# Pytorch-cifar100
practice on cifar100 using pytorch
## Requirements
This is my experiment eviroument
- python3.6
- pytorch1.6.0+cu101
- tensorboard 2.2.2(optional)
## Usage
### 1. enter directory
```bash
$ cd pytorch-cifar100
```
### 2. dataset
I will use cifar100 dataset from torchvision since it's more convenient, but I also
kept the sample code for writing your own dataset module in dataset folder, as an
example for people don't know how to write it.
### 3. run tensorbard(optional)
Install tensorboard
```bash
$ pip install tensorboard
$ mkdir runs
Run tensorboard
$ tensorboard --logdir='runs' --port=6006 --host='localhost'
```
### 4. train the model
You need to specify the net you want to train using arg -net
```bash
# use gpu to train vgg16
$ python train.py -net vgg16 -gpu
```
sometimes, you might want to use warmup training by set ```-warm``` to 1 or 2, to prevent network
diverge during early training phase.
The supported net args are:
```
squeezenet
mobilenet
mobilenetv2
shufflenet
shufflenetv2
vgg11
vgg13
vgg16
vgg19
densenet121
densenet161
densenet201
googlenet
inceptionv3
inceptionv4
inceptionresnetv2
xception
resnet18
resnet34
resnet50
resnet101
resnet152
preactresnet18
preactresnet34
preactresnet50
preactresnet101
preactresnet152
resnext50
resnext101
resnext152
attention56
attention92
seresnet18
seresnet34
seresnet50
seresnet101
seresnet152
nasnet
```
Normally, the weights file with the best accuracy would be written to the disk with name suffix 'best'(default in checkpoint folder).
### 5. test the model
Test the model using test.py
```bash
$ python test.py -net vgg16 -weights path_to_vgg16_weights_file
```
## Implementated NetWork
- vgg [Very Deep Convolutional Networks for Large-Scale Image Recognition](https://arxiv.org/abs/1409.1556v6)
- googlenet [Going Deeper with Convolutions](https://arxiv.org/abs/1409.4842v1)
- inceptionv3 [Rethinking the Inception Architecture for Computer Vision](https://arxiv.org/abs/1512.00567v3)
- inceptionv4, inception_resnet_v2 [Inception-v4, Inception-ResNet and the Impact of Residual Connections on Learning](https://arxiv.org/abs/1602.07261)
- xception [Xception: Deep Learning with Depthwise Separable Convolutions](https://arxiv.org/abs/1610.02357)
- resnet [Deep Residual Learning for Image Recognition](https://arxiv.org/abs/1512.03385v1)
- resnext [Aggregated Residual Transformations for Deep Neural Networks](https://arxiv.org/abs/1611.05431v2)
- resnet in resnet [Resnet in Resnet: Generalizing Residual Architectures](https://arxiv.org/abs/1603.08029v1)
- densenet [Densely Connected Convolutional Networks](https://arxiv.org/abs/1608.06993v5)
- shufflenet [ShuffleNet: An Extremely Efficient Convolutional Neural Network for Mobile Devices](https://arxiv.org/abs/1707.01083v2)
- shufflenetv2 [ShuffleNet V2: Practical Guidelines for Efficient CNN Architecture Design](https://arxiv.org/abs/1807.11164v1)
- mobilenet [MobileNets: Efficient Convolutional Neural Networks for Mobile Vision Applications](https://arxiv.org/abs/1704.04861)
- mobilenetv2 [MobileNetV2: Inverted Residuals and Linear Bottlenecks](https://arxiv.org/abs/1801.04381)
- residual attention network [Residual Attention Network for Image Classification](https://arxiv.org/abs/1704.06904)
- senet [Squeeze-and-Excitation Networks](https://arxiv.org/abs/1709.01507)
- squeezenet [SqueezeNet: AlexNet-level accuracy with 50x fewer parameters and <0.5MB model size](https://arxiv.org/abs/1602.07360v4)
- nasnet [Learning Transferable Architectures for Scalable Image Recognition](https://arxiv.org/abs/1707.07012v4)
## Training Details
I didn't use any training tricks to improve accuray, if you want to learn more about training tricks,
please refer to my another [repo](https://github.com/weiaicunzai/Bag_of_Tricks_for_Image_Classification_with_Convolutional_Neural_Networks), contains
various common training tricks and their pytorch implementations.
I follow the hyperparameter settings in paper [Improved Regularization of Convolutional Neural Networks with Cutout](https://arxiv.org/abs/1708.04552v2), which is init lr = 0.1 divide by 5 at 60th, 120th, 160th epochs, train for 200
epochs with batchsize 128 and weight decay 5e-4, Nesterov momentum of 0.9. You could also use the hyperparameters from paper [Regularizing Neural Networks by Penalizing Confident Output Distributions](https://arxiv.org/abs/1701.06548v1) and [Random Erasing Data Augmentation](https://arxiv.org/abs/1708.04896v2), which is initial lr = 0.1, lr divied by 10 at 150th and 225th epochs, and training for 300 epochs with batchsize 128, this is more commonly used. You could decrese the batchsize to 64 or whatever suits you, if you dont have enough gpu memory.
You can choose whether to use TensorBoard to visualize your training procedure
## Results
The result I can get from a certain model, since I use the same hyperparameters to train all the networks, some networks might not get the best result from these hyperparameters, you could try yourself by finetuning the hyperparameters to get
better result.
|dataset|network|params|top1 err|top5 err|memory|epoch(lr = 0.1)|epoch(lr = 0.02)|epoch(lr = 0.004)|epoch(lr = 0.0008)|total epoch|
|:---:|:---:|:---:|:---:|:---:|:---:|:---:|:---:|:---:|:---:|:---:
|cifar100|mobilenet|3.3M|34.02|10.56|0.69GB|60|60|40|40|200|
|cifar100|mobilenetv2|2.36M|31.92|09.02|0.84GB|60|60|40|40|200|
|cifar100|squeezenet|0.78M|30.59|8.36|0.73GB|60|60|40|40|200|
|cifar100|shufflenet|1.0M|29.94|8.35|0.84GB|60|60|40|40|200|
|cifar100|shufflenetv2|1.3M|30.49|8.49|0.78GB|60|60|40|40|200|
|cifar100|vgg11_bn|28.5M|31.36|11.85|1.98GB|60|60|40|40|200|
|cifar100|vgg13_bn|28.7M|28.00|9.71|1.98GB|60|60|40|40|200|
|cifar100|vgg16_bn|34.0M|27.07|8.84|2.03GB|60|60|40|40|200|
|cifar100|vgg19_bn|39.0M|27.77|8.84|2.08GB|60|60|40|40|200|
|cifar100|resnet18|11.2M|24.39|6.95|3.02GB|60|60|40|40|200|
|cifar100|resnet34|21.3M|23.24|6.63|3.22GB|60|60|40|40|200|
|cifar100|resnet50|23.7M|22.61|6.04|3.40GB|60|60|40|40|200|
|cifar100|resnet101|42.7M|22.22|5.61|3.72GB|60|60|40|40|200|
|cifar100|resnet152|58.3M|22.31|5.81|4.36GB|60|60|40|40|200|
|cifar100|preactresnet18|11.3M|27.08|8.53|3.09GB|60|60|40|40|200|
|cifar100|preactresnet34|21.5M|24.79|7.68|3.23GB|60|60|40|40|200|
|cifar100|preactresnet50|23.9M|25.73|8.15|3.42GB|60|60|40|40|200|
|cifar100|preactresnet101|42.9M|24.84|7.83|3.81GB|60|60|40|40|200|
|cifar100|preactresnet152|58.6M|22.71|6.62|4.20GB|60|60|40|40|200|
|cifar100|resnext50|14.8M|22.23|6.00|1.91GB|60|60|40|40|200|
|cifar100|resnext101|25.3M|22.22|5.99|2.63GB|60|60|40|40|200|
|cifar100|resnext152|33.3M|22.40|5.58|3.18GB|60|60|40|40|200|
|cifar100|attention59|55.7M|33.75|12.90|3.47GB|60|60|40|40|200|
|cifar100|attention92|102.5M|36.52|11.47|3.88GB|60|60|40|40|200|
|cifar100|densenet121|7.0M|22.99|6.45|1.28GB|60|60|40|40|200|
|cifar100|densenet161|26M|21.56|6.04|2.10GB|60|60|60|40|200|
|cifar100|densenet201|18M|21.46|5.9|2.10GB|60|60|40|40|200|
|cifar100|googlenet|6.2M|21.97|5.94|2.05GB|60|60|40|40|200|
|cifar100|inceptionv3|22.3M|22.81|6.39|2.26GB|60|60|40|40|200|
|cifar100|inceptionv4|41.3M|24.14|6.90|4.11GB|60|60|40|40|200|
|cifar100|inceptionresnetv2|65.4M|27.51|9.11|4.14GB|60|60|40|40|200|
|cifar100|xception|21.0M|25.07|7.32|1.67GB|60|60|40|40|200|
|cifar100|seresnet18|11.4M|23.56|6.68|3.12GB|60|60|40|40|200|
|cifar100|seresnet34|21.6M|22.07|6.12|3.29GB|60|60|40|40|200|
|cifar100|seresnet50|26.5M|21.42|5.58|3.70GB|60|60|40|40|200|
|cifar100|seresnet101|47.7M|20.98|5.41|4.39GB|60|60|40|40|200|
|cifar100|seresnet152|66.2M|20.66|5.19|5.95GB|60|60|40|40|200|
|cifar100|nasnet|5.2M|22.71|5.91|3.69GB|60|60|40|40|200|
|cifar100|wideresnet-40-10|55.9M|21.25|5.77|0.63GB|60|60|40|40|200|
没有合适的资源?快使用搜索试试~ 我知道了~
资源推荐
资源详情
资源评论
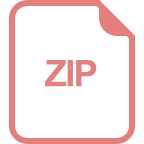
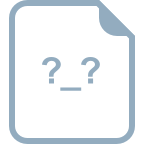
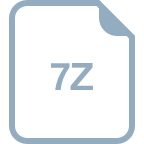
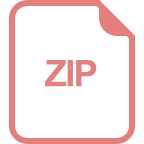
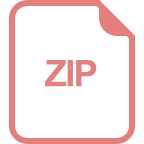
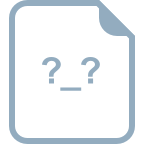
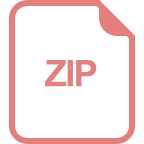
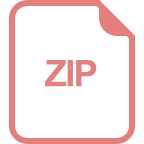
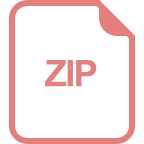
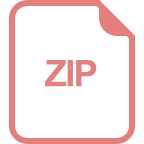
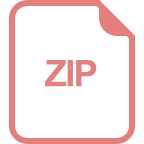
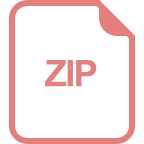
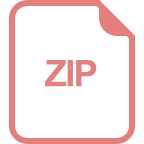
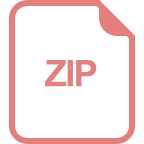
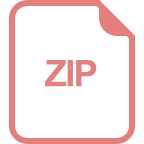
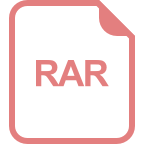
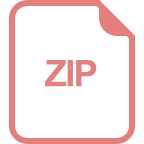
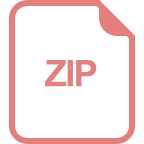
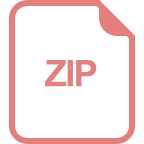
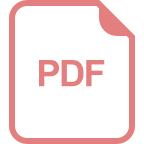
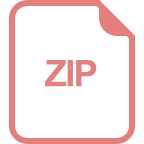
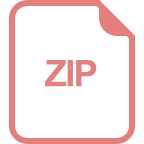
收起资源包目录



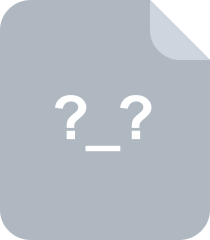
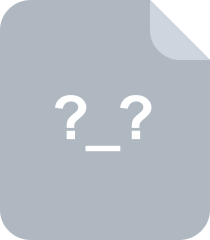
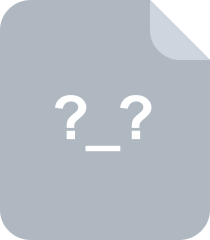

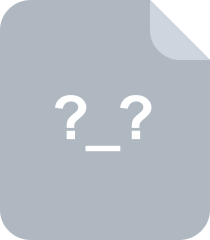
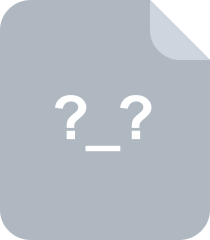
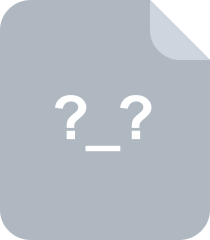
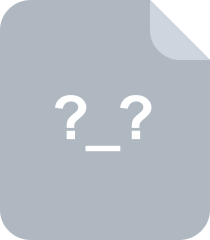
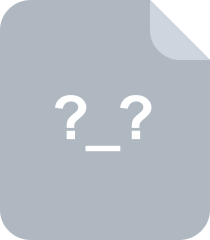
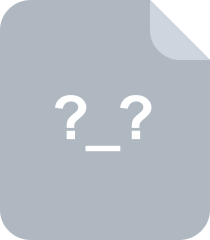
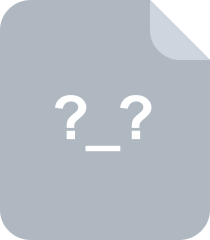
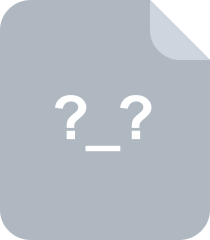
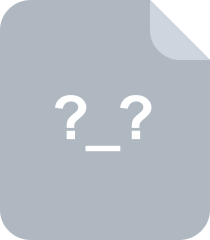
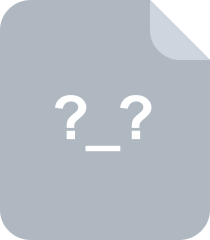
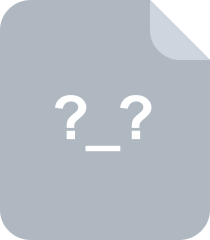
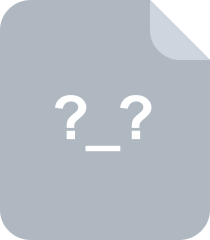
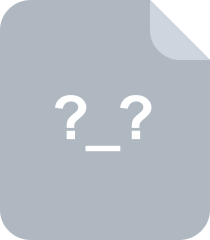
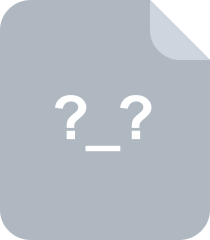
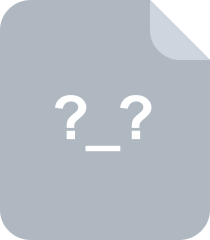
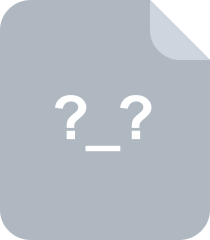
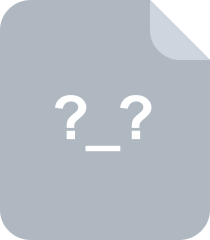
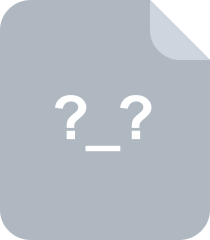
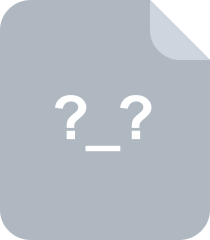
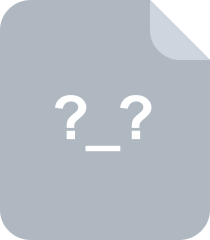
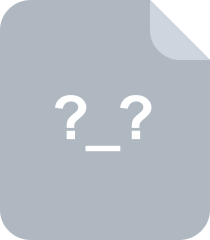
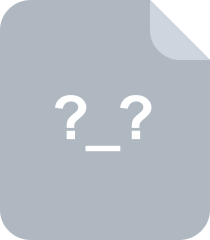
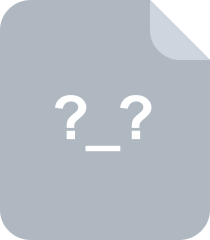
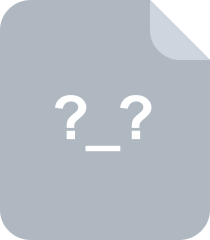
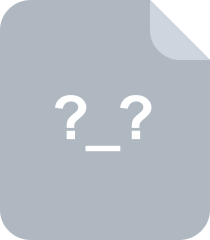
共 28 条
- 1

西西nayss
- 粉丝: 84
- 资源: 4749

下载权益

C知道特权

VIP文章

课程特权

开通VIP
上传资源 快速赚钱
我的内容管理 展开
我的资源 快来上传第一个资源
我的收益
登录查看自己的收益我的积分 登录查看自己的积分
我的C币 登录后查看C币余额
我的收藏
我的下载
下载帮助

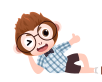
最新资源
资源上传下载、课程学习等过程中有任何疑问或建议,欢迎提出宝贵意见哦~我们会及时处理!
点击此处反馈


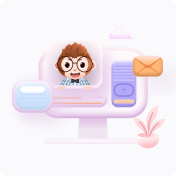
安全验证
文档复制为VIP权益,开通VIP直接复制

- 1
- 2
- 3
前往页