# EfficientNet PyTorch
### Quickstart
Install with `pip install efficientnet_pytorch` and load a pretrained EfficientNet with:
```python
from efficientnet_pytorch import EfficientNet
model = EfficientNet.from_pretrained('efficientnet-b0')
```
### Updates
#### Update (Aug 25, 2020)
This update adds:
* A new `include_top` (default: `True`) option ([#208](https://github.com/lukemelas/EfficientNet-PyTorch/pull/208))
* Continuous testing with [sotabench](https://sotabench.com/)
* Code quality improvements and fixes ([#215](https://github.com/lukemelas/EfficientNet-PyTorch/pull/215) [#223](https://github.com/lukemelas/EfficientNet-PyTorch/pull/223))
#### Update (May 14, 2020)
This update adds comprehensive comments and documentation (thanks to @workingcoder).
#### Update (January 23, 2020)
This update adds a new category of pre-trained model based on adversarial training, called _advprop_. It is important to note that the preprocessing required for the advprop pretrained models is slightly different from normal ImageNet preprocessing. As a result, by default, advprop models are not used. To load a model with advprop, use:
```python
model = EfficientNet.from_pretrained("efficientnet-b0", advprop=True)
```
There is also a new, large `efficientnet-b8` pretrained model that is only available in advprop form. When using these models, replace ImageNet preprocessing code as follows:
```python
if advprop: # for models using advprop pretrained weights
normalize = transforms.Lambda(lambda img: img * 2.0 - 1.0)
else:
normalize = transforms.Normalize(mean=[0.485, 0.456, 0.406],
std=[0.229, 0.224, 0.225])
```
This update also addresses multiple other issues ([#115](https://github.com/lukemelas/EfficientNet-PyTorch/issues/115), [#128](https://github.com/lukemelas/EfficientNet-PyTorch/issues/128)).
#### Update (October 15, 2019)
This update allows you to choose whether to use a memory-efficient Swish activation. The memory-efficient version is chosen by default, but it cannot be used when exporting using PyTorch JIT. For this purpose, we have also included a standard (export-friendly) swish activation function. To switch to the export-friendly version, simply call `model.set_swish(memory_efficient=False)` after loading your desired model. This update addresses issues [#88](https://github.com/lukemelas/EfficientNet-PyTorch/pull/88) and [#89](https://github.com/lukemelas/EfficientNet-PyTorch/pull/89).
#### Update (October 12, 2019)
This update makes the Swish activation function more memory-efficient. It also addresses pull requests [#72](https://github.com/lukemelas/EfficientNet-PyTorch/pull/72), [#73](https://github.com/lukemelas/EfficientNet-PyTorch/pull/73), [#85](https://github.com/lukemelas/EfficientNet-PyTorch/pull/85), and [#86](https://github.com/lukemelas/EfficientNet-PyTorch/pull/86). Thanks to the authors of all the pull requests!
#### Update (July 31, 2019)
_Upgrade the pip package with_ `pip install --upgrade efficientnet-pytorch`
The B6 and B7 models are now available. Additionally, _all_ pretrained models have been updated to use AutoAugment preprocessing, which translates to better performance across the board. Usage is the same as before:
```python
from efficientnet_pytorch import EfficientNet
model = EfficientNet.from_pretrained('efficientnet-b7')
```
#### Update (June 29, 2019)
This update adds easy model exporting ([#20](https://github.com/lukemelas/EfficientNet-PyTorch/issues/20)) and feature extraction ([#38](https://github.com/lukemelas/EfficientNet-PyTorch/issues/38)).
* [Example: Export to ONNX](#example-export)
* [Example: Extract features](#example-feature-extraction)
* Also: fixed a CUDA/CPU bug ([#32](https://github.com/lukemelas/EfficientNet-PyTorch/issues/32))
It is also now incredibly simple to load a pretrained model with a new number of classes for transfer learning:
```python
model = EfficientNet.from_pretrained('efficientnet-b1', num_classes=23)
```
#### Update (June 23, 2019)
The B4 and B5 models are now available. Their usage is identical to the other models:
```python
from efficientnet_pytorch import EfficientNet
model = EfficientNet.from_pretrained('efficientnet-b4')
```
### Overview
This repository contains an op-for-op PyTorch reimplementation of [EfficientNet](https://arxiv.org/abs/1905.11946), along with pre-trained models and examples.
The goal of this implementation is to be simple, highly extensible, and easy to integrate into your own projects. This implementation is a work in progress -- new features are currently being implemented.
At the moment, you can easily:
* Load pretrained EfficientNet models
* Use EfficientNet models for classification or feature extraction
* Evaluate EfficientNet models on ImageNet or your own images
_Upcoming features_: In the next few days, you will be able to:
* Train new models from scratch on ImageNet with a simple command
* Quickly finetune an EfficientNet on your own dataset
* Export EfficientNet models for production
### Table of contents
1. [About EfficientNet](#about-efficientnet)
2. [About EfficientNet-PyTorch](#about-efficientnet-pytorch)
3. [Installation](#installation)
4. [Usage](#usage)
* [Load pretrained models](#loading-pretrained-models)
* [Example: Classify](#example-classification)
* [Example: Extract features](#example-feature-extraction)
* [Example: Export to ONNX](#example-export)
6. [Contributing](#contributing)
### About EfficientNet
If you're new to EfficientNets, here is an explanation straight from the official TensorFlow implementation:
EfficientNets are a family of image classification models, which achieve state-of-the-art accuracy, yet being an order-of-magnitude smaller and faster than previous models. We develop EfficientNets based on AutoML and Compound Scaling. In particular, we first use [AutoML Mobile framework](https://ai.googleblog.com/2018/08/mnasnet-towards-automating-design-of.html) to develop a mobile-size baseline network, named as EfficientNet-B0; Then, we use the compound scaling method to scale up this baseline to obtain EfficientNet-B1 to B7.
<table border="0">
<tr>
<td>
<img src="https://raw.githubusercontent.com/tensorflow/tpu/master/models/official/efficientnet/g3doc/params.png" width="100%" />
</td>
<td>
<img src="https://raw.githubusercontent.com/tensorflow/tpu/master/models/official/efficientnet/g3doc/flops.png", width="90%" />
</td>
</tr>
</table>
EfficientNets achieve state-of-the-art accuracy on ImageNet with an order of magnitude better efficiency:
* In high-accuracy regime, our EfficientNet-B7 achieves state-of-the-art 84.4% top-1 / 97.1% top-5 accuracy on ImageNet with 66M parameters and 37B FLOPS, being 8.4x smaller and 6.1x faster on CPU inference than previous best [Gpipe](https://arxiv.org/abs/1811.06965).
* In middle-accuracy regime, our EfficientNet-B1 is 7.6x smaller and 5.7x faster on CPU inference than [ResNet-152](https://arxiv.org/abs/1512.03385), with similar ImageNet accuracy.
* Compared with the widely used [ResNet-50](https://arxiv.org/abs/1512.03385), our EfficientNet-B4 improves the top-1 accuracy from 76.3% of ResNet-50 to 82.6% (+6.3%), under similar FLOPS constraint.
### About EfficientNet PyTorch
EfficientNet PyTorch is a PyTorch re-implementation of EfficientNet. It is consistent with the [original TensorFlow implementation](https://github.com/tensorflow/tpu/tree/master/models/official/efficientnet), such that it is easy to load weights from a TensorFlow checkpoint. At the same time, we aim to make our PyTorch implementation as simple, flexible, and extensible as possible.
If you have any feature requests or questions, feel free to leave them as GitHub issues!
### Installation
Install via pip:
```bash
pip install efficientnet_pytorch
```
Or install from source:
```bash
git clone https://github.com/lukemelas/EfficientNet-PyTorch
cd EfficientNet-Pytorch
pip install -e .
```
##
没有合适的资源?快使用搜索试试~ 我知道了~
EfficientNet-PyTorch:EfficientNet的PyTorch实现
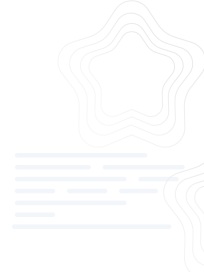
共33个文件
py:17个
sh:5个
md:4个


温馨提示
EfficientNet PyTorch 快速开始 使用pip install efficientnet_pytorch的net_pytorch并使用以下命令加载经过预训练的EfficientNet: from efficientnet_pytorch import EfficientNet model = EfficientNet . from_pretrained ( 'efficientnet-b0' ) 更新 更新(2020年8月25日) 此更新添加: 一个新的include_top (默认: True )选项( ) 使用连续测试 代码质量改进和修复( ) 更新(2020年5月14日) 此更新添加了全面的注释和文档(感谢@workingcoder)。 更新(2020年1月23日) 此更新基于对抗训练添加了新类别的预训练模型,称为advprop 。 重要的是要注意,
资源详情
资源评论
资源推荐
收起资源包目录


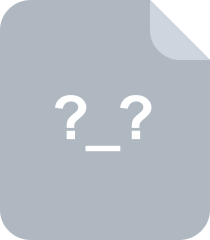
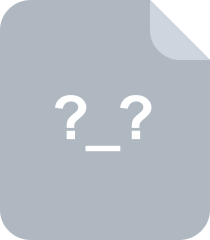
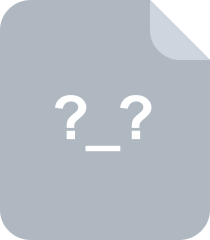
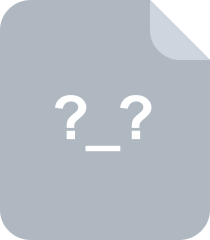



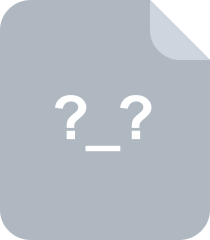
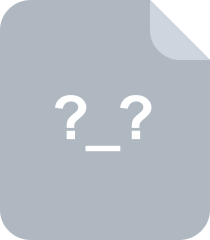
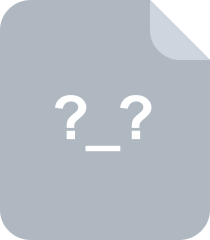

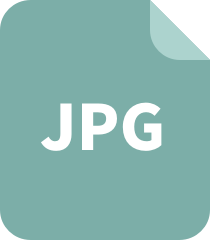
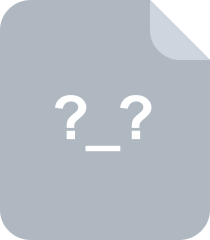
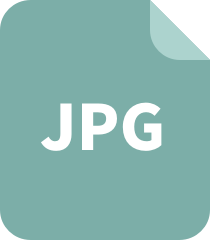
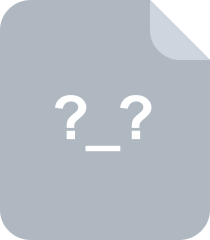
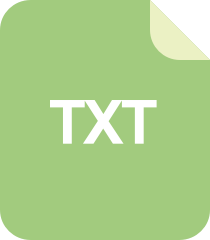
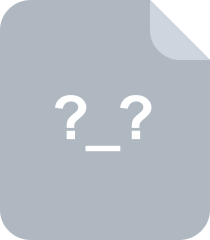

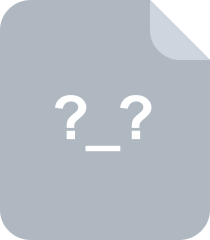


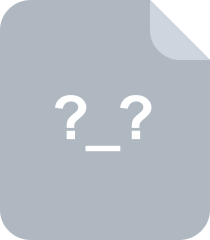

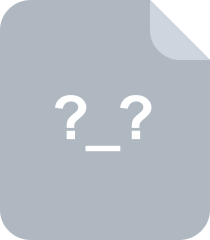
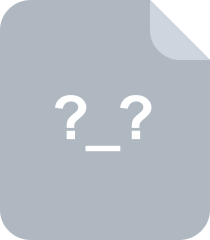
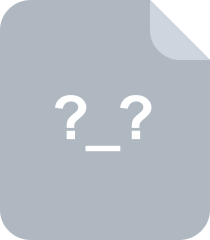
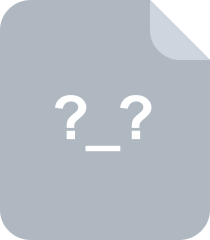
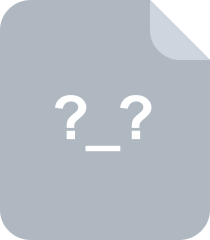
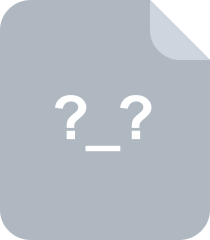
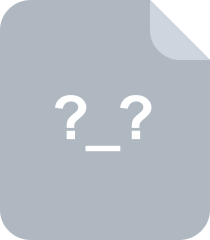
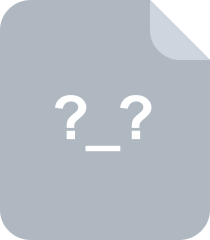
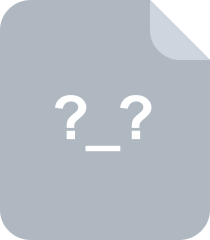
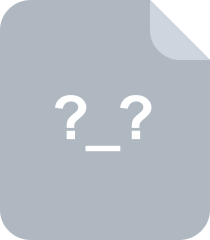
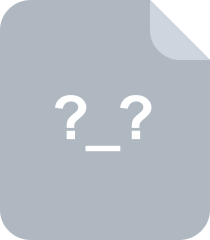

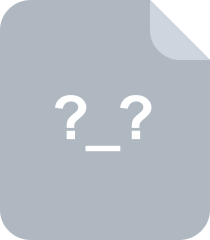
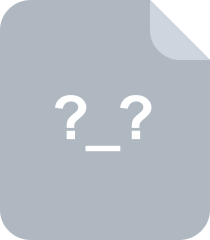
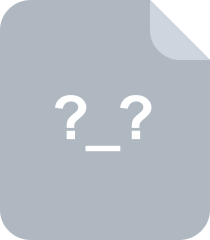
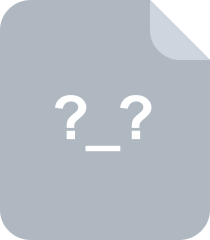

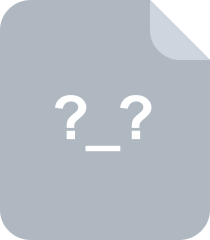
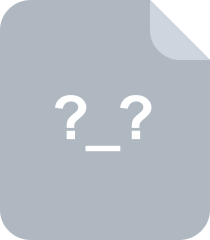
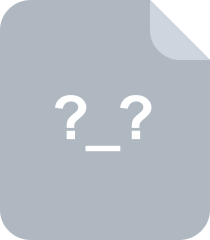
共 33 条
- 1
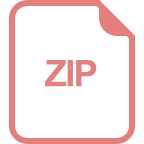
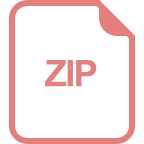
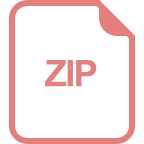
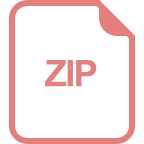
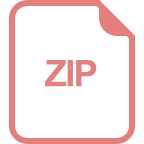
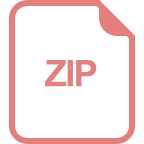
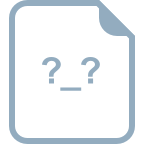
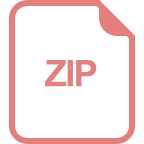
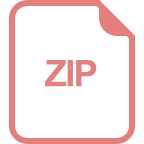
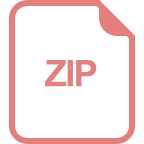
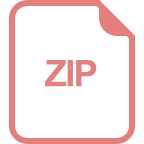
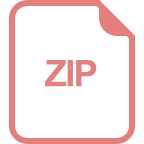
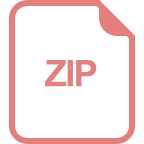
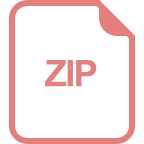
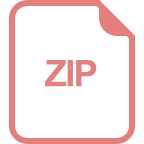
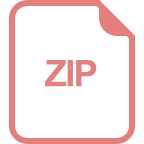
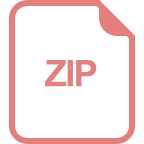
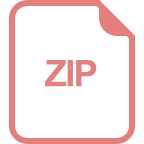
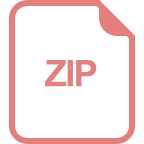
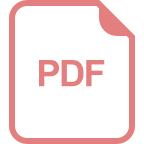

泰国旅行
- 粉丝: 37
- 资源: 4773
上传资源 快速赚钱
我的内容管理 展开
我的资源 快来上传第一个资源
我的收益
登录查看自己的收益我的积分 登录查看自己的积分
我的C币 登录后查看C币余额
我的收藏
我的下载
下载帮助

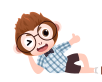
最新资源
- 【年度培训】培训效果评估报告(修改版)行政人事CLUB.doc
- 【年度培训】培训计划表行政人事CLUB.doc
- 【年度培训】公司员工培训总结报告行政人事CLUB.doc
- 【年度培训】年度培训计划及预算方案行政人事CLUB.doc
- 【年度培训】年度培训总结模板(内附表格,拿来即用)行政人事CLUB.doc
- 【年度培训】2023年度公司培训计划方案行政人事CLUB.doc
- 【年度培训】公司年度培训工作计划(完整版)行政人事CLUB.doc
- 【年度培训】【企业培训师】年度培训工作总结报告行政人事CLUB.doc
- 【年度培训】培训需求调查行政人事CLUB.pptx
- 【年度培训】DP152企业培训体系建设需求分析PPT行政人事CLUB.pptx
- 【年度培训】人力资源员工培训管理系统(完美版)行政人事CLUB.xlsx
- 【年度培训】2023年全年培训计划的副本行政人事CLUB.pptx
- 探索大规模语言模型在上下文学习中的决策边界机制
- 【年度培训】培训需求分析及评估行政人事CLUB.ppt
- 【年度培训】培训效果评估问卷行政人事CLUB.xlsx
- 【年度培训】培训员工跟踪表行政人事CLUB.xlsx
资源上传下载、课程学习等过程中有任何疑问或建议,欢迎提出宝贵意见哦~我们会及时处理!
点击此处反馈


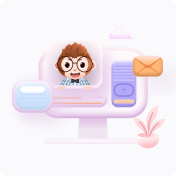
安全验证
文档复制为VIP权益,开通VIP直接复制

评论1