# 导入工具包
import numpy as np
import argparse
import cv2
# 设置参数
ap = argparse.ArgumentParser()
ap.add_argument("-i", "--image",default="images/page.jpg",
help = "Path to the image to be scanned")
args = vars(ap.parse_args())
def order_points(pts):
# 一共4个坐标点
rect = np.zeros((4, 2), dtype = "float32")
# 按顺序找到对应坐标0123分别是 左上,右上,右下,左下
# 计算左上,右下
s = pts.sum(axis = 1)
rect[0] = pts[np.argmin(s)]
rect[2] = pts[np.argmax(s)]
# 计算右上和左下
diff = np.diff(pts, axis = 1)
rect[1] = pts[np.argmin(diff)]
rect[3] = pts[np.argmax(diff)]
return rect
def four_point_transform(image, pts):
# 获取输入坐标点
rect = order_points(pts)
(tl, tr, br, bl) = rect
# 计算输入的w和h值
widthA = np.sqrt(((br[0] - bl[0]) ** 2) + ((br[1] - bl[1]) ** 2))
widthB = np.sqrt(((tr[0] - tl[0]) ** 2) + ((tr[1] - tl[1]) ** 2))
maxWidth = max(int(widthA), int(widthB))
heightA = np.sqrt(((tr[0] - br[0]) ** 2) + ((tr[1] - br[1]) ** 2))
heightB = np.sqrt(((tl[0] - bl[0]) ** 2) + ((tl[1] - bl[1]) ** 2))
maxHeight = max(int(heightA), int(heightB))
# 变换后对应坐标位置
dst = np.array([
[0, 0],
[maxWidth - 1, 0],
[maxWidth - 1, maxHeight - 1],
[0, maxHeight - 1]], dtype = "float32")
# 计算变换矩阵
M = cv2.getPerspectiveTransform(rect, dst)
warped = cv2.warpPerspective(image, M, (maxWidth, maxHeight))
# 返回变换后结果
return warped
def resize(image, width=None, height=None, inter=cv2.INTER_AREA):
dim = None
(h, w) = image.shape[:2]
if width is None and height is None:
return image
if width is None:
r = height / float(h)
dim = (int(w * r), height)
else:
r = width / float(w)
dim = (width, int(h * r))
resized = cv2.resize(image, dim, interpolation=inter)
return resized
if __name__ == '__main__':
# 读取输入
image = cv2.imread(args["image"])
#坐标也会相同变化
ratio = image.shape[0] / 500.0
orig = image.copy()
image = resize(orig, height = 500)
# 预处理
gray = cv2.cvtColor(image, cv2.COLOR_BGR2GRAY)
gray = cv2.GaussianBlur(gray, (5, 5), 0)
edged = cv2.Canny(gray, 75, 200)
# 展示预处理结果
print("STEP 1: 边缘检测")
cv2.imshow("Image", image)
cv2.imshow("Edged", edged)
cv2.waitKey(0)
cv2.destroyAllWindows()
# 轮廓检测 opencv3
# cnts = cv2.findContours(edged.copy(), cv2.RETR_LIST, cv2.CHAIN_APPROX_SIMPLE)[1][0]
# cnts = sorted(cnts, key = cv2.contourArea, reverse = True)[:5]
# 使用OpenCV 4.x的方式来调用findContours
contours, hierarchy = cv2.findContours(edged.copy(), cv2.RETR_LIST, cv2.CHAIN_APPROX_SIMPLE)
# 确保轮廓是适当的数据类型
cnts = [np.array(cnt, dtype='float32') for cnt in contours]
# 排序并选择最大的5个轮廓
cnts = sorted(cnts, key=cv2.contourArea, reverse=True)[:5]
# 遍历轮廓
screenCnt = None
for c in cnts:
# 计算轮廓近似
peri = cv2.arcLength(c, True)
# C表示输入的点集
# epsilon表示从原始轮廓到近似轮廓的最大距离,它是一个准确度参数
# True表示封闭的
approx = cv2.approxPolyDP(c, 0.02 * peri, True)
# 4个点的时候就拿出来
if len(approx) == 4:
screenCnt = approx.astype(int)
break
if screenCnt is not None:
# 展示结果
print("STEP 2: 获取轮廓")
# print(screenCnt)
cv2.drawContours(image, [screenCnt], -1, (0, 255, 0), 2)
cv2.imshow("Outline", image)
cv2.waitKey(0)
cv2.destroyAllWindows()
# 透视变换
warped = four_point_transform(orig, screenCnt.reshape(4, 2) * ratio)
# 二值处理
warped = cv2.cvtColor(warped, cv2.COLOR_BGR2GRAY)
ref = cv2.threshold(warped, 100, 255, cv2.THRESH_BINARY)[1]
cv2.imwrite('scan.jpg', ref)
# 展示结果
print("STEP 3: 变换")
cv2.imshow("Original", resize(orig, height = 650))
cv2.imshow("Scanned", resize(ref, height = 650))
cv2.waitKey(0)


小陈phd
- 粉丝: 3190
- 资源: 19
最新资源
- 空中俯视物体检测14-YOLO(v5至v9)、COCO、CreateML、Darknet、Paligemma数据集合集.rar
- labview框架 产线MES系统 物料管理,排产计划,设备管理,报表管理,功能齐全 扫码追溯 PLC通信 数据库存储 标签打印
- 免费下载:Marvel Studios The Marvel Cinematic Universe an Official Timeline (Anthony Breznican
- 免费下载:Radiant The Life and Line of Keith Haring (Brad Gooch)_jYShi.zip
- docx工具 DocX工具包是LabVIEW的一款附加软件 该附 加软件提供了一个LabVIEW API,可用于创建 Microsoft Word Open XML格式文档(docx)文 件 该附加软
- 免费下载:Infrared and Raman Spectroscopy (Günter G. Hoffmann)_48Uzo.zip
- 免费下载:Cities in the Sky The Quest to Build the Worlds Tallest Skyscrapers (Jason M. Barr)_NwbMo.zip
- 免费下载:Avengers Forever Infinity Comic (2022) 4 (Jason Aaron)_8KxTK.zip
- 免费下载:Ms. Marvel 1 (Sana Amanat, Stephen Wacker, G. Willow Wilson etc.)_IGJr0.zip
- 免费下载:普通高中教科书 信息技术 必修2 信息系统与社会 (上海市中小学(幼儿园)_1riYg.zip
- 免费下载:O Contador de Histórias Memórias de Vida e Música (Dave Grohl)_3QKQD.zip
- 免费下载:Hilma af Klint a Biography (Julia Voss)_tFy2T.zip
- 免费下载:Deliver Me from Nowhere (Warren Zanes)_EYvAJ.zip
- 免费下载:Earth to Moon A Memoir (Moon Unit Zappa)_ZEcu6.zip
- 免费下载:You Are (Not) Deadpool (Tim Dedopulos)_ut1SY.zip
- 免费下载:(한영 AI교차번역) Physics 100 Ideas in 100 Words (DK)_cENu8.zip
资源上传下载、课程学习等过程中有任何疑问或建议,欢迎提出宝贵意见哦~我们会及时处理!
点击此处反馈


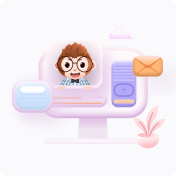