genetic-programming
===================
[Symbolic regression](http://en.wikipedia.org/wiki/Symbolic_regression) solver, based on [genetic programming](http://en.wikipedia.org/wiki/Genetic_programming) methodology.
### Table of contents
1. [Description](#description) <br/>
1.1 [Crossover](#crossover) <br/>
1.2 [Mutation](#mutation) <br/>
1.3 [Optimization of coefficients](#optimization-of-coefficients) <br/>
1.4 [Reducing complexity of syntax trees](#reducing-complexity-of-syntax-trees) <br/>
2. [Demo](#demo) <br/>
2.1 [f(x,y,z) - ?](#fxyz---) <br/>
3. [Quick start](#quick-start) <br/>
3.1 [Just download jar](#just-download-jar) <br/>
3.2 [Try it with Maven](#try-it-with-maven) <br/>
3.3 [Hello world](#hello-world)
# Description #
Each mathematical expression can be represented in form of syntax tree: <br/>
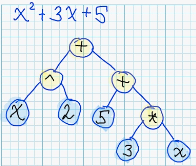
Actually, it worth to keep in mind, that there exists infinite number of different syntax trees, which corresponds to semantically equivalent expressions. For example: <br/>
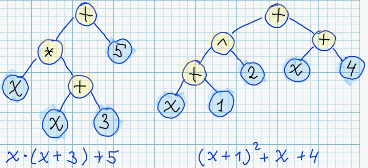
In practice, on of the most generic problems - is reconstruction of original function, having the information about its values in some specific points.
It is possible to apply [genetic algorithm](http://en.wikipedia.org/wiki/Genetic_algorithm) - for solving of given problem:
1. In terms of Genetic Algorithm - each syntax tree can be treated as a "chromosome" (an entity, which can "mutate" and change by "crossover" with other "chromosome")
2. It is needed to define [fitness function](http://en.wikipedia.org/wiki/Fitness_function): the function, which will calculate, how good each formula (which was encoded by syntax tree) - can represent existing data (e.g.: using [mean squared error](http://en.wikipedia.org/wiki/Mean_squared_error) value).
### Crossover ###
During "crossover" - syntax tree is modified by substituion of its subtree, with some subtree from other syntax tree.
Following image explains implementation of "crossover" operation over syntax trees: <br/>
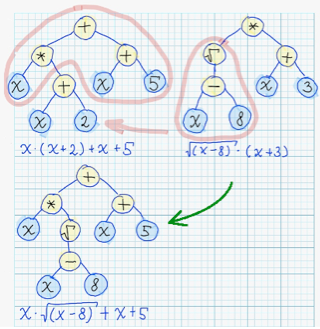
### Mutation ###
Currently implemented following "mutation" operations:
1. Substituion of some node of syntax tree - with node, which corresponds to different arithmetical operation: <br/>
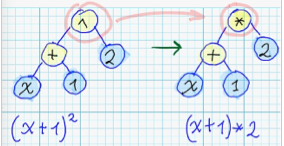
2. Substituion of some subtree with randomly generated subtree: <br/>
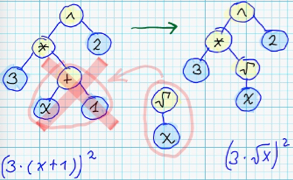
3. Removing of some intermediate node from syntax tree: <br/>
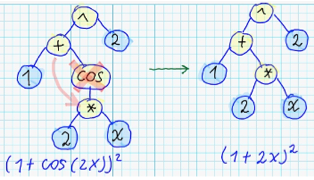
4. Expanding tree from root: <br/>
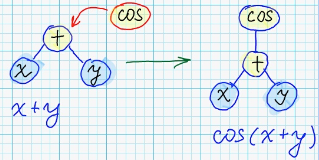
5. Swapping subtrees for non-commutative oparations: <br/>
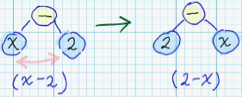
### Optimization of coefficients ###
Actually, some syntax tree might represent correct structure of searchable function, but due to some non-optimal values of coefficients - given syntax tree can be considered as non-optimal by fitness function.
For example, following image displays target values of searchable function (red crosses) - and two functions-candidates (green and blue): <br/>
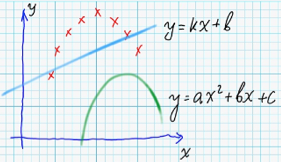
Blue line has smaller value of mean squared error, but, actually - green parabola - would be a better candidate for the final solution.
By this reason, current implementation of Symbolic Regression Solver - uses second pass of Genetic Algorithm - for optimizing of coefficients of each syntax tree. On the picture below - represented the way, how coefficients of each syntax tree - could be transformed to "chromosome": <br/>
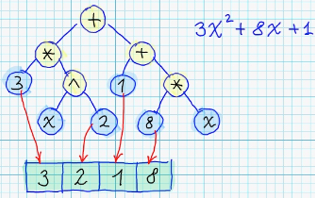
### Reducing complexity of syntax trees ###
1. Simplification of subtrees, which contains only constant-valued: <br/>
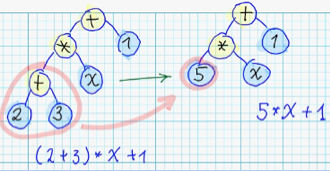
2. Pruning trees, which has height larger then threshold: <br/>
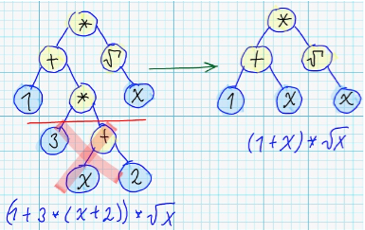
# Demo #
## f(x,y,z) - ? ##
Lets try to reconstruct original function, by following target values:
x | y | z | f(x,y,z)
---- | ---- | ---- | -------
26 | 35 | 1 | 830
8 | 24 | -11 | 130
20 | 1 | 10 | 477
33 | 11 | 2 | 1217
37 | 16 | 7 | 1524
Lets launch console application for "evolving" of **f(x,y,z)**:
1. Download http://github.com/lagodiuk/genetic-programming/tree/master/bin/symbolic_regression_1.0.jar
2. Create file **xyz.txt** with following content:
```txt
# allowed functions are: ADD SUB MUL DIV SQRT POW LN SIN COS
# set which functions to use:
ADD MUL SUB
# looking for:
f(x, y, z) — ?
# define training set:
f(26, 35, 1) = 830
f(8, 24, -11) = 130
f(20, 1, 10) = 477
f(33, 11, 2) = 1217
f(37, 16, 7) = 1524
```
(this file can be downloaded from: https://github.com/lagodiuk/genetic-programming/blob/master/bin/xyz.txt)
3. Launch: `java -jar symbolic_regression_1.0.jar xyz.txt` - and observe output for each iteration (output will be in format: iteration numbre, value of mean squared error, and evolved formula).
Below presented picture, which shows dynamics of changes of mean squared error, according to the best "evolved" syntax trees (the axis "x" - represents number of iteration): <br/>
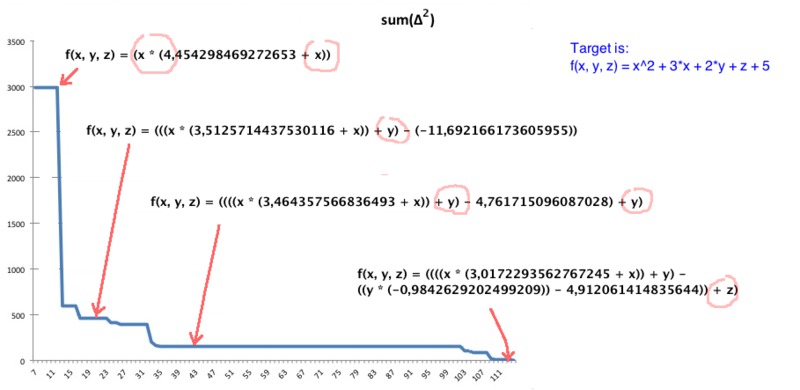
# Quick start #
## just download jar ##
The most simple way is download http://github.com/lagodiuk/genetic-programming/tree/master/bin/symbolic_regression_1.0.jar and add it to your classpath.
## try it with maven ##
This project depends on [Generic Genetic Algorithm project](https://github.com/lagodiuk/genetic-algorithm) (as a maven dependency).
<ol>
<li> git clone https://github.com/lagodiuk/genetic-algorithm.git </li>
<li> git clone https://github.com/lagodiuk/genetic-programming.git </li>
<li> mvn -f genetic-algorithm/pom.xml install </li>
<li> mvn -f genetic-programming/pom.xml install </li>
</ol>
Now you can add following maven dependencies to your project:
```xml
<dependency>
<groupId>com.lagodiuk</groupId>
<artifactId>ga</artifactId>
<version>1.0.1</version>
</dependency>
<dependency>
<groupId>com.lagodiuk</groupId>
<artifactId>gp</artifactId>
<version>1.0</version>
</dependency>
```
### hello world ###
```java
import java.util.LinkedList;
import java.util.List;
import com.lagodiuk.gp.symbolic.SymbolicRegressionEngine;
import com.lagodiuk.gp.symbolic.SymbolicRegressionIterationListener;
import com.lagodiuk.gp.symbolic.TabulatedFunctionFitness;
import com.lagodiuk.gp.symbolic.Target;
import com.lagodiuk.gp.symbolic.interpreter.Expression;
import com.lagodiuk.gp.symbolic.interpreter.Functions;
/**
* f(x) - ? <br/>
*
* f(0) = 0 <br/>
* f(1) = 11 <br/>
* f(2) = 24 <br/>
* f(3) = 39 <br/>
* f(4) = 56 <br/>
* f(5) = 75 <br/>
* f(6) = 96 <br/>
*
* (target function is f(x) = x^2 + 10*x)
*/
public class HelloSymbolicRegression {
public static

GDMS
- 粉丝: 33
- 资源: 4529
最新资源
- 51单片机多路温度采集系统(二) C程序、proteus仿真、报告、仿真操作视频 实现对温度进行多路检测并准确显示 支持LCD1602循环显示当前8组温度值
- 四轮独立驱动电动汽车转矩分配控制 CarSim与Simulink联合 三自由度车辆模型(纵向、横向、横摆) 控制方法为离散LQR(包括连续系统的离散方法和求解方法) 带有完整详细的控制器、二自由度稳定
- MATLAB环境下一种基于模型的脉冲小波及其稀疏表示在轴承故障诊断中的应用 算法运行环境为MATLAB R2018A,将脉冲小波及其稀疏表示应用于轴承故障诊断 算法可迁移至金融时间序列,地震 微震
- MATLAB代码:电网-热网-气网的调度模型 目标函数:最小化火电发电成本、天然气源出力成本 电力系统中的机组包括传统燃煤机组、燃气机组以及CHP机组 负荷除了常规负荷外,还包括电锅炉 考虑39
- 基于滑膜控制的后轮主动(ARS)和DYC的协调稳定性控制,上层ARS产生期望后轮转角度,DYC产生横摆力矩Mz,下层采用基于附着系数和车速对附加横摆力矩进行分配,控制效果良好,能实现车辆在高低附着系数
- 多区温控程序,单区温控程序 温控仪表程序控制,MCGS通讯温控仪表控制温度升温工艺控制程序, 各种品牌PID仪表通讯触摸屏,30段温控程序,升温,恒温,降温,宇电控温工艺,岛电工艺程序,MCGS通讯
- 双闭环转速、电流直流调速系统的课程设计(MATLAB仿真) matlab simulink搭建的双闭环直流调速系统,电气模型,采用了ASR和ACR两个PI调节器,可以再保证系统稳定的条件下实现转速
- 智能软开关 主动配电网 优化运行 sop 规划 调度 配电网 重构 在电力系统运行中,智能软开关sop具有灵活地调节潮流和电压的能力 智能软开关sop是相较于传统联络开关提出的新的开关形式 智能软
- 多电压等级直流微店网母线电压控制研究 1、高频隔离DC DC变器模型(DAB-双有源全桥),基于MATLAB Simulink建模仿真 电压电流双闭环控制,功率双向流动,ZVS软开关 2、buck
- Modbus 主站 从站 在STM32单片机上的实现,企业在用的程序
- MATLAB代码:多源动态最优潮流的分布鲁棒优化方法 关键词:鲁棒优化;最优潮流;数据驱动;多源电力系统;不确定性 参考文档:《多源动态最优潮流的分布鲁棒优化方法》 仿真平台:MATLAB YALM
- 威纶通触摸屏与4台台达变频器485通讯,不经过pLc,有启动,停止,正转,反转频率输出,频率设定,电流输出,电压输出,DC-bus电压 马达转速
- 威纶通触摸屏与台达变频器485通讯,不经过PLC,有启动,停止,正转,反转频率输出,频率设定,电流输出,电压输出, 马达转速,运行状态
- MATLAB仿真-基于下垂控制的离网仿真 可观察负载突增下频率变化以及频率变化率 主电路为三相逆变器、LC滤波器、功率负载 控制方法为下垂控制 附带原理lunwen
- 默纳克系统升级工具烧录程序软件升级工具v3.14 v3.16 老国标烧录软件V1.26 Bootloader烧录工具V2.41 V3.10 一共5个烧录程序,软件升级
- 三菱FX3U PLC,三轴搬运程序,程序结构清晰 通俗易懂,注释齐全,控制三个台达B2伺服,信捷触摸屏程序,有电气CAD图纸
资源上传下载、课程学习等过程中有任何疑问或建议,欢迎提出宝贵意见哦~我们会及时处理!
点击此处反馈


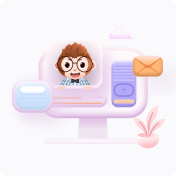
评论0