// OpenNN: Open Neural Networks Library
// www.opennn.net
//
// N U M E R I C A L D I F F E R E N T I A T I O N C L A S S H E A D E R
//
// Artificial Intelligence Techniques SL
// artelnics@artelnics.com
#ifndef NUMERICALDIFFERENTIATION_H
#define NUMERICALDIFFERENTIATION_H
// System includes
#include<iostream>
#include<vector>
#include<vector>
#include<limits>
#include<cstddef>
// OpenNN includes
#include "config.h"
using namespace std;
using namespace Eigen;
namespace OpenNN
{
/// This class contains methods for numerical differentiation of functions.
/// In particular it implements the forward and central differences methods for derivatives, Jacobians, hessians or hessian forms.
class NumericalDifferentiation
{
public:
// Constructors
explicit NumericalDifferentiation();
// Destructor
virtual ~NumericalDifferentiation();
/// Enumeration of available methods for numerical differentiation.
enum NumericalDifferentiationMethod{ForwardDifferences, CentralDifferences};
const NumericalDifferentiationMethod& get_numerical_differentiation_method() const;
string write_numerical_differentiation_method() const;
const Index& get_precision_digits() const;
const bool& get_display() const;
void set(const NumericalDifferentiation&);
void set_numerical_differentiation_method(const NumericalDifferentiationMethod&);
void set_numerical_differentiation_method(const string&);
void set_precision_digits(const Index&);
void set_display(const bool&);
void set_default();
type calculate_eta() const;
type calculate_h(const type&) const;
Tensor<type, 1> calculate_h(const Tensor<type, 1>&) const;
Tensor<type, 2> calculate_h(const Tensor<type, 2>&) const;
Tensor<type, 4> calculate_h(const Tensor<type, 4>&) const;
Tensor<type, 1> calculate_backward_differences_derivatives(const Tensor<type, 1>&, const Tensor<type, 1>&) const;
// Serialization methods
void from_XML(const tinyxml2::XMLDocument&);
void write_XML(tinyxml2::XMLPrinter&) const;
/// Returns the derivative of a function using the forward differences method.
/// @param t Object constructor containing the member method to differentiate.
/// @param f Pointer to the member method.
/// @param x Differentiation point.
template<class T>
type calculate_forward_differences_derivatives(const T& t, type(T::*f)(const type&) const, const type& x) const
{
const type y = (t.*f)(x);
const type h = calculate_h(x);
const type y_forward = (t.*f)(x + h);
const type d = (y_forward - y)/h;
return d;
}
/// Returns the derivative of a function using the central differences method.
/// @param t Object constructor containing the member method to differentiate.
/// @param f Pointer to the member method.
/// @param x Differentiation point.
template<class T>
type calculate_central_differences_derivatives(const T& t, type(T::*f)(const type&) const , const type& x) const
{
const type h = calculate_h(x);
const type y_forward = (t.*f)(x+h);
const type y_backward = (t.*f)(x-h);
const type d = (y_forward - y_backward)/(static_cast<type>(2.0)*h);
return d;
}
/// Returns the derivative of a function acording to the numerical differentiation method to be used.
/// @param t Object constructor containing the member method to differentiate.
/// @param f Pointer to the member method.
/// @param x Differentiation point.
template<class T>
type calculate_derivatives(const T& t, type(T::*f)(const type&) const , const type& x) const
{
switch(numerical_differentiation_method)
{
case ForwardDifferences:
{
return calculate_forward_differences_derivatives(t, f, x);
}
case CentralDifferences:
{
return calculate_central_differences_derivatives(t, f, x);
}
}
return 0.0;
}
/// Returns the derivatives of a vector function using the forward differences method.
/// @param t Object constructor containing the member method to differentiate.
/// @param f Pointer to the member method.
/// @param x Input vector.
template<class T>
Tensor<type, 1> calculate_forward_differences_derivatives(const T& t, Tensor<type, 1>(T::*f)(const Tensor<type, 1>&) const, const Tensor<type, 1>& x) const
{
const Tensor<type, 1> h = calculate_h(x);
const Tensor<type, 1> y = (t.*f)(x);
const Tensor<type, 1> x_forward = x + h;
const Tensor<type, 1> y_forward = (t.*f)(x_forward);
const Tensor<type, 1> d = (y_forward - y)/h;
return d;
}
template<class T>
Tensor<type, 2> calculate_forward_differences_derivatives(const T& t, Tensor<type, 2>(T::*f)(const Tensor<type, 2>&) const, const Tensor<type, 2>& x) const
{
const Tensor<type, 2> h = calculate_h(x);
const Tensor<type, 2> y = (t.*f)(x);
const Tensor<type, 2> x_forward = x + h;
const Tensor<type, 2> y_forward = (t.*f)(x_forward);
const Tensor<type, 2> d = (y_forward - y)/h;
return d;
}
/// Returns the derivatives of a vector function using the central differences method.
/// @param t : Object constructor containing the member method to differentiate.
/// @param f: Pointer to the member method.
/// @param x: Input vector.
template<class T>
Tensor<type, 1> calculate_central_differences_derivatives(const T& t, Tensor<type, 1>(T::*f)(const Tensor<type, 1>&) const, const Tensor<type, 1>& x) const
{
const Tensor<type, 1> h = calculate_h(x);
const Tensor<type, 1> x_forward = x + h;
const Tensor<type, 1> x_backward = x - h;
const Tensor<type, 1> y_forward = (t.*f)(x_forward);
const Tensor<type, 1> y_backward = (t.*f)(x_backward);
const Tensor<type, 1> y = (t.*f)(x);
const Tensor<type, 1> d = (y_forward - y_backward)/(static_cast<type>(2.0)*h);
return d;
}
template<class T>
Tensor<type, 2> calculate_central_differences_derivatives(const T& t, Tensor<type, 2>(T::*f)(const Tensor<type, 2>&) const, const Tensor<type, 2>& x) const
{
const Tensor<type, 2> h = calculate_h(x);
const Tensor<type, 2> x_forward = x + h;
const Tensor<type, 2> x_backward = x - h;
const Tensor<type, 2> y_forward = (t.*f)(x_forward);
const Tensor<type, 2> y_backward = (t.*f)(x_backward);
const Tensor<type, 2> y = (t.*f)(x);
const Tensor<type, 2> d = (y_forward - y_backward)/(static_cast<type>(2.0)*h);
return d;
}
template<class T>
Tensor<type, 4> calculate_central_differences_derivatives(const T& t, Tensor<type, 4>(T::*f)(const Tensor<type, 4>&) const, const Tensor<type, 4>& x) const
{
const Tensor<type, 4> h = calculate_h(x);
const Tensor<type, 4> x_forward = x + h;
const Tensor<type, 4> x_backward = x - h;
const Tensor<type, 4> y_forward = (t.*f)(x_forward);
const Tensor<type, 4> y_backward = (t.*f)(x_backward);
const Tensor<type, 4> y = (t.*f)(x);
const Tensor<type, 4> d = (y_forward - y_backward)/(static_cast<type>(2.0)*h);
return d;
}
/// Returns the derivatives of a vector function acording to the numerical differentiation method to be used.
/// @param t : Object constructor containing the member method to differentiate.
/// @param f: Pointer to the member method.
/// @param x: Input vector.
template<class T>
Tensor<type, 1> calculate_derivatives(const T& t, Tensor<type, 1>(T::*f)(const Tensor<type, 1>&) const, const Tensor<type, 1>& x) const
{
switch(numerical_differentiation_method)
{
case ForwardDifferences:
{
return calculate_forward_differences_derivatives(t, f, x);
}
case CentralDifferences:
{
return calculate_central_di
没有合适的资源?快使用搜索试试~ 我知道了~
openNN-v5.0.5【无需积分值】
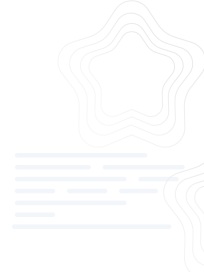
共47个文件
h:46个
lib:1个

需积分: 0 7 下载量 108 浏览量
2022-07-20
11:10:06
上传
评论
收藏 5.18MB ZIP 举报
温馨提示
【无需积分值】 openNN是高效的C++神经网路库。 已在windows 64位下成功 lib文件夹中是所需的静态链接库 include文件夹中是所需的全部头文件 具体使用方法见https://blog.csdn.net/weixin_43325228/article/details/125887630
资源详情
资源评论
资源推荐
收起资源包目录



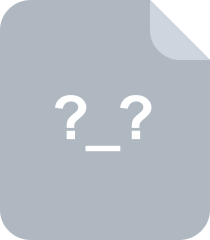
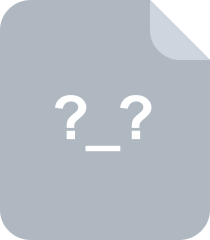
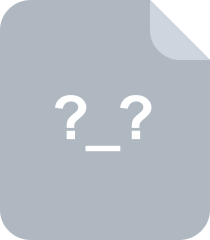
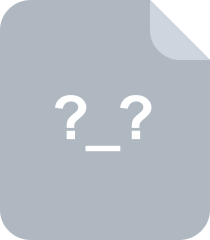
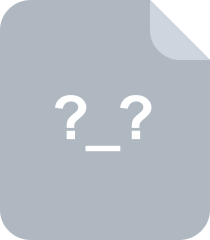
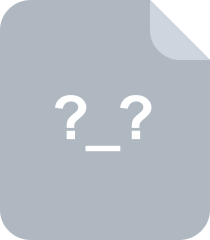
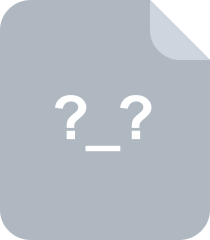
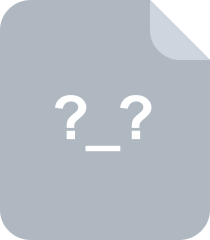
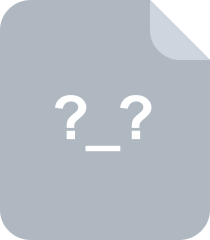
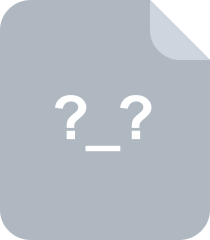
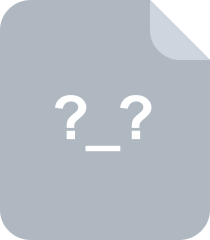
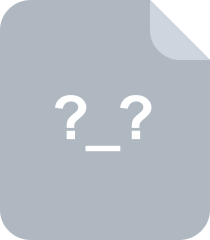
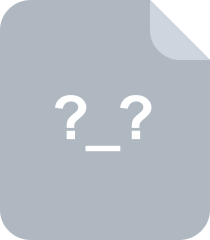
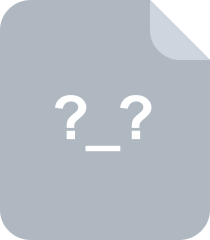
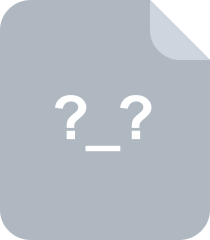
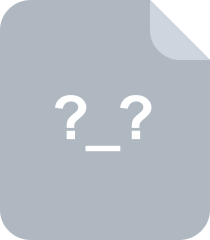
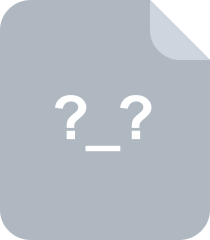
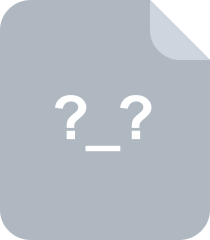
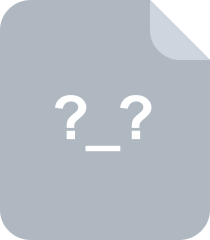
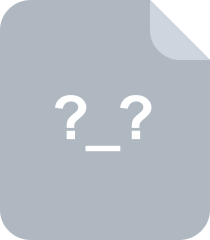
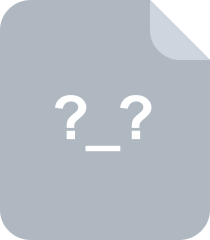
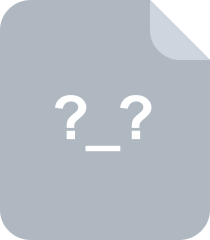
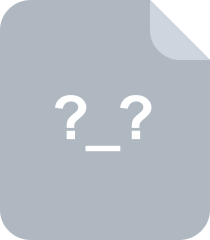
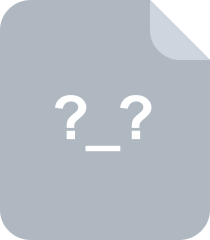
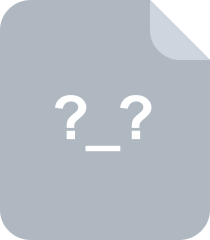
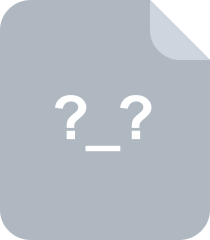
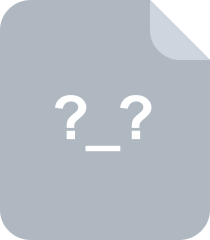
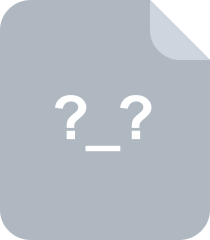
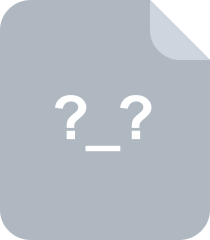
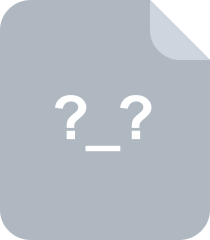
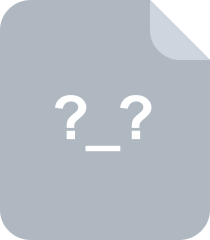
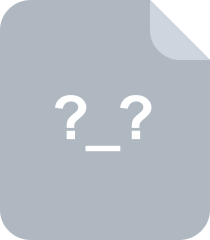
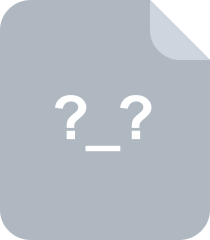
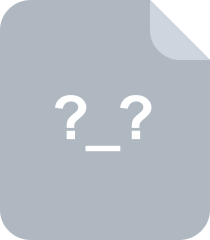
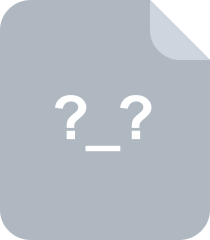
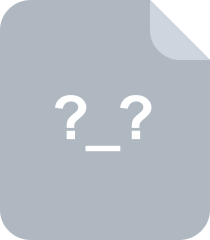
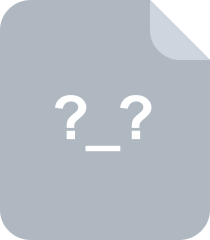
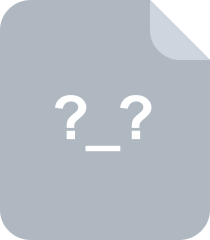
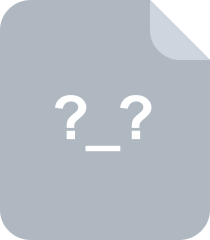
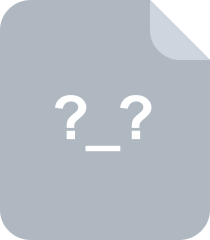
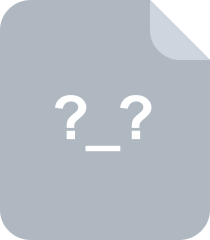
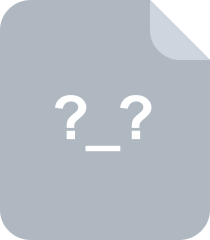
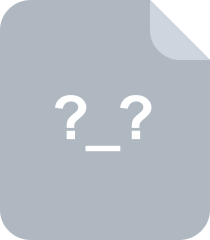
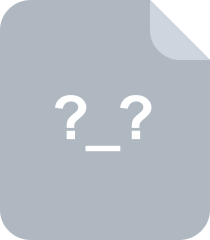
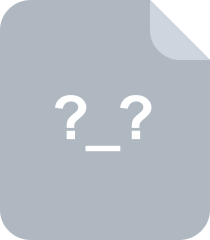
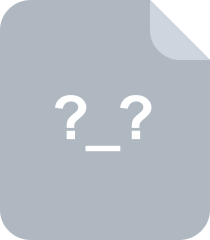

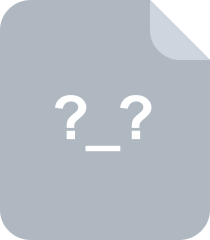
共 47 条
- 1
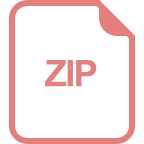
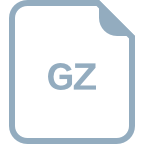
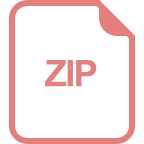
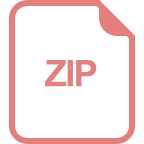
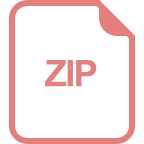
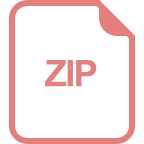
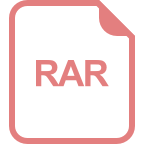
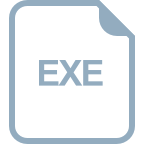
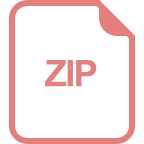
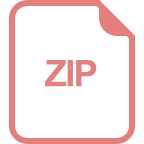
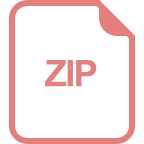
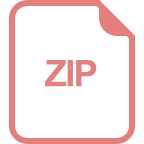
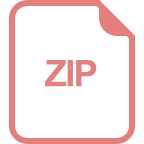
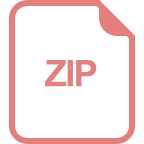
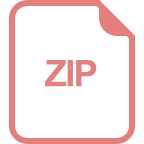
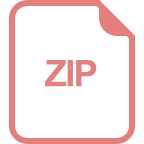
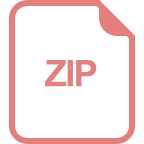
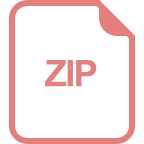
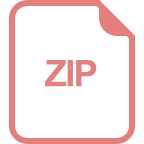
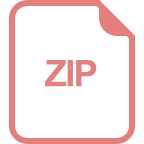
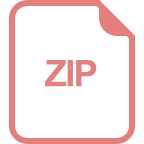
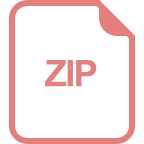
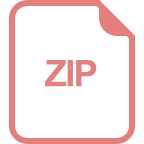

jedi-knight
- 粉丝: 266
- 资源: 4
上传资源 快速赚钱
我的内容管理 展开
我的资源 快来上传第一个资源
我的收益
登录查看自己的收益我的积分 登录查看自己的积分
我的C币 登录后查看C币余额
我的收藏
我的下载
下载帮助

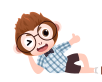
最新资源
- 5G建设和AI技术推动下,中证5G通信ETF的投资价值探讨
- Python项目之淘宝模拟登录.zip
- 课程设计项目:python+QT实现的小型编译器.zip
- (源码)基于AVR ATmega644的智能卡AES解密系统.zip
- (源码)基于C++插件框架的计算与打印系统.zip
- (源码)基于Spring Boot和Vue的苍穹外卖管理系统.zip
- (源码)基于wxWidgets库的QMiniIDE游戏开发环境管理系统.zip
- 通过C++实现原型模式(Prototype Pattern).rar
- 学习记录111111111111111111111111
- 通过java实现原型模式(Prototype Pattern).rar
资源上传下载、课程学习等过程中有任何疑问或建议,欢迎提出宝贵意见哦~我们会及时处理!
点击此处反馈


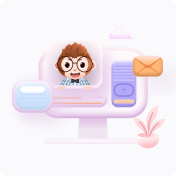
安全验证
文档复制为VIP权益,开通VIP直接复制

评论0