# COVID-19 Document Classification
This repo provides a platform for testing document classification models on COVID-19 Literature.
It is an extension of the [Hedwig](https://github.com/castorini/hedwig) library and contains all necessary code to reproduce the results of some document classification models on a COVID-19 dataset created from the [LitCovid](https://www.ncbi.nlm.nih.gov/research/coronavirus/) collection. More information about the models tested and experiments carried out can be found [here]().
The Hedwig library was modified to work with a newer version of PyTorch and the Transformers library in order to import custom models. It was also extended to adapt [DocBERT](https://arxiv.org/abs/1904.08398) to use the Longformer model.
## Data
### LitCovid Data
The LitCovid document classification dataset found under the following directory can be used to reproduce the results found in the paper.
```
hedwig-data/datasets/LitCovid/
```
You can find a version compatible with the Hedwig library `train.tsv` and a raw version `LitCovid.train.csv` which includes PMIDs for each article.
We have also included a script to download the most up to date version of the LitCovid dataset by running the following commands:
```bash
cd scripts
bash load_litcovid.sh
```
This script will download, process and save both the processed and raw versions into the `data/FullLitCovid` directory. Please move the files to `hedwig-data/datasets/LitCovid` for further training.
This new data will not maintain the train, dev, test split found in the paper.
### Word Embeddings
Along with the dataset, you must download the word2vec embeddings for the traditional deep learning models.
Follow these instructions from the Hedwig repo to download the embeddings. The only files needed for our purposes should be under:
```
hedwig-data/embeddings
```
Option 1. Our [Wasabi](https://wasabi.com/)-hosted mirror:
```bash
$ wget http://nlp.rocks/hedwig -O hedwig-data.zip
$ unzip hedwig-data.zip
```
Option 2. Our school-hosted repository, [`hedwig-data`](https://git.uwaterloo.ca/jimmylin/hedwig-data):
```bash
$ git clone https://github.com/castorini/hedwig.git
$ git clone https://git.uwaterloo.ca/jimmylin/hedwig-data.git
```
Next, organize your directory structure as follows:
```
.
├── hedwig
└── hedwig-data
```
After cloning the hedwig-data repo, you need to unzip the embeddings and run the preprocessing script:
```bash
cd hedwig-data/embeddings/word2vec
tar -xvzf GoogleNews-vectors-negative300.tgz
```
### Pre-Trained Longformer
Finally, you'll need the Longformer Pre-trained model.
Run the following commands to download the pre-trained Longformer from their [original repo](https://github.com/allenai/longformer).
```bash
cd hedwig
wget https://ai2-s2-research.s3-us-west-2.amazonaws.com/longformer/longformer-base-4096.tar.gz
tar -xvzf longformer-base-4096.tar.gz
```
### CORD-19 Test Dataset
The full articles, PMIDs and labels for the CORD-19 test dataset can be found under `data/cord19_annotations.tsv`.
The Jupyter notebook `scripts/clean_cord19_dataset.ipynb` creates the Hedwig compatible version found in `hedwig-data/dataset/LitCovid/cord19_test.tsv`.
## Requirements
We'd recommend creating a custom pip virtual environment and install all requirements via pip:
```
$ pip install -r requirements.txt
```
Code depends on data from NLTK (e.g., stopwords) so you'll have to download them.
Run the Python interpreter and type the commands:
```python
>>> import nltk
>>> nltk.download()
```
## Model Training
Running the following commands in the hedwig directory to train the transformer based models and the conventional deep learning models respectively:
```bash
bash run_all_bert.sh
bash run_all_deep.sh
```
To reproduce the results for traditional machine learning models on the LitCovid dataset run the Jupyter notebook `scripts/traditional_models.ipynb
`.
To reproduce the results on the CORD-19 test dataset run the following:
```bash
bash run_baselines_cord19.sh
```

Hsmiau
- 粉丝: 984
- 资源: 4653
最新资源
- 线控转向失效下容错差动转向协同控制策略研究-面向四轮轮毂电机驱动电动汽车,线控转向失效下的容错差动转向与横摆力矩协同控制方法,线控转向失效下的容错差动转向控制 以四轮轮毂电机驱动智能电动汽车为研究对
- 基于SSM的物业管理系统(有报告)。Javaee项目。ssm项目。
- Springboot+vue的人事管理系统(有报告),Javaee项目,springboot vue前后端分离项目。
- 基于SSM的影视创作论坛(有报告)。Javaee项目。ssm项目。
- 基于FPGA的FSK实现:Verilog代码详解与仿真验证,附上板测试报告及高难度代码深度解析文档,基于FPGA的FSK实现详解:Verilog代码实践与仿真上板全流程,基于fpga的fsk实现,代码
- Linux环境下ffmpeg与SDL2驱动的视频播放器构建方法
- Springboot+vue的在线试题题库管理系统(有报告),Javaee项目,springboot vue前后端分离项目。
- 基于Springboot的会员制医疗预约服务管理信息系统(有报告)。Javaee项目,springboot项目。
- 《含光伏550kW 33节点系统PSCAD接线图及其对谐波含量低的影响分析:兼顾电动汽车充电桩负荷的研究》,含光伏接入的33节点系统PSCAD接线图解析:550kW容量下的谐波含量微小分析及其与双电动
- ssm+vue的公司人力资源管理系统(有报告)。Javaee项目,ssm vue前后端分离项目。
- 基于SSM的老年公寓信息管理(有报告)。Javaee项目
- 基于SSM的文化线上体验馆(有报告)。Javaee项目。ssm项目。
- ssm+vue的OA办公系统(有报告)。Javaee项目,ssm vue前后端分离项目。
- 11.2版本SLM模拟教程:利用Flow3D软件进行高能量密度下匙孔孔隙的数值模拟与计算流体动力学分析,Flow3D模拟优化:11.2版本SLM增材制造数值模拟教程-模拟高能量密度下选区激光熔化匙孔
- 基于SSM的高校疫情防控出入信息管理系统(有报告)。Javaee项目。
- Springboot+vue的高校毕业与学位资格审核系统。Javaee项目,springboot vue前后端分离项目。
资源上传下载、课程学习等过程中有任何疑问或建议,欢迎提出宝贵意见哦~我们会及时处理!
点击此处反馈


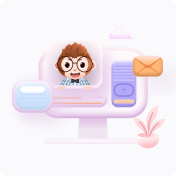
评论0