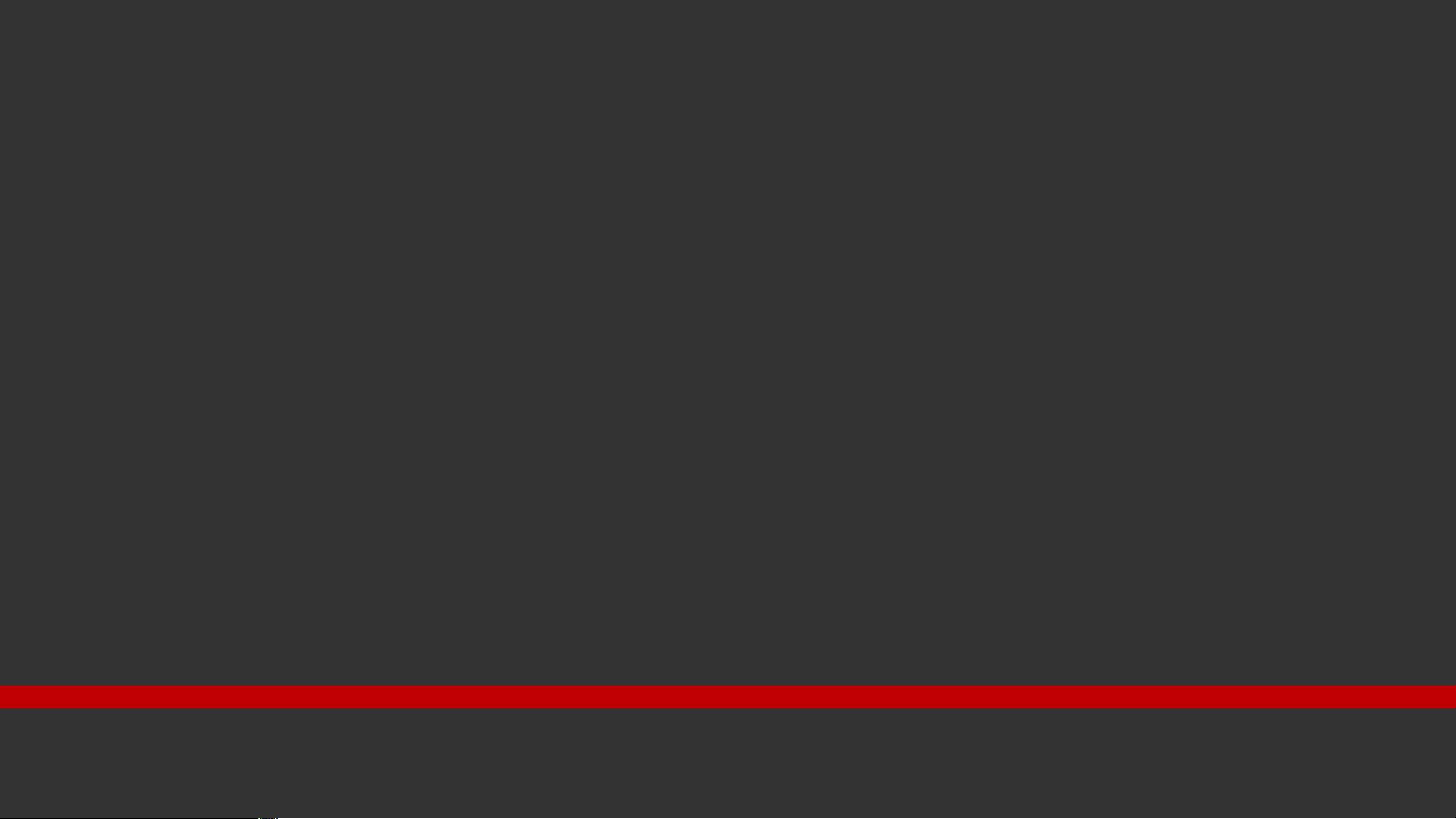
Challenge 6:
Quantification
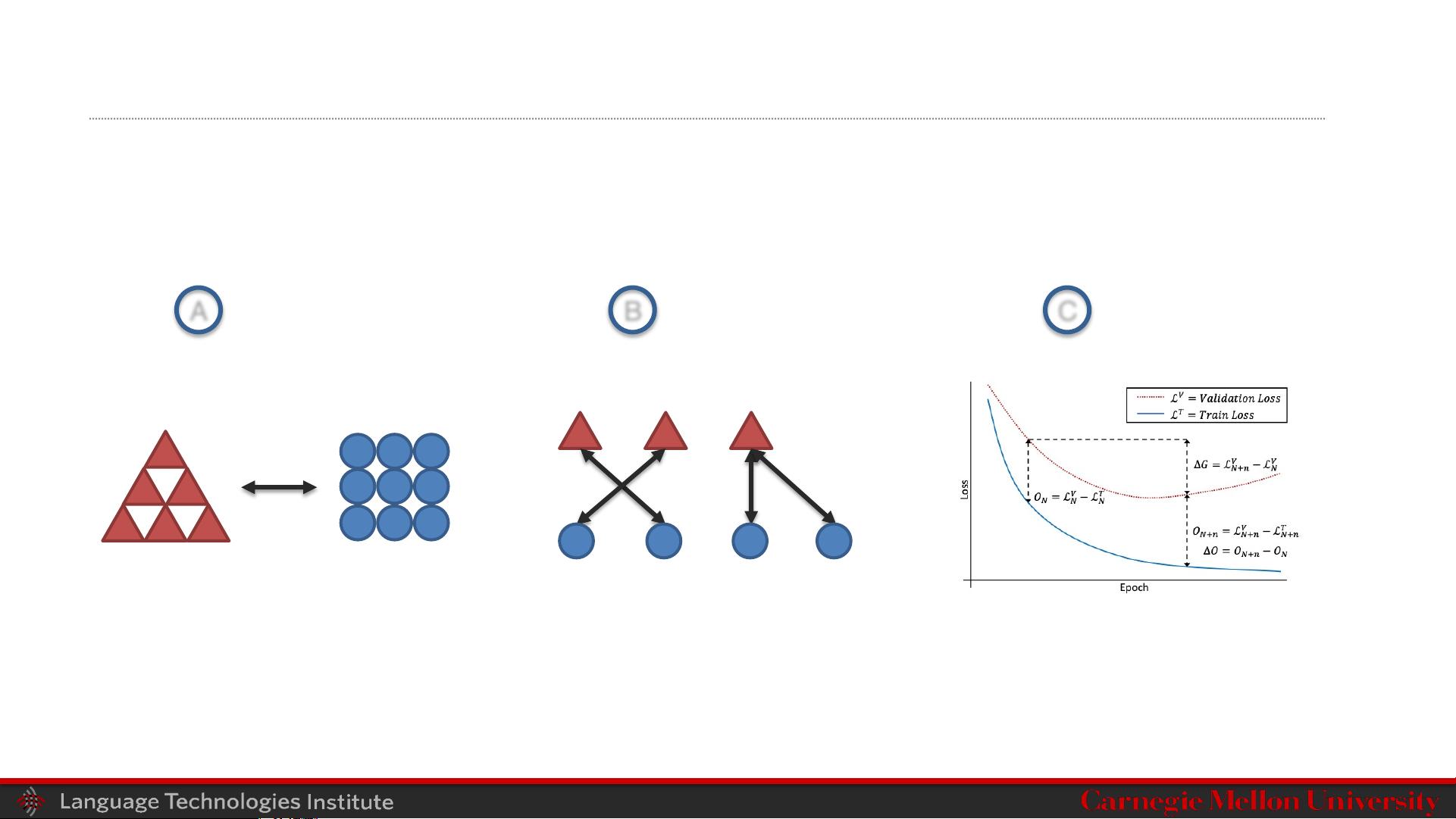
2
Quantification
Definition: Empirical and theoretical study to better understand heterogeneity,
cross-modal interactions, and the multimodal learning process.
Learning
C
Heter ogeneity
A
In teract ions
B
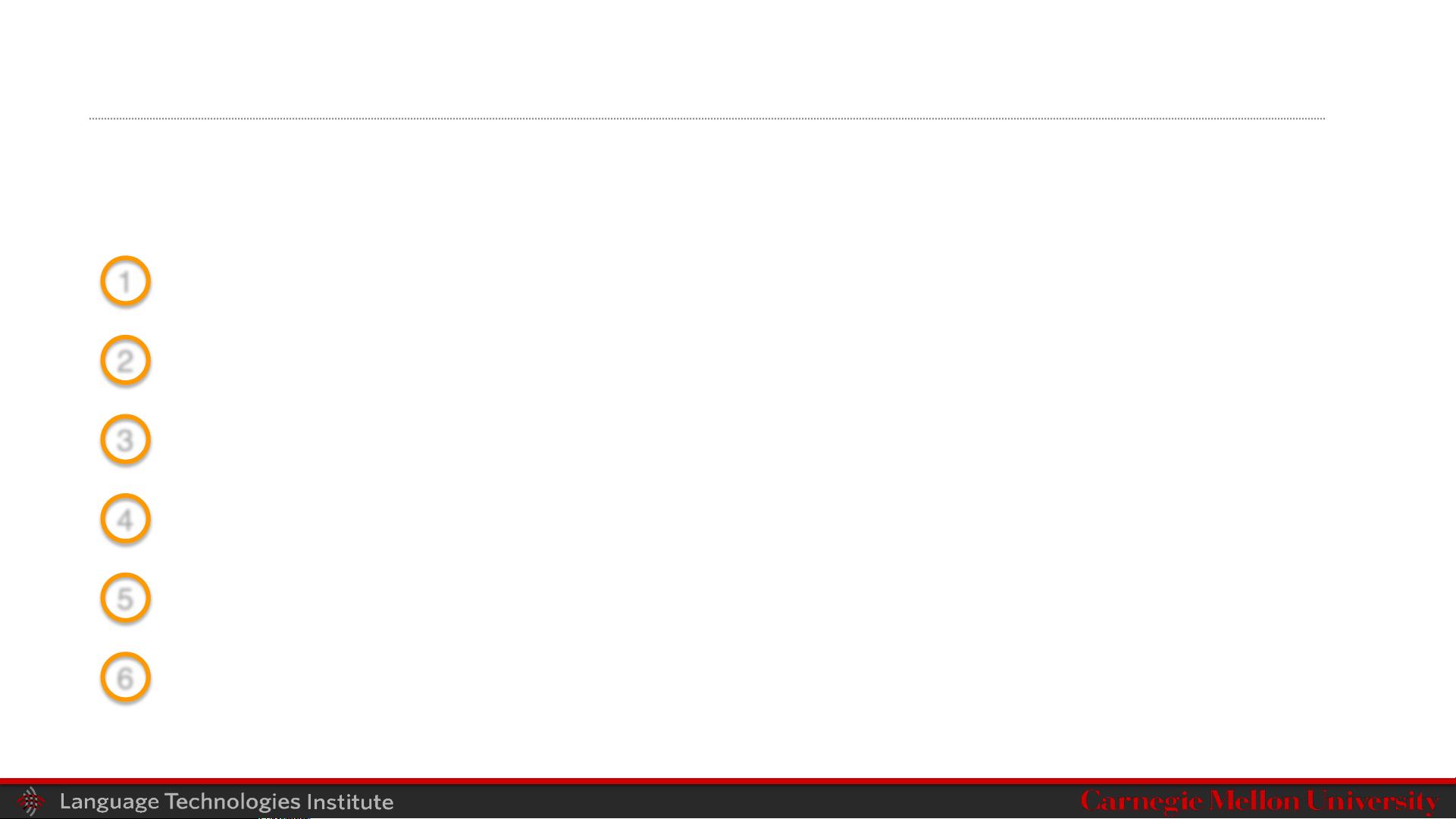
3
Sub-Challenge 1: Heterogeneity
1
Structure: static, temporal, spatial, hierarchical, invariances
2 Representation space: discrete, continuous, interpretable
3 Information: entropy, density, information overlap, range
4 Precision: sampling rate, resolution, granularity
5 Noise: uncertainty, signal-to-noise ratio, missing data
6 Relevance: task relevance, context dependence
Definition: Quantifying the dimensions of heterogeneity in multimodal datasets and
how they subsequently influence modeling and learning.
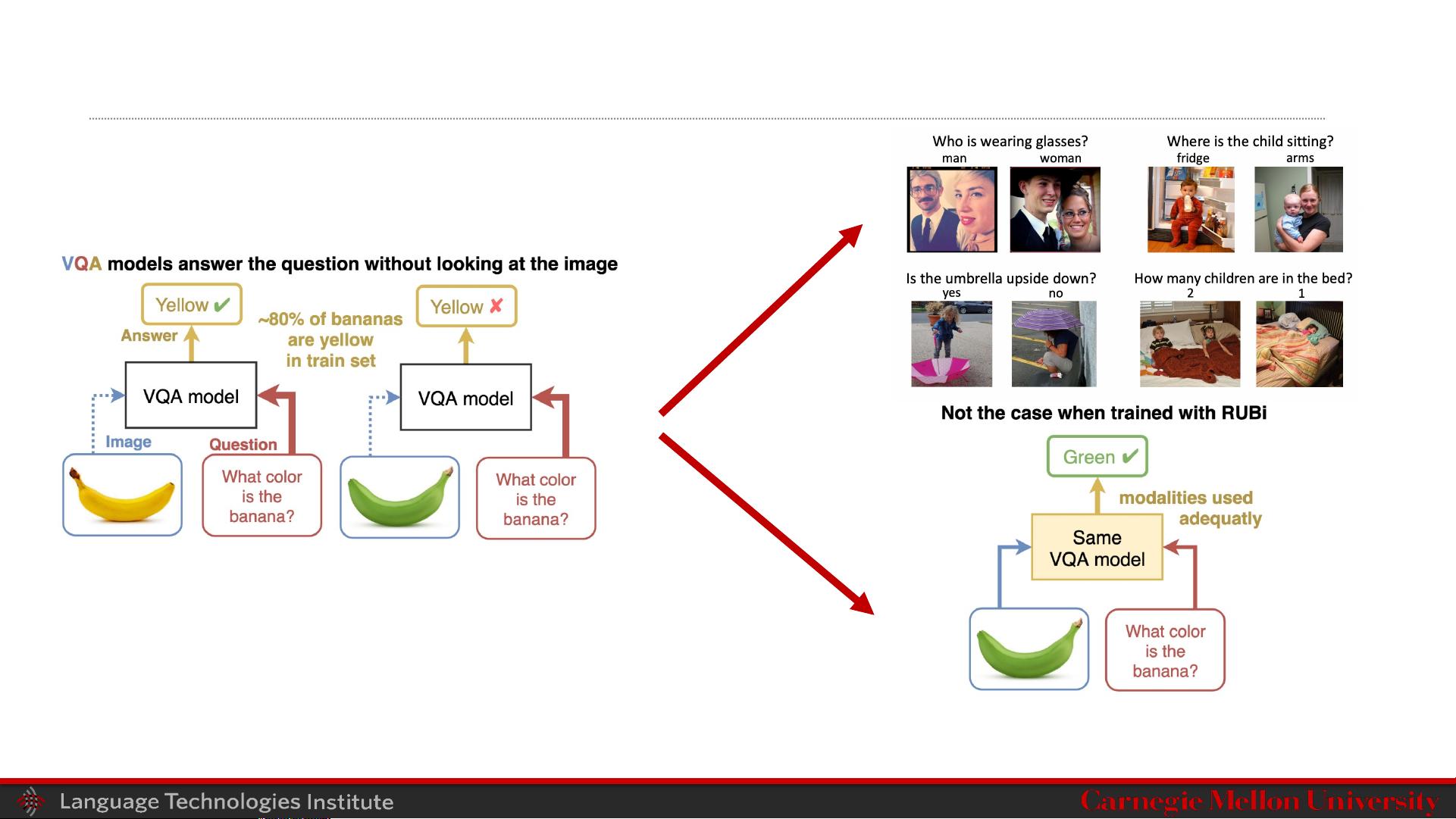
4
Unimodal biases and modality collapse
(recall information and relevance)
[Goyal et al., Making the V in VQA Matter: Elevating the Role of Image Understanding in Visual Question Answering. CVPR 2017]
Modality Biases
[Javaloy et al., Mitigating Modality Collapse in Multimodal VAEs via Impartial Optimization. ICML 2022]
[Wu et al., Characterizing and Overcoming the Greedy Nature of Learning in Multi-modal Deep Neural Networks. ICML 2022]
Balancing
modalities
Balancing
training
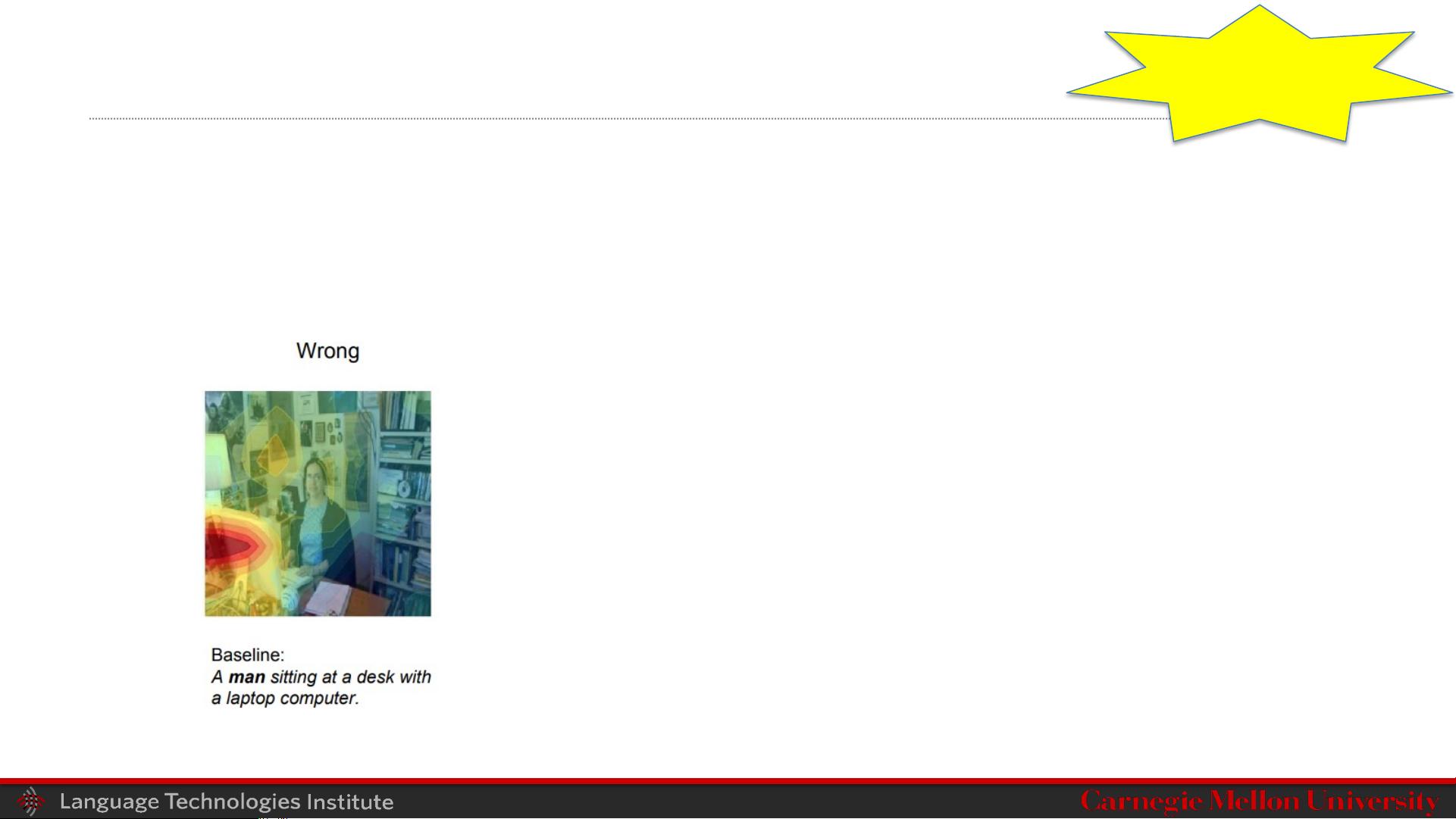
5
Fairness and social biases – unimodal social biases
(recall information and relevance)
[Hendricks et al., Women also Snowboard: Overcoming Bias in Captioning Models. ECCV 2018]
Finding: Image captioning models capture spurious correlations
between gender and generated actions
Modality Biases
Open
challenges
- 1
- 2
前往页