没有合适的资源?快使用搜索试试~ 我知道了~
资源推荐
资源详情
资源评论
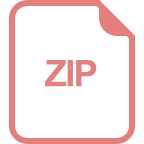
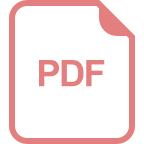
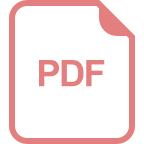
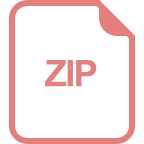
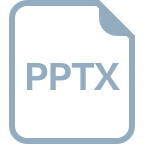
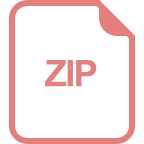
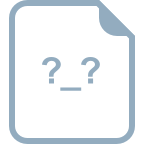
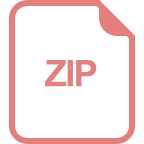
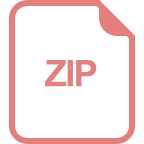
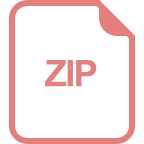
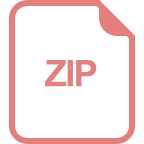
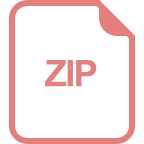
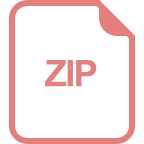
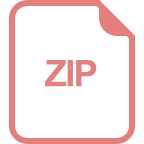
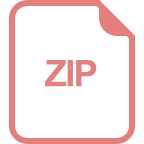
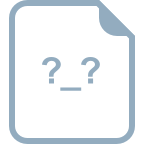
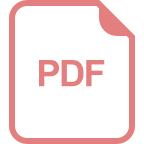
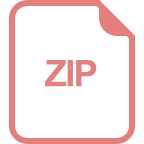
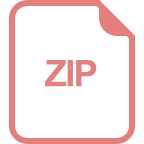
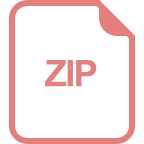
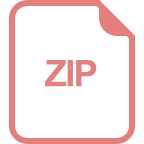
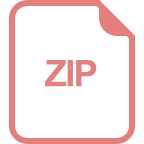
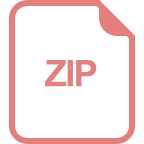
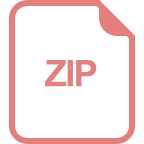
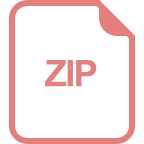
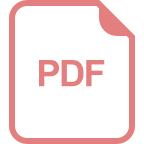
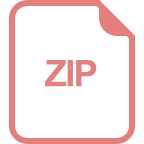
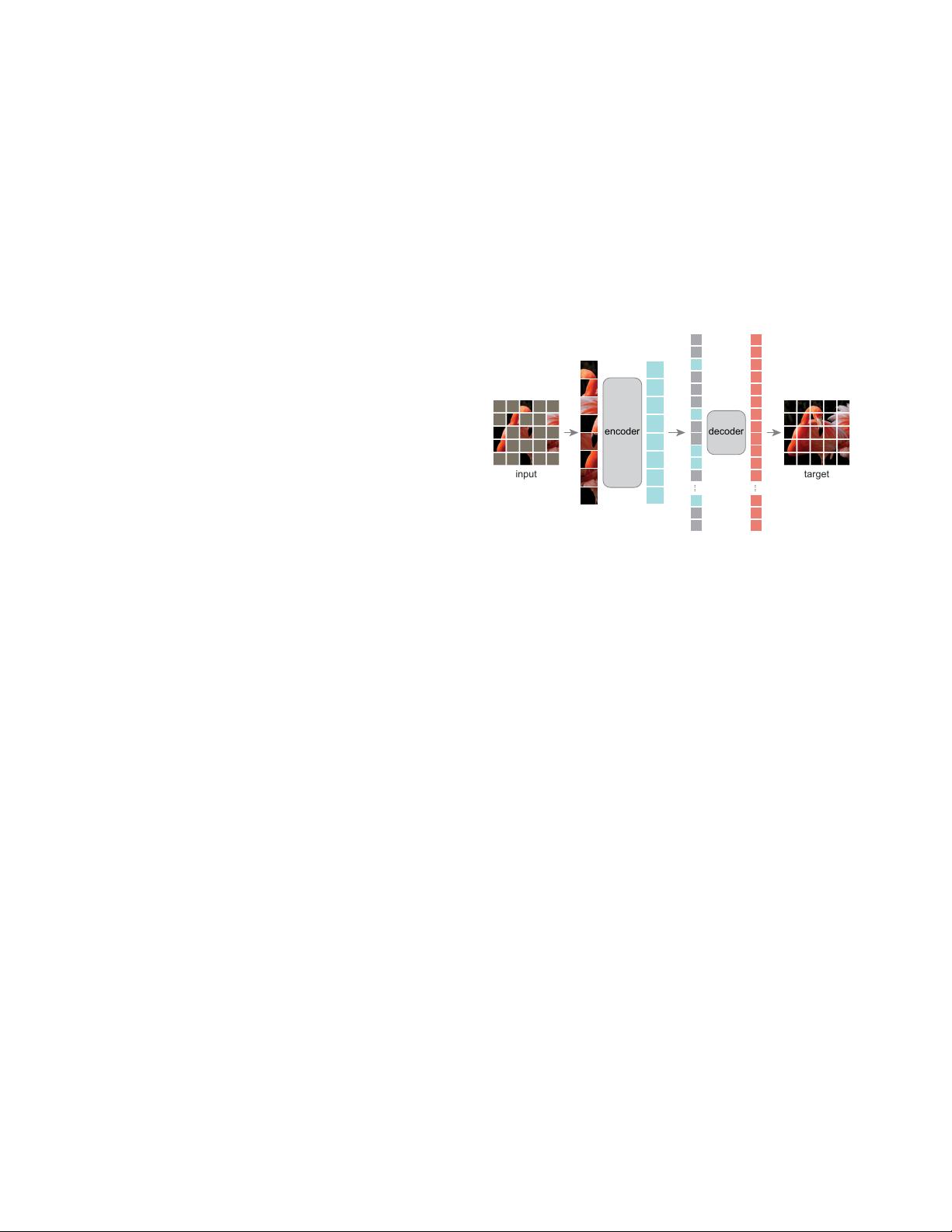
Masked Autoencoders Are Scalable Vision Learners
Kaiming He
∗,†
Xinlei Chen
∗
Saining Xie Yanghao Li Piotr Doll
´
ar Ross Girshick
∗
equal technical contribution
†
project lead
Facebook AI Research (FAIR)
Abstract
This paper shows that masked autoencoders (MAE) are
scalable self-supervised learners for computer vision. Our
MAE approach is simple: we mask random patches of the
input image and reconstruct the missing pixels. It is based
on two core designs. First, we develop an asymmetric
encoder-decoder architecture, with an encoder that oper-
ates only on the visible subset of patches (without mask to-
kens), along with a lightweight decoder that reconstructs
the original image from the latent representation and mask
tokens. Second, we find that masking a high proportion
of the input image, e.g., 75%, yields a nontrivial and
meaningful self-supervisory task. Coupling these two de-
signs enables us to train large models efficiently and ef-
fectively: we accelerate training (by 3× or more) and im-
prove accuracy. Our scalable approach allows for learning
high-capacity models that generalize well: e.g., a vanilla
ViT-Huge model achieves the best accuracy (87.8%) among
methods that use only ImageNet-1K data. Transfer per-
formance in downstream tasks outperforms supervised pre-
training and shows promising scaling behavior.
1. Introduction
Deep learning has witnessed an explosion of archi-
tectures of continuously growing capability and capacity
[33, 25, 57]. Aided by the rapid gains in hardware, mod-
els today can easily overfit one million images [13] and
begin to demand hundreds of millions of—often publicly
inaccessible—labeled images [16].
This appetite for data has been successfully addressed in
natural language processing (NLP) by self-supervised pre-
training. The solutions, based on autoregressive language
modeling in GPT [47, 48, 4] and masked autoencoding in
BERT [14], are conceptually simple: they remove a portion
of the data and learn to predict the removed content. These
methods now enable training of generalizable NLP models
containing over one hundred billion parameters [4].
The idea of masked autoencoders, a form of more gen-
eral denoising autoencoders [58], is natural and applicable
in computer vision as well. Indeed, closely related research
encoder
....
....
decoder
input target
Figure 1. Our MAE architecture. During pre-training, a large
random subset of image patches (e.g., 75%) is masked out. The
encoder is applied to the small subset of visible patches. Mask
tokens are introduced after the encoder, and the full set of en-
coded patches and mask tokens is processed by a small decoder
that reconstructs the original image in pixels. After pre-training,
the decoder is discarded and the encoder is applied to uncorrupted
images (full sets of patches) for recognition tasks.
in vision [59, 46] preceded BERT. However, despite signif-
icant interest in this idea following the success of BERT,
progress of autoencoding methods in vision lags behind
NLP. We ask: what makes masked autoencoding different
between vision and language? We attempt to answer this
question from the following perspectives:
(i) Until recently, architectures were different. In vision,
convolutional networks [34] were dominant over the last
decade [33]. Convolutions typically operate on regular grids
and it is not straightforward to integrate ‘indicators’ such as
mask tokens [14] or positional embeddings [57] into con-
volutional networks. This architectural gap, however, has
been addressed with the introduction of Vision Transform-
ers (ViT) [16] and should no longer present an obstacle.
(ii) Information density is different between language
and vision. Languages are human-generated signals that
are highly semantic and information-dense. When training
a model to predict only a few missing words per sentence,
this task appears to induce sophisticated language under-
standing. Images, on the contrary, are natural signals with
heavy spatial redundancy—e.g., a missing patch can be re-
covered from neighboring patches with little high-level un-
15979
2022 IEEE/CVF Conference on Computer Vision and Pattern Recognition (CVPR)
978-1-6654-6946-3/22/$31.00 ©2022 IEEE
DOI 10.1109/CVPR52688.2022.01553
2022 IEEE/CVF Conference on Computer Vision and Pattern Recognition (CVPR) | 978-1-6654-6946-3/22/$31.00 ©2022 IEEE | DOI: 10.1109/CVPR52688.2022.01553
Authorized licensed use limited to: Shandong Normal University. Downloaded on April 15,2023 at 07:13:39 UTC from IEEE Xplore. Restrictions apply.
资源评论
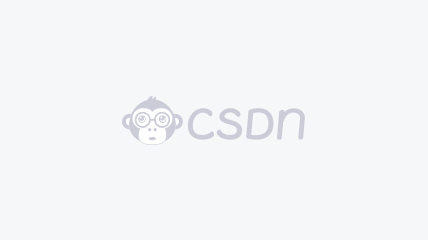

薛定谔的猫ovo
- 粉丝: 5w+
- 资源: 4
上传资源 快速赚钱
我的内容管理 展开
我的资源 快来上传第一个资源
我的收益
登录查看自己的收益我的积分 登录查看自己的积分
我的C币 登录后查看C币余额
我的收藏
我的下载
下载帮助

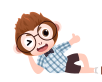
最新资源
资源上传下载、课程学习等过程中有任何疑问或建议,欢迎提出宝贵意见哦~我们会及时处理!
点击此处反馈


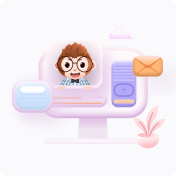
安全验证
文档复制为VIP权益,开通VIP直接复制
