## Spatial CNN Prototxt Generator
This script is for generating [Spatial CNN](https://github.com/XingangPan/SCNN) caffe prototxt file.
### How to use
- Run run.sh to generate Spatial CNN module, modify parameters to yours.
'--height', '--width', and '--channel' are number of rows, columns, and channels of the last feature maps respectively.
- Copy the text in the generated SCNN.prototxt, and paste it to the right position in your original model prototxt file (the one that you want to add Spatial CNN).
- Don't forget to modify the 'bottom' of the next layer to "SCNN".
- Example:
Original prototxt file: examples/resnet101.prototxt
Generated SCNN text: examples/SCNN.prototxt
New prototxt file with SCNN: examples/resnet101_SCNN.prototxt
(For this example, the prototxt is aligned with the caffe version at https://github.com/hszhao/PSPNet)
You can visualize the network architecture at http://ethereon.github.io/netscope/#/editor.
### About Spatial CNN (SCNN)

- Spatial CNN enables explicit and effective spatial information propagation between neurons in the same layer of a CNN.
- For more details, please refer to [our paper](https://arxiv.org/abs/1712.06080).
### Practical Concerns
- In practice I initialize SCNN layers with variance sqrt(5) times smaller than the MSRA initialization for numarical stability concern.
- It would be helpful to initialize all other layers with a pretrained model. Directly training from random initialization may cause SCNN to diverge.
- The best way to utilize SCNN is to insert it right after the last feature maps (top hidden layer, before the classification layer).
- The number of channels (num_c) of the last feature maps should not be too large since the memory consumption of SCNN is sensitive to num_c.
In practive I find 128 to be an appropriate choice for num_c, 512 might be a little bit large. You can try larger num_c if you have enough GPU memory.
- To reduce num_c in ResNet50/101, directly change num_output from 512 to 128 in "Conv5_4" layer may decrease the performance. Aternatively, you can add an extra "Conv5_5" layer to map the 512 feature maps to 128 with 1x1 convolution, as did in 'examples/resnet101.prototxt'.

还是那个小宇
- 粉丝: 34
- 资源: 4729
最新资源
- 使用扩频技术的BPSK接收机Matlab仿真.rar
- 使用高阶统计量的STBC盲信道估计Matlab代码.rar
- 使用汉明(7, 4)块码的信道编码Matlab代码.rar
- 使用了BPSK调制并展示了使用OFDM时BER的降低Matlab实现.rar
- 使用雷尔衰落信道和加性高斯白噪声的MC CDMA系统的PAPR(峰值平均功率比)Matlab代码.rar
- 使用连续波的超宽带接收器_发射器Matlab代码.rar
- 使用蒙特卡洛模拟对BPSK传输在噪声环境下的仿真误码率Matlab代码.rar
- 使用蒙特卡洛模拟对噪声中QPSK传输的仿真误码率Matlab代码.rar
- 使用欧几里得距离最小化的QAM检测方法Matlab代码.rar
- 使用限幅和 SLM 技术表示具有 PAPR 降低功能的 OFDM 信号matlab代码.rar
- 使用预测特征值方法对源数进行盲检测Matlab代码.rar
- 使用奇偶校验矩阵对二进制消息进行编码Matlab代码.rar
- 使用预测性Viterbi算法在有色噪声中检测符号的最大似然检测Matlab代码.rar
- 使用正交相移键控(QPSK)4-QAM进行传输。使用最大似然检测方案计算模拟的误码率(BER)Matlab代码.rar
- 使用最小二乘法和最小均方误差方法的信道估计的OFDM系统Matlab代码.rar
- 使用最小均方(LMS)算法在频域进行信道估计Matlab代码.rar
资源上传下载、课程学习等过程中有任何疑问或建议,欢迎提出宝贵意见哦~我们会及时处理!
点击此处反馈


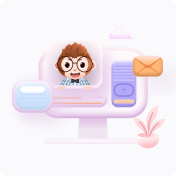
评论0