---
license: apache-2.0
tags:
- vision
- image-classification
datasets:
- imagenet-1k
---
# ResNet-50 v1.5
ResNet model pre-trained on ImageNet-1k at resolution 224x224. It was introduced in the paper [Deep Residual Learning for Image Recognition](https://arxiv.org/abs/1512.03385) by He et al.
Disclaimer: The team releasing ResNet did not write a model card for this model so this model card has been written by the Hugging Face team.
## Model description
ResNet (Residual Network) is a convolutional neural network that democratized the concepts of residual learning and skip connections. This enables to train much deeper models.
This is ResNet v1.5, which differs from the original model: in the bottleneck blocks which require downsampling, v1 has stride = 2 in the first 1x1 convolution, whereas v1.5 has stride = 2 in the 3x3 convolution. This difference makes ResNet50 v1.5 slightly more accurate (\~0.5% top1) than v1, but comes with a small performance drawback (~5% imgs/sec) according to [Nvidia](https://catalog.ngc.nvidia.com/orgs/nvidia/resources/resnet_50_v1_5_for_pytorch).
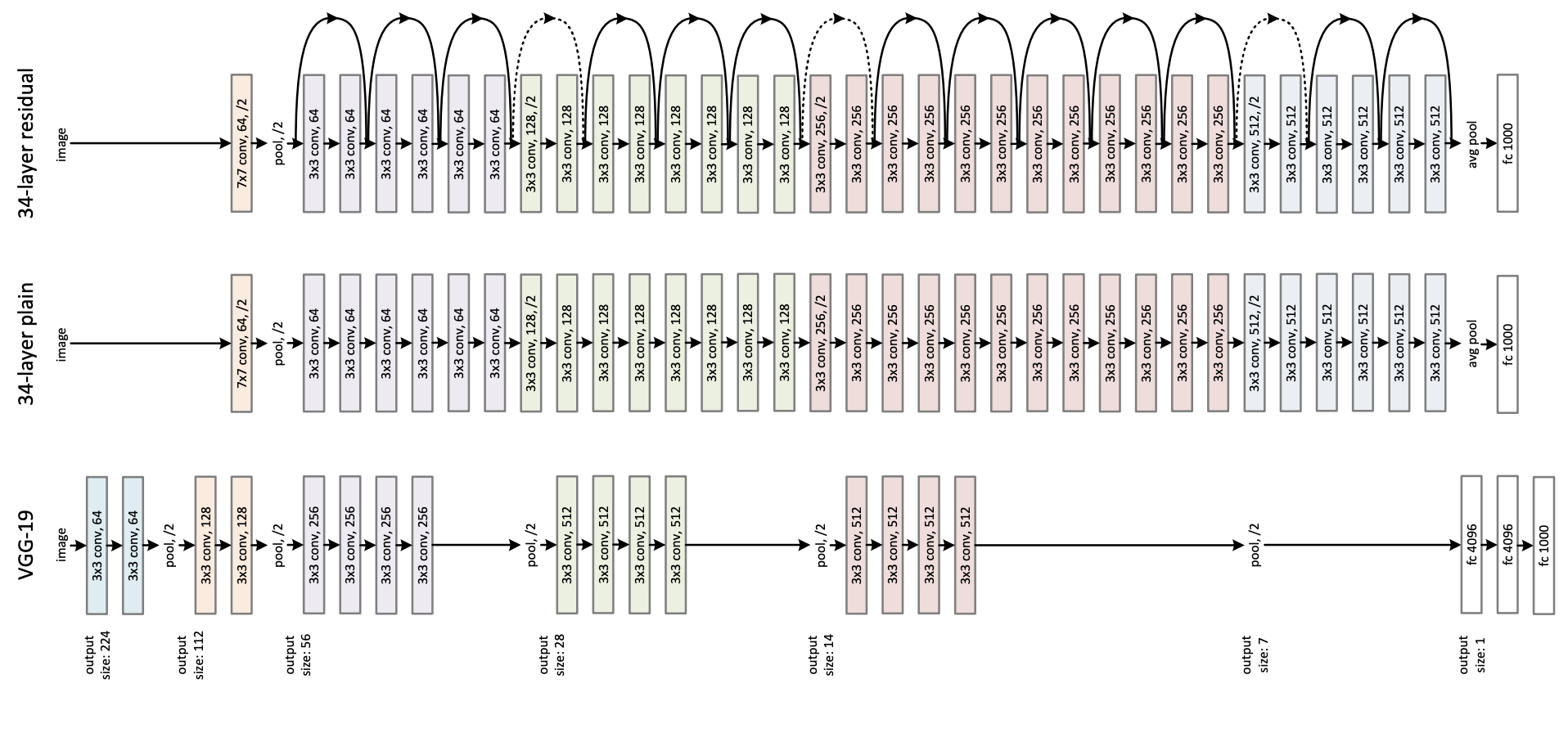
## Intended uses & limitations
You can use the raw model for image classification. See the [model hub](https://huggingface.co/models?search=resnet) to look for
fine-tuned versions on a task that interests you.
### How to use
Here is how to use this model to classify an image of the COCO 2017 dataset into one of the 1,000 ImageNet classes:
```python
from transformers import AutoImageProcessor, ResNetForImageClassification
import torch
from datasets import load_dataset
dataset = load_dataset("huggingface/cats-image")
image = dataset["test"]["image"][0]
processor = AutoImageProcessor.from_pretrained("microsoft/resnet-50")
model = ResNetForImageClassification.from_pretrained("microsoft/resnet-50")
inputs = processor(image, return_tensors="pt")
with torch.no_grad():
logits = model(**inputs).logits
# model predicts one of the 1000 ImageNet classes
predicted_label = logits.argmax(-1).item()
print(model.config.id2label[predicted_label])
```
For more code examples, we refer to the [documentation](https://huggingface.co/docs/transformers/main/en/model_doc/resnet).
### BibTeX entry and citation info
```bibtex
@inproceedings{he2016deep,
title={Deep residual learning for image recognition},
author={He, Kaiming and Zhang, Xiangyu and Ren, Shaoqing and Sun, Jian},
booktitle={Proceedings of the IEEE conference on computer vision and pattern recognition},
pages={770--778},
year={2016}
}
```


0仰望星空007
- 粉丝: 4587
- 资源: 603
最新资源
- (174927846)图书管理系统课设.doc
- 基于PLC音乐喷泉控制系统设计音乐喷泉组态设计音乐喷泉
- (143928440)SQL-数据库图书管理系统.docx
- TensorFlow 实战案例:利用 LSTM 进行电量预测(包含代码和数据)
- (176289228)H60-html手机网站-网页源码移动端前端-H5模板-自适应响应式源码.zip
- (176301404)响应式-HTML手机电脑网站-网页源码移动端前端-H5模板-自适应响应式源码.zip
- 基于MATLAB人体异常姿态行为检测识别源码+GUI界面(高分项目)
- 扫地机器人路径规划问题,算法是全覆盖内螺旋算法,使用MATLAB实现,下列为运行图过程截图 这段代码是一个扫地机器人的仿真程序 程序的主要功能是模拟机器人在一个房间内清扫的过程 下面我将对程序进行详细
- 数据分析-01-ZFB交易行为数据分析(包含代码和数据)
- matlab的人体异常行为检测识别系统(源码,文档,GUI).zip
- (175586048)数据可视化大屏通用模板
- unityppt插件打开PPT
- (17209246)迷宫 课程设计
- (8788644)迷宫问题课程设计
- S7-200 PLC和组态王组态温度PID控制加热炉电阻炉 组态王动画仿真,带PLC源代码,plc程序每一条都带着解释,组态王源代码,图纸,IO地址分配
- python圣诞树生成-python
资源上传下载、课程学习等过程中有任何疑问或建议,欢迎提出宝贵意见哦~我们会及时处理!
点击此处反馈


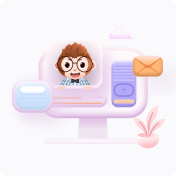