# WaveNet vocoder
[](https://pypi.python.org/pypi/wavenet_vocoder)
[](https://travis-ci.org/r9y9/wavenet_vocoder)
[](https://ci.appveyor.com/project/r9y9/wavenet-vocoder)
The goal of the repository is to provide an implementation of the WaveNet vocoder, which can generate high quality raw speech samples conditioned on linguistic or acoustic features.
Audio samples are available at https://r9y9.github.io/wavenet_vocoder/.
## Online TTS demo
A notebook supposed to be executed on https://colab.research.google.com is available:
- [Tacotron2: WaveNet-based text-to-speech demo](https://colab.research.google.com/github/r9y9/Colaboratory/blob/master/Tacotron2_and_WaveNet_text_to_speech_demo.ipynb)
## Highlights
- Focus on local and global conditioning of WaveNet, which is essential for vocoder.
- Mixture of logistic distributions loss / sampling
- Various audio samples and pre-trained models
- Fast inference by caching intermediate states in convolutions. Similar to [arXiv:1611.09482](https://arxiv.org/abs/1611.09482)
## Pre-trained models
**Note**: This is not itself a text-to-speech (TTS) model. With a pre-trained model provided here, you can synthesize waveform given a *mel spectrogram*, not raw text. You will need mel-spectrogram prediction model (such as Tacotron2) to use the pre-trained models for TTS.
| Model URL | Data | Hyper params URL | Git commit | Steps |
|----------------------------------------------------------------------------------------------------------------------------------|------------|------------------------------------------------------------------------------------------------------|----------------------------------------------------------------------------------------------------|---------------|
| [link](https://www.dropbox.com/s/zdbfprugbagfp2w/20180510_mixture_lj_checkpoint_step000320000_ema.pth?dl=0) | LJSpeech | [link](https://www.dropbox.com/s/0vsd7973w20eskz/20180510_mixture_lj_checkpoint_step000320000_ema.json?dl=0) | [2092a64](https://github.com/r9y9/wavenet_vocoder/commit/2092a647e60ce002389818de1fa66d0a2c5763d8) | 1000k~ steps |
| [link](https://www.dropbox.com/s/d0qk4ow9uuh2lww/20180212_mixture_multispeaker_cmu_arctic_checkpoint_step000740000_ema.pth?dl=0) | CMU ARCTIC | [link](https://www.dropbox.com/s/i35yigj5hvmeol8/20180212_multispeaker_cmu_arctic_mixture.json?dl=0) | [b1a1076](https://github.com/r9y9/wavenet_vocoder/tree/b1a1076e8b5d9b3e275c28f2f7f4d7cd0e75dae4) | 740k steps |
To use pre-trained models, first checkout the specific git commit noted above. i.e.,
```
git checkout ${commit_hash}
```
And then follows "Synthesize from a checkpoint" section in the README. Note that old version of synthesis.py may not accept `--preset=<json>` parameter and you might have to change `hparams.py` according to the preset (json) file.
You could try for example:
```
# Assuming you have downloaded LJSpeech-1.1 at ~/data/LJSpeech-1.1
# pretrained model (20180510_mixture_lj_checkpoint_step000320000_ema.pth)
# hparams (20180510_mixture_lj_checkpoint_step000320000_ema.json)
git checkout 2092a64
python preprocess.py ljspeech ~/data/LJSpeech-1.1 ./data/ljspeech \
--preset=20180510_mixture_lj_checkpoint_step000320000_ema.json
python synthesis.py --preset=20180510_mixture_lj_checkpoint_step000320000_ema.json \
--conditional=./data/ljspeech/ljspeech-mel-00001.npy \
20180510_mixture_lj_checkpoint_step000320000_ema.pth \
generated
```
You can find a generated wav file in `generated` directory. Wonder how it works? then take a look at code:)
## Requirements
- Python 3
- CUDA >= 8.0
- PyTorch >= v0.4.0
- TensorFlow >= v1.3
## Installation
The repository contains a core library (PyTorch implementation of the WaveNet) and utility scripts. All the library and its dependencies can be installed by:
```
git clone https://github.com/r9y9/wavenet_vocoder && cd wavenet_vocoder
pip install -e ".[train]"
```
If you only need the library part, then you can install it by the following command:
```
pip install wavenet_vocoder
```
## Getting started
### Preset parameters
There are many hyper parameters to be turned depends on data. For typical datasets, parameters known to work good (**preset**) are provided in the repository. See `presets` directory for details. Notice that
1. `preprocess.py`
2. `train.py`
3. `synthesis.py`
accepts `--preset=<json>` *optional* parameter, which specifies where to load preset parameters. If you are going to use preset parameters, then you must use same `--preset=<json>` throughout preprocessing, training and evaluation. e.g.,
```
python preprocess.py --preset=presets/cmu_arctic_8bit.json cmu_arctic ~/data/cmu_arctic
python train.py --preset=presets/cmu_arctic_8bit.json --data-root=./data/cmu_arctic
```
instead of
```
python preprocess.py cmu_arctic ~/data/cmu_arctic
# warning! this may use different hyper parameters used at preprocessing stage
python train.py --preset=presets/cmu_arctic_8bit.json --data-root=./data/cmu_arctic
```
### 0. Download dataset
- CMU ARCTIC (en): http://festvox.org/cmu_arctic/
- LJSpeech (en): https://keithito.com/LJ-Speech-Dataset/
### 1. Preprocessing
Usage:
```
python preprocess.py ${dataset_name} ${dataset_path} ${out_dir} --preset=<json>
```
Supported `${dataset_name}`s for now are
- `cmu_arctic` (multi-speaker)
- `ljspeech` (single speaker)
Assuming you use preset parameters known to work good for CMU ARCTIC dataset and have data in `~/data/cmu_arctic`, then you can preprocess data by:
```
python preprocess.py cmu_arctic ~/data/cmu_arctic ./data/cmu_arctic --preset=presets/cmu_arctic_8bit.json
```
When this is done, you will see time-aligned extracted features (pairs of audio and mel-spectrogram) in `./data/cmu_arctic`.
### 2. Training
>Note: for multi gpu training, you have better ensure that batch_size % num_gpu == 0
Usage:
```
python train.py --data-root=${data-root} --preset=<json> --hparams="parameters you want to override"
```
Important options:
- `--speaker-id=<n>`: (Multi-speaker dataset only) it specifies which speaker of data we use for training. If this is not specified, all training data are used. This should only be specified when you are dealing with a multi-speaker dataset. For example, if you are trying to build a speaker-dependent WaveNet vocoder for speaker `awb` of CMU ARCTIC, then you have to specify `--speaker-id=0`. Speaker ID is automatically assigned as follows:
```py
In [1]: from nnmnkwii.datasets import cmu_arctic
In [2]: [(i, s) for (i,s) in enumerate(cmu_arctic.available_speakers)]
Out[2]:
[(0, 'awb'),
(1, 'bdl'),
(2, 'clb'),
(3, 'jmk'),
(4, 'ksp'),
(5, 'rms'),
(6, 'slt')]
```
#### Training un-conditional WaveNet
```
python train.py --data-root=./data/cmu_arctic/
--hparams="cin_channels=-1,gin_channels=-1"
```
You have to disable global and local conditioning by setting `gin_channels` and `cin_channels` to negative values.
#### Training WaveNet conditioned on mel-spectrogram
```
python train.py --data-root=./data/cmu_arctic/ --speaker-id=0 \
--hparams="cin_channels=80,gin_channels=-1"
```
#### Training WaveNet conditioned on mel-spectrogram and speaker embedding
```
python train.py --data-root=./data/cmu_arctic/ \
--hparams="cin_channels=80,gin_channels=16,n_speakers=7"
```
### 3. Monitor with Tensorboard
Logs are dumped in `./log` directory by default. You can monitor logs by tensorboard:
```
tensorboard --logdir=lo

狛绝的追随者
- 粉丝: 27
- 资源: 4611
最新资源
- Python中的图像处理.zip
- Python中的一个简单区块链.zip
- Python中的网络分析.zip
- Python中的一个小词云生成器.zip
- springboot189基于SpringBoot电商平台的设计与实现.zip
- springboot189基于SpringBoot电商平台的设计与实现.zip
- Python中的在线机器学习.zip
- springboot190基于springboot框架的工作流程管理系统的设计与实现.zip
- springboot190基于springboot框架的工作流程管理系统的设计与实现.zip
- Python中的张量和动态神经网络,具有强大的GPU加速.zip
- Python中的音频指纹和识别.zip
- Python中数据结构和算法的最小示例.zip
- Python中近似近邻库的基准测试.zip
- Python中一个强大的SpiderWeb爬虫系统.zip
- RE2是一个快速、安全、线程友好的正则表达式引擎替代方案,可以回溯PCRE、Perl和Python中使用的正则表达式引.zip
- Python资源大全中文版包括Web框架网络爬虫模板引擎数据库数据可视化图片处理等由开源前哨和Python开发者微信公.zip
资源上传下载、课程学习等过程中有任何疑问或建议,欢迎提出宝贵意见哦~我们会及时处理!
点击此处反馈


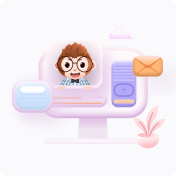
评论0