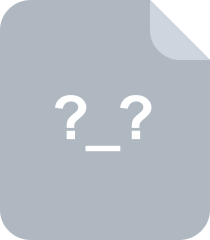
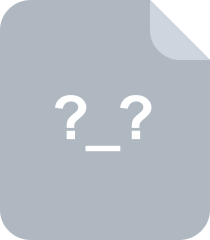
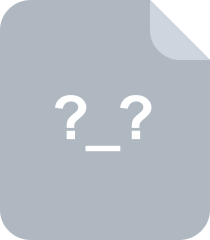
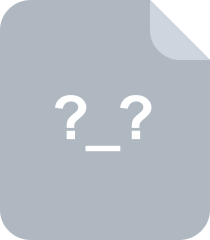
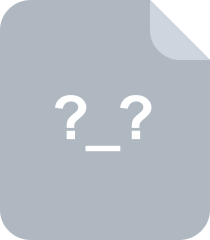
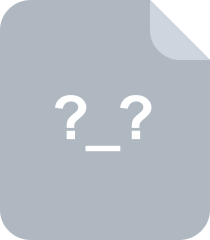
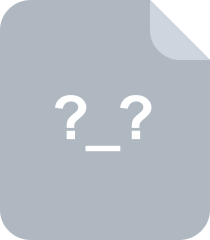
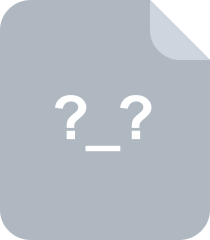
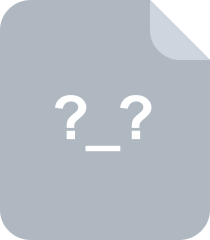
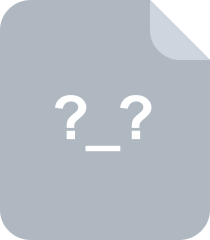
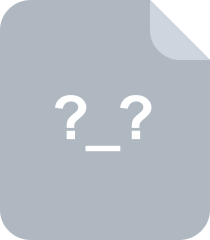
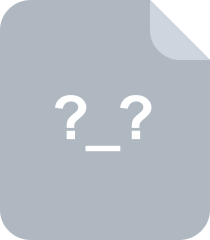
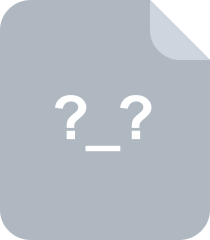
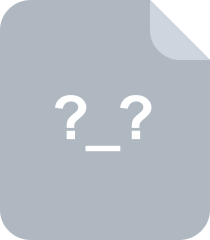
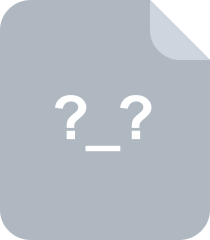
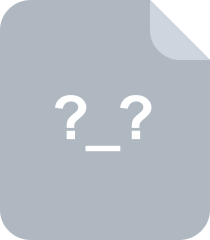
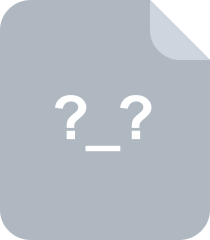
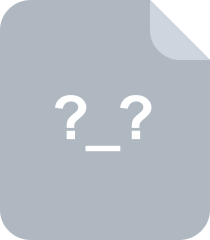
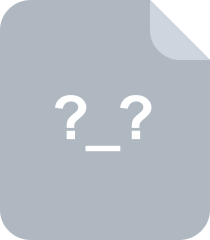
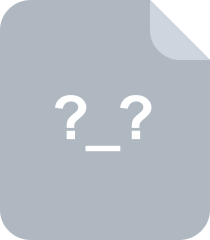
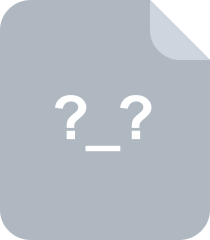
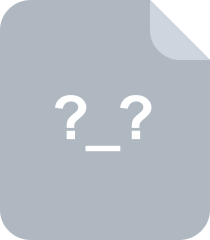
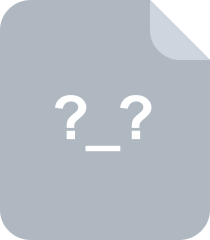
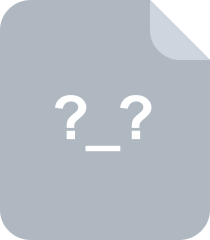
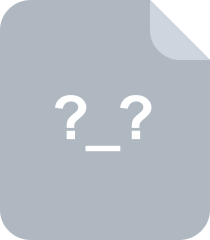
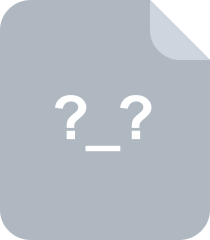
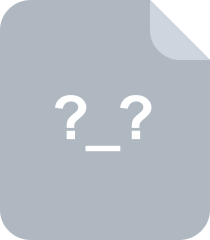
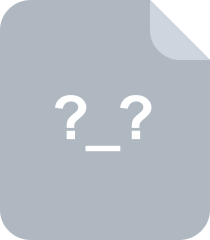
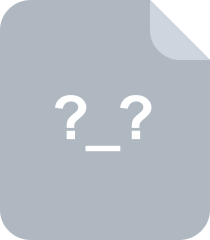
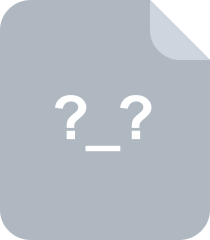
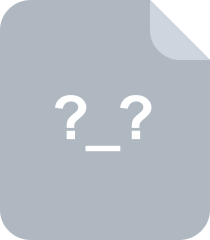
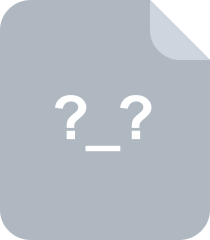
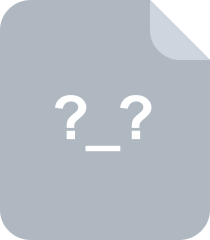
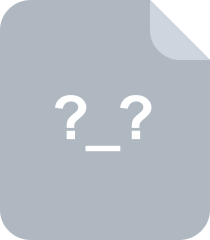
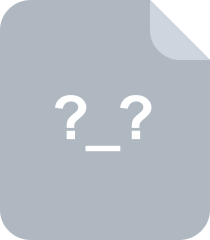
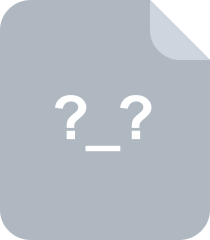
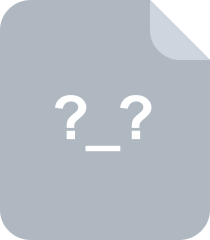
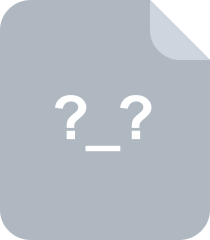
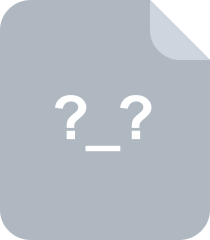
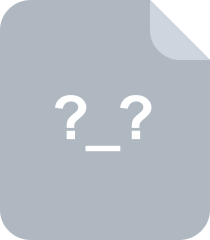
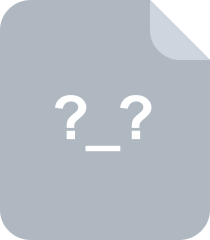
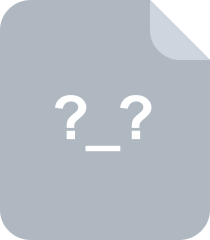
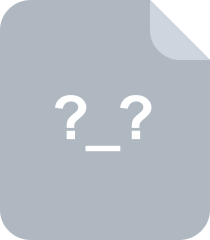
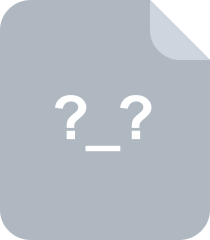
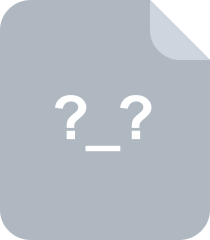
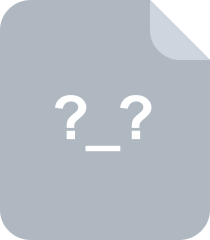
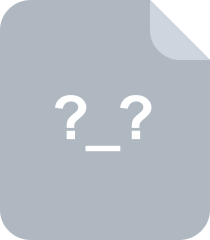
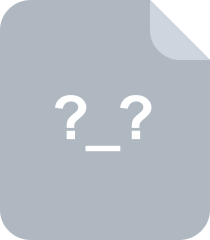
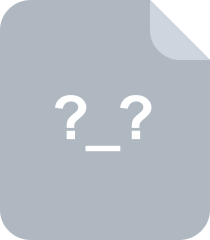
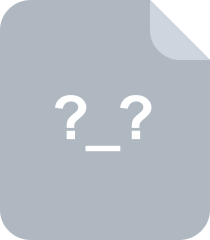
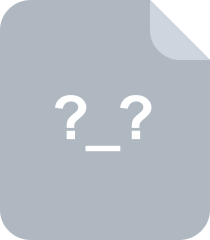
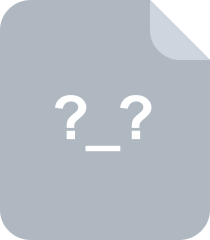
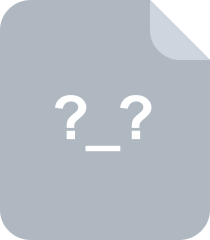
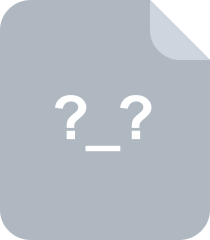
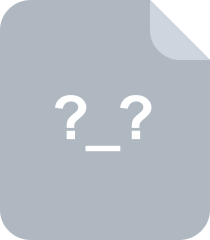
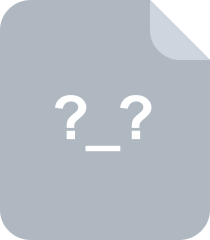
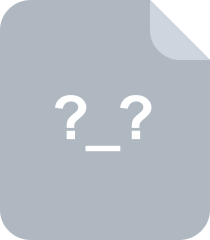
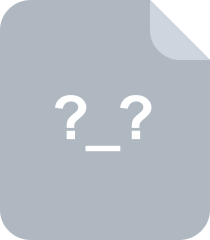
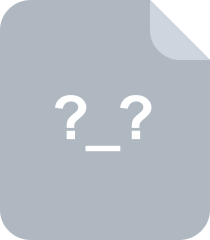
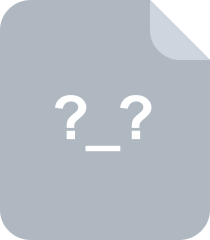
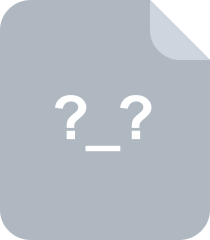
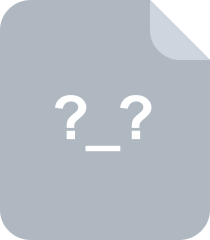
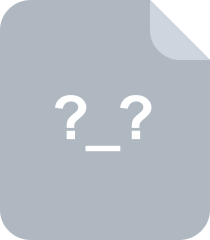
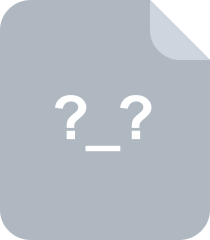
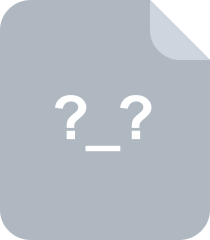
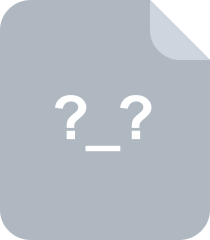
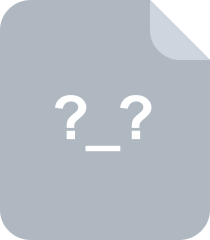
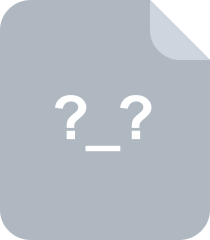
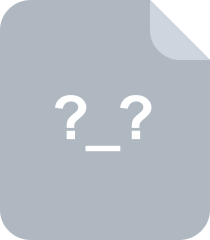
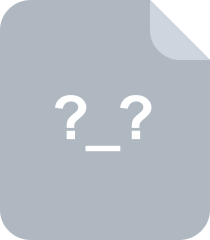
共 2000 条
- 1
- 2
- 3
- 4
- 5
- 6
- 20
资源评论
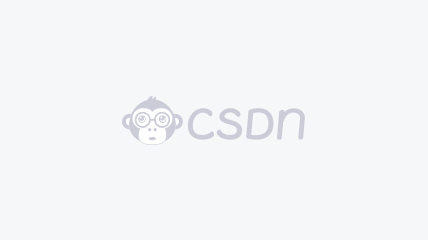

程序媛小y
- 粉丝: 5625
- 资源: 213
上传资源 快速赚钱
我的内容管理 展开
我的资源 快来上传第一个资源
我的收益
登录查看自己的收益我的积分 登录查看自己的积分
我的C币 登录后查看C币余额
我的收藏
我的下载
下载帮助

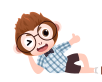
最新资源
- 中期检查+结项报告参考模板+教改类课题+开题报告【重磅,更新!】
- DGA(流量入侵)网络安全数据集
- 【毕业设计/课程设计】免费springbootvue阿博图书馆管理系统源码
- <项目代码>YOLOv8 手机识别<目标检测>
- 【毕业设计/课程设计】免费springboot+vue教师工作量管理系统源码
- 开发 Deeplab V3 卷积神经网络,以划分 NAIP 中的地面太阳能电池阵.ipynb
- python《通过图卷积网络进行城市交通流预测的研究》+项目源码+文档说明+说明
- 【重磅,更新!】基于2008-2022年熵值法计算的环境污染指数
- 【毕业设计/课程设计】免费springboot+vue甘肃非物质文化网站的源码
- 使用免费卫星图像划分北卡罗来纳州所有地面安装太阳能电池阵的方法.ipynb
资源上传下载、课程学习等过程中有任何疑问或建议,欢迎提出宝贵意见哦~我们会及时处理!
点击此处反馈


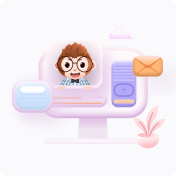
安全验证
文档复制为VIP权益,开通VIP直接复制
