<img src="./SETR.png" width="800px"></img>
## SETR - Pytorch
Since the original paper (Rethinking Semantic Segmentation from a Sequence-to-Sequence Perspective with Transformers.) has no official code,I implemented SETR-Progressive UPsampling(SETR-PUP) using pytorch.
Original paper: <a href="https://arxiv.org/abs/2012.15840">Rethinking Semantic Segmentation from a Sequence-to-Sequence Perspective with Transformers.</a>
## Vit
The Vit model is also implemented, and you can use it for image classification.
## Usage SETR
```python
from SETR.transformer_seg import SETRModel
import torch
if __name__ == "__main__":
net = SETRModel(patch_size=(32, 32),
in_channels=3,
out_channels=1,
hidden_size=1024,
num_hidden_layers=8,
num_attention_heads=16,
decode_features=[512, 256, 128, 64])
t1 = torch.rand(1, 3, 256, 256)
print("input: " + str(t1.shape))
# print(net)
print("output: " + str(net(t1).shape))
```
If the output size is (1, 1, 256, 256), the code runs successfully.
## Usage Vit
```python
from SETR.transformer_seg import Vit
import torch
if __name__ == "__main__":
model = Vit(patch_size=(7, 7),
in_channels=1,
out_class=10,
hidden_size=1024,
num_hidden_layers=1,
num_attention_heads=16)
print(model)
t1 = torch.rand(1, 1, 28, 28)
print("input: " + str(t1.shape))
print("output: " + str(model(t1).shape))
```
The output shape is (1, 10).
## current examples
1. task_mnist: The simplest example, using the Vit model to classify the minst dataset.
2. task_car_seg: The example is sample segmentation task. data download: <a href="https://www.kaggle.com/c/carvana-image-masking-challenge/data">https://www.kaggle.com/c/carvana-image-masking-challenge/data</a>
## more
More examples will be updated later.
没有合适的资源?快使用搜索试试~ 我知道了~
SETR-pytorch:SETR模型的实现,原始论文
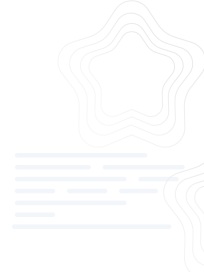
共12个文件
py:5个
pyc:2个
gitignore:1个

需积分: 48 22 下载量 181 浏览量
2021-05-23
05:22:18
上传
评论 2
收藏 184KB ZIP 举报
温馨提示
塞特-火炬 由于原始论文(使用变压器从序列到序列的角度重新思考语义分割)没有官方代码,因此我使用pytorch实现了SETR-Progressive UPsampling(SETR-PUP)。 原始论文: 维生素 Vit模型也已实现,您可以将其用于图像分类。 用法SETR from SETR . transformer_seg import SETRModel import torch if __name__ == "__main__" : net = SETRModel ( patch_size = ( 32 , 32 ), in_channels = 3 , out_channels = 1 , hidden_size = 1024 ,
资源推荐
资源详情
资源评论
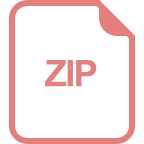
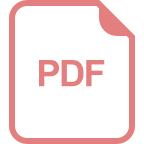
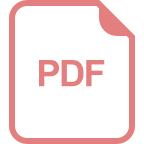
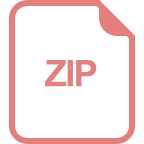
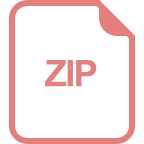
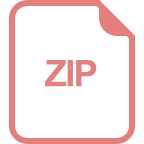
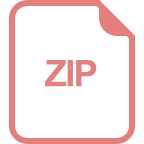
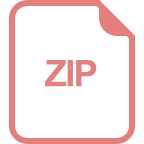
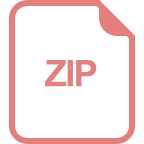
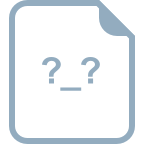
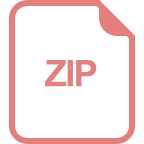
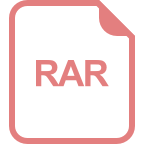
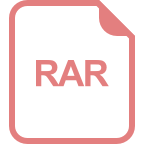
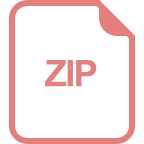
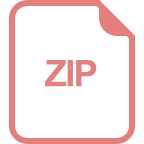
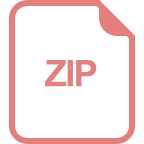
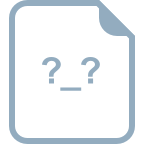
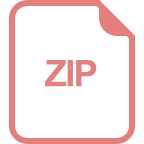
收起资源包目录


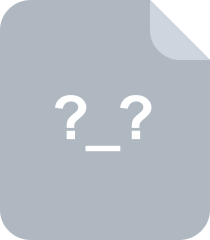
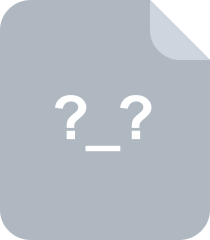

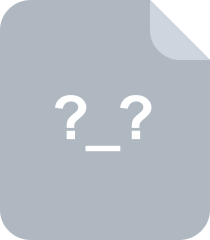

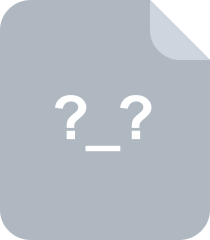
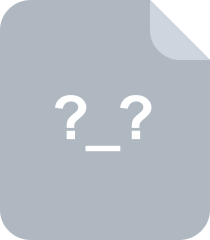
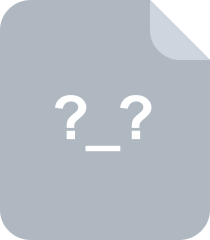

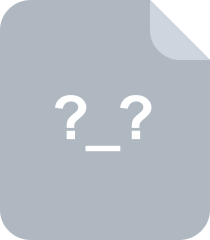
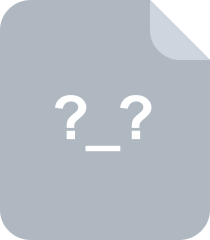
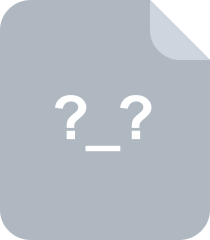
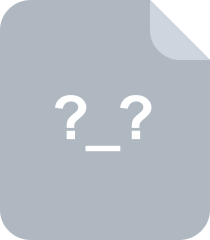
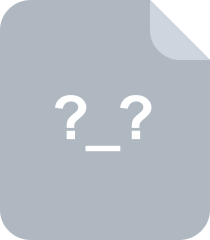
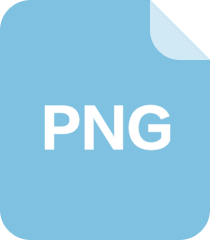
共 12 条
- 1
资源评论
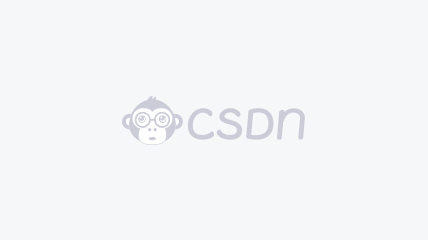
- 一只没有情感的机器猪2021-11-11请问怎么训练??没有跑的教程吗
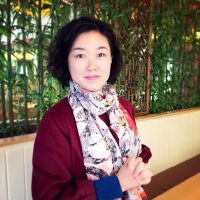
乘风破浪的海伦
- 粉丝: 28
- 资源: 4546
上传资源 快速赚钱
我的内容管理 展开
我的资源 快来上传第一个资源
我的收益
登录查看自己的收益我的积分 登录查看自己的积分
我的C币 登录后查看C币余额
我的收藏
我的下载
下载帮助

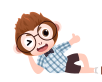
最新资源
- 基于Python的在线学习与推荐系统设计与实现(论文+源码)-kaic
- 蓝桥杯2024年第十五届省赛真题-前缀总分
- com.qihoo.appstore_300101305-1.apk
- tensorflow-gpu-2.7.1-cp37-cp37m-manylinux2010-x86-64.whl
- tensorflow-2.7.2-cp37-cp37m-manylinux2010-x86-64.whl
- tensorflow-2.7.1-cp39-cp39-manylinux2010-x86-64.whl
- 蓝桥杯2024年第十五届省赛真题-传送阵
- com.qihoo.appstore_300101305.apk
- linux之线程同步一.doc
- keil5配色方案10种
资源上传下载、课程学习等过程中有任何疑问或建议,欢迎提出宝贵意见哦~我们会及时处理!
点击此处反馈


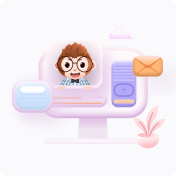
安全验证
文档复制为VIP权益,开通VIP直接复制
