<p align="center">
<img src="demo/logo.png" width="200" height="100">
</p>
## LightTrack: A Generic Framework for Online Top-Down Human Pose Tracking
### Update 5/16/2019: Add Camera Demo
[[Project Page](http://guanghan.info/projects/LightTrack)] [[Paper](https://arxiv.org/pdf/1905.02822.pdf)] [[Github](http://github.com/Guanghan/lighttrack)]
[](https://paperswithcode.com/sota/pose-tracking-on-posetrack2017?p=lighttrack-a-generic-framework-for-online-top)
**With the provided code, you can easily:**
- **Perform online pose tracking on live webcam.**
- **Perform online pose tracking on arbitrary videos.**
- **Replicate ablation study experiments on PoseTrack'18 Validation Set.**
- **Train models on your own.**
- **Replace pose estimators or improve data association modules for future research.**
#### Real-life Application Scenarios:
- [Surveillance](https://youtu.be/P9Bzs3cSF-w) / [Sport analytics](https://youtu.be/PZVGYmr7Ryk) / Security / Self-driving / Selfie video / Short videos (Douyin, Tiktok, etc.)
## Table of Contents
- [LightTrack](#LightTrack)
* [Table of Contents](#table-of-contents)
* [Overview](#overview)
* [Prerequisites](#Prerequisites)
* [Getting Started](#getting-started)
* [Demo on Live Camera](#Demo-on-Live-Camera)
* [Demo on Arbitrary Videos](#Demo-on-Arbitrary-Videos)
* [Validate on PoseTrack 2018](#validate-on-posetrack-2018)
* [Evaluation on PoseTrack 2018](#evaluation-on-posetrack-2018)
* [Qualitative Results](#qualitative-results-on-posetrack)
* [Quantitative Results](#quantitative-results-on-posetrack)
* [Performance on PoseTrack 2017 Benchmark (Test Set)](#quantitative-results-on-posetrack)
* [Training](#training)
* [Pose Estimation Module](#1-pose-estimation-module)
* [Pose Matching Module: SGCN](#2-pose-matching-module)
* [Limitations](#limitations)
* [Citation](#citation)
* [Reference](#reference)
* [Contact](#contact)
## Overview
LightTrack is an effective light-weight framework for human pose tracking, truly online and generic for top-down pose tracking.
The code for [the paper](https://arxiv.org/pdf/1905.02822.pdf) includes LightTrack framework as well as its replaceable component modules, including detector, pose estimator and matcher, the code of which largely borrows or adapts from [Cascaded Pyramid Networks](https://github.com/chenyilun95/tf-cpn) [[1]], [PyTorch-YOLOv3](https://github.com/eriklindernoren/PyTorch-YOLOv3), [st-gcn](https://github.com/yysijie/st-gcn) and [OpenSVAI](https://doc-opensvai.readthedocs.io/en/latest/) [[3]].

In contrast to **Visual Object Tracking** (VOT) methods, in which the visual features are implicitly represented by kernels or CNN feature maps, we track each human pose by recursively updating the bounding box and its corresponding pose in an explicit manner. The bounding box region of a target is inferred from the explicit features, i.e., the human keypoints. Human keypoints can be considered as a series of special visual features.
The advantages of using pose as explicit features include:
* (1) The explicit features are human-related and interpretable, and have very strong and stable relationship with the bounding box position. Human pose enforces direct constraint on the bounding box region.
* (2) The task of pose estimation and tracking requires human keypoints be predicted in the first place. Taking advantage of the predicted keypoints is efficient in tracking the ROI region, which is almost free. This mechanism makes the online tracking possible.
* (3) It naturally keeps the identity of the candidates, which greatly alleviates the burden of data association in the system. Even when data association is necessary, we can re-use the pose features for skeleton-based pose matching.
(Here we adopt **Siamese Graph Convolutional Networks (SGCN)** for efficient identity association.)
**Single Pose Tracking** (SPT) and **Single Visual Object Tracking** (VOT) are thus incorporated into one unified functioning entity, easily implemented by a replaceable single-person human pose estimation module.
Below is a simple step-by-step explanation of how the LightTrack framework works.

(1). Detection only at the 1st Frame. Blue bboxes indicate tracklets inferred from keypoints.

(2). Detection at every other 10 Frames. Red bbox indicates keyframe detection.

(3). Detection at every other 10 Frames for multi-person:
* At non-keyframes, IDs are naturally kept for each person;
* At keyframes, IDs are associated via spatial consistency.
For more technical details, please refer to our arXiv paper.
## Prerequisites
- Set up a Python3 environment with provided anaconda environment file.
```Shell
# This anaconda environment should contain everything needed, including tensorflow, pytorch, etc.
conda env create -f environment.yml
```
### (Optional: set up the environment on your own)
- Install [PyTorch](http://pytorch.org/) 1.0.0 (or higher) and TorchVision. (Siamese Graph Convolutional Network)
- Install [Tensorflow](https://www.tensorflow.org/install) 1.12. Tensorflow v2.0 is not tested yet. (Human Pose Estimator)
- Install some other packages:
```Shell
pip install cython opencv-python pillow matplotlib
```
## Getting Started
- Clone this repository and enter the ~~dragon~~ lighttrack folder:
```Shell
git clone https://github.com/Guanghan/lighttrack.git;
# build some necessities
cd lighttrack/lib;
make;
cd ../graph/torchlight;
python setup.py install
# enter lighttrack
cd ../../
```
- If you'd like to train LightTrack, download the [COCO dataset](http://cocodataset.org/#download) and the [PoseTrack dataset](https://posetrack.net/users/download.php) first. Note that this script will take a while and dump 21gb of files into `./data/coco`. For PoseTrack dataset, you can replicate our ablation experiment results on the validation set. You will need to register at the official website and create entries in order to submit your test results to the server.
```Shell
sh data/download_coco.sh
sh data/download_posetrack17.sh
sh data/download_posetrack18.sh
```
### Demo on Live Camera
| PoseTracking Framework | Keyframe Detector | Keyframe ReID Module | Pose Estimator | FPS |
|:----------:|:-----------:|:--------------:|:----------------:|:---------:|
| LightTrack | YOLOv3 | Siamese GCN | MobileNetv1-Deconv | 220* / 15 |
- Download weights.
```Shell
cd weights;
bash ./download_weights.sh # download weights for backbones (only for training), detectors, pose estimators, pose matcher, etc.
cd -;
```
- Perform pose tracking demo on your Webcam.
```Shell
# access virtual environment
source activate py36;
# Perform LightTrack demo (on camera) with light-weight detector and pose estimator
python demo_camera_mobile.py
```
### Demo on Arbitrary Videos
| PoseTracking Framework | Keyframe Detector | Keyframe ReID Module | Pose Estimator | FPS |
|:----------:|:-----------:|:--------------:|:----------------:|:---------:|
| LightTrack | YOLOv3 | Siamese GCN | MobileNetv1-Deconv | 220* / 15 |
- Download demo video.
```Shell
cd data/demo;
bash ./download_demo_video.sh # download the video for demo; you could later replace it with your own video for fun
cd -;
```
- Perform online tracking demo.
```Shell
# access virtual environment
source activate py36;
# Perform LightTrack demo (on arbitrary video) with light-weight detector and pose estimator
python demo_video_mobile.py
```
- After processing, pose tracking results are stored in standardized OpenSVAI format JSON files, located at [**data/demo/jsons/**].
- Visualized images and videos h
没有合适的资源?快使用搜索试试~ 我知道了~
video-to-pose3D:一键将视频转换为3D姿势
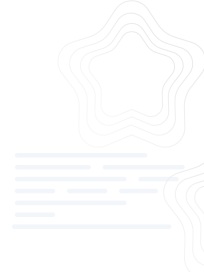
共483个文件
py:274个
md:19个
yaml:19个

需积分: 22 14 下载量 87 浏览量
2021-05-04
18:32:04
上传
评论 1
收藏 11.45MB ZIP 举报
温馨提示
视频转Pose3D 通过视频预测3d人体姿势 先决条件 环境 Linux系统 Python> 3.6发行版 依存关系 配套 火炬> 1.0.0 tqdm 枕头 科学的 大熊猫 h5py 视觉 尼巴贝 opencv-python(使用pip安装) matplotlib 2D联合探测器 字母(推荐) 从( | )下载duc_se.pth ,放置到./joints_detectors/Alphapose/models/sppe 从( | )下载yolov3-spp.weights ,放置到./joints_detectors/Alphapose/models/yolo HR-Net(我的测试环境中3d关节性能不佳) 从下载pose_hrnet * ),放置到./joints_detectors/hrnet/models/pytorch/pose_coco/ 从下载yo
资源详情
资源评论
资源推荐
收起资源包目录

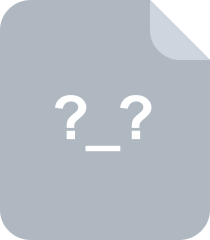
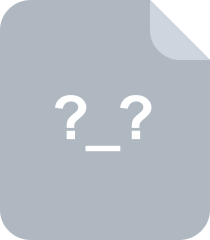
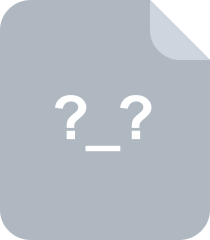
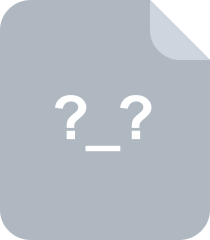
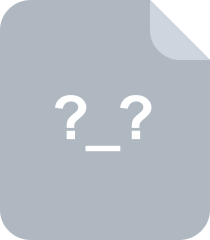
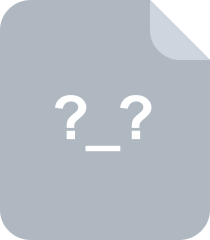
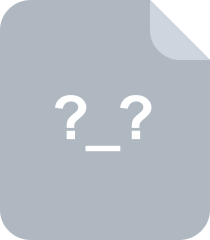
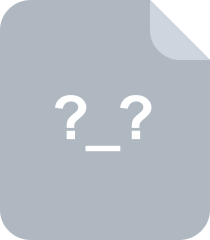
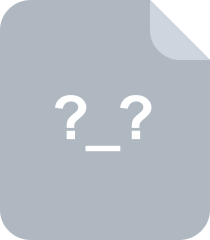
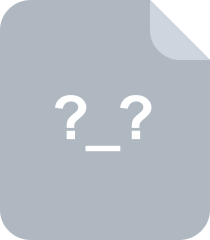
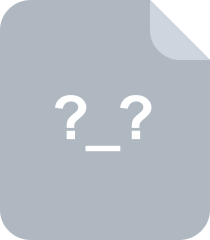
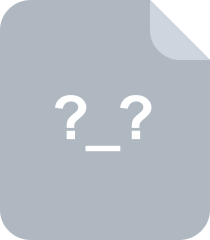
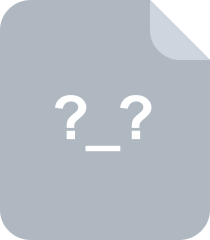
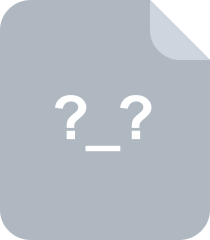
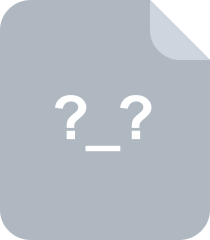
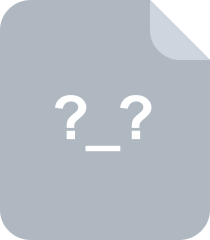
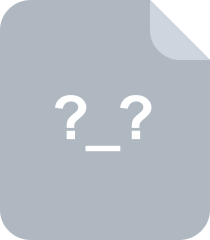
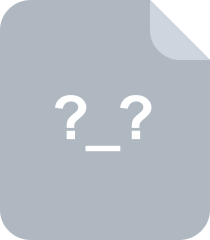
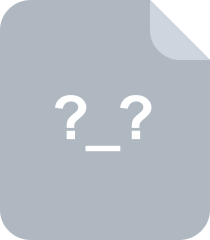
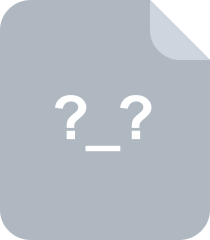
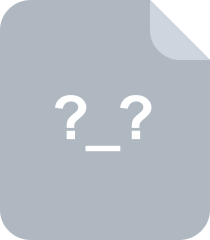
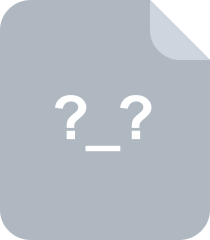
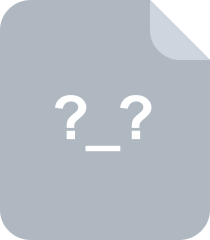
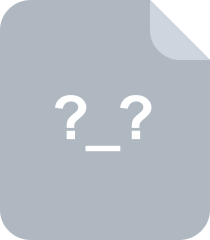
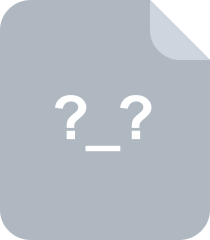
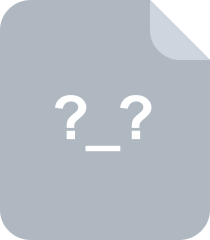
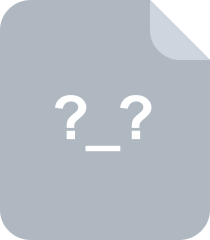
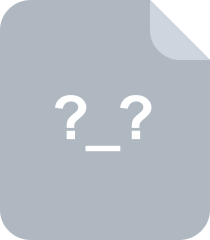
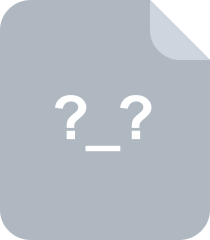
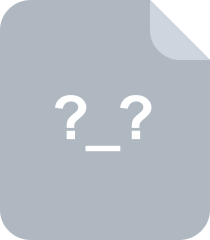
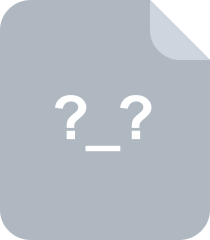
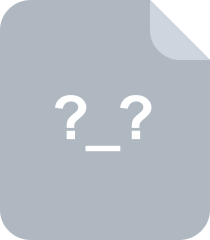
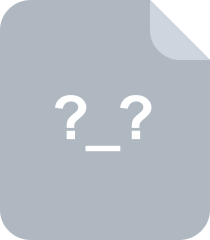
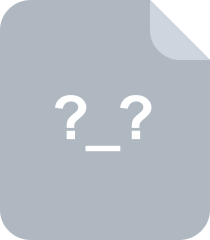
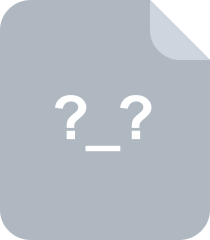
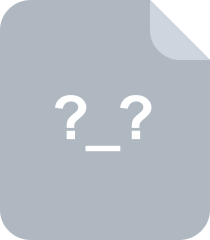
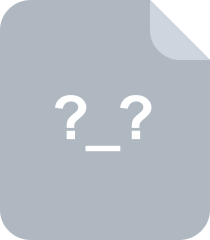
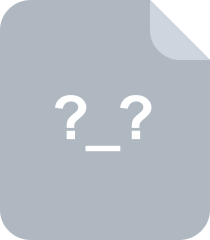
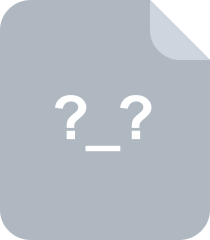
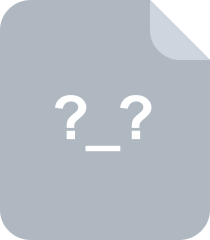
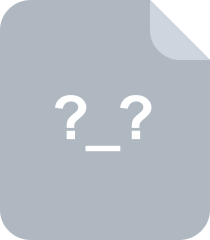
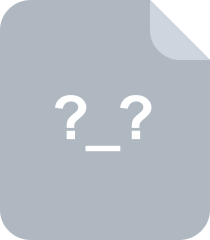
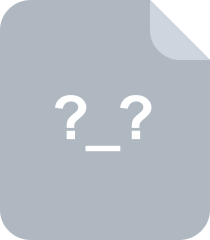
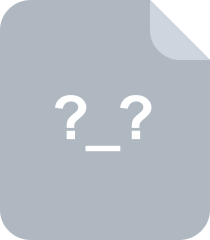
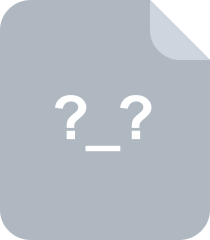
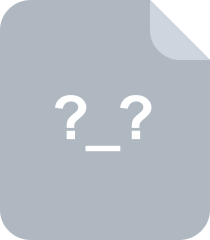
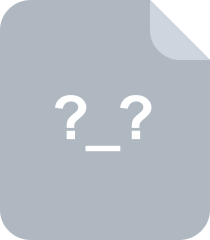
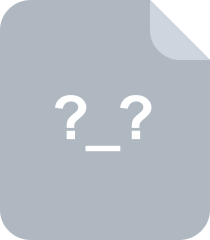
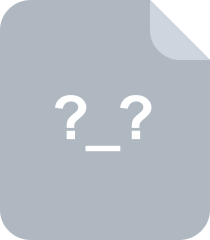
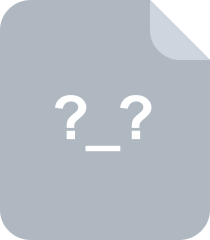
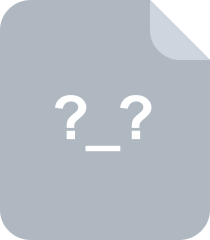
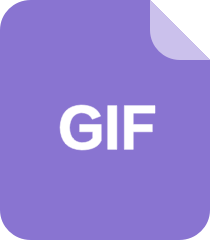
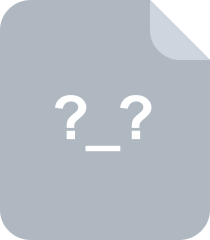
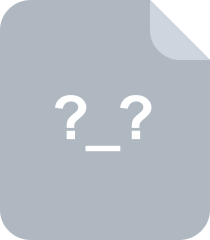
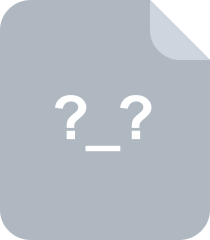
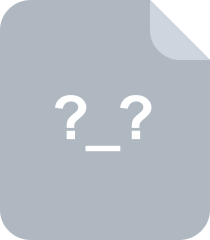
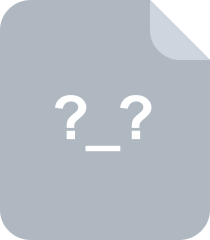
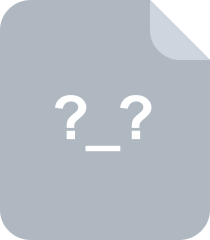
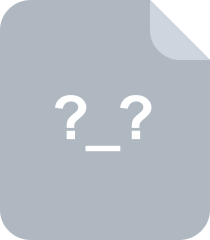
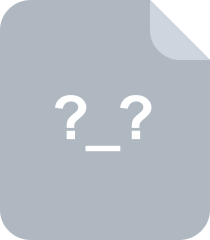
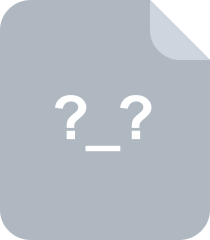
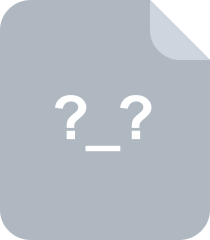
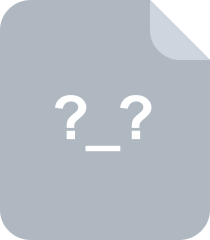
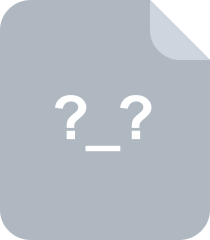
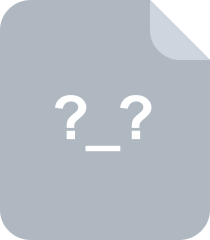
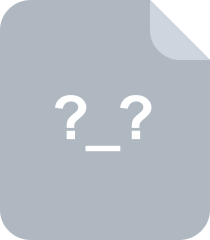
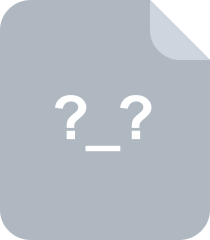
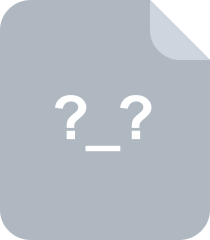
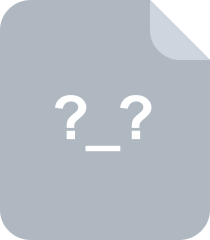
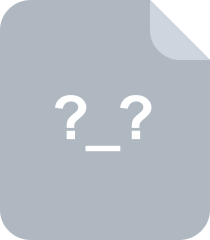
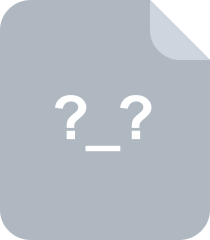
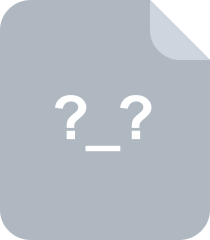
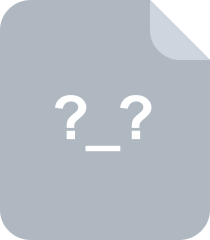
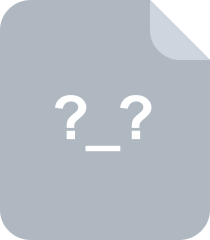
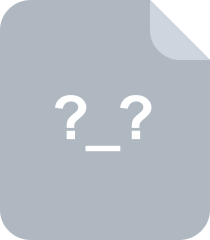
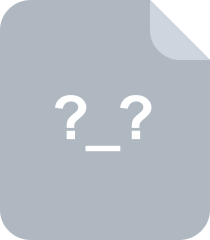
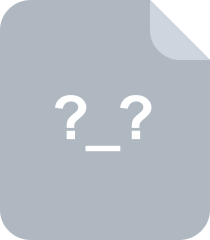
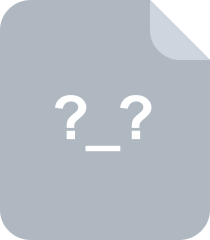
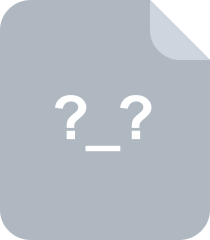
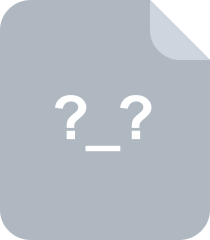
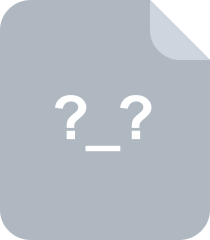
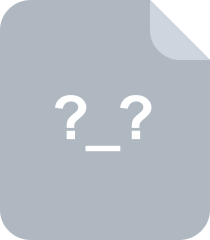
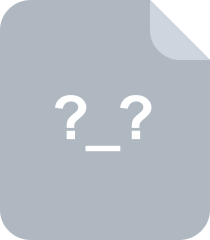
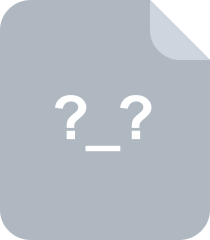
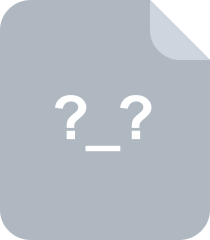
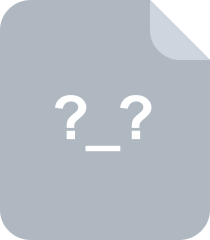
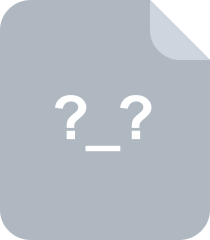
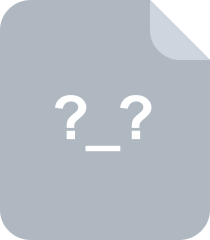
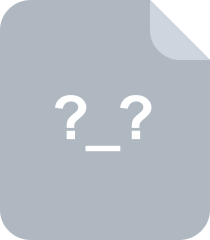
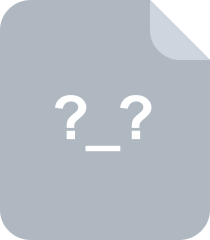
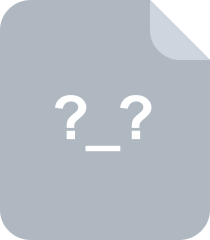
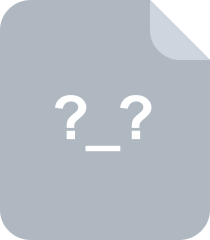
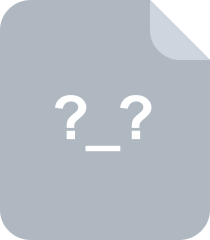
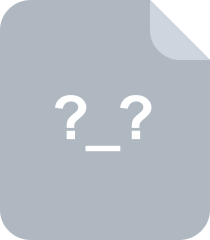
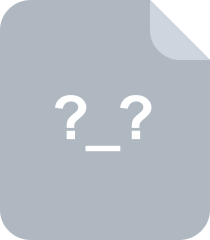
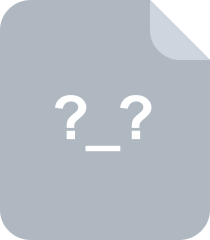
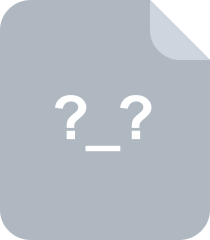
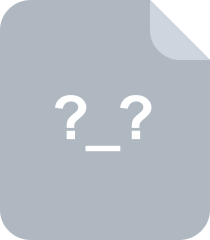
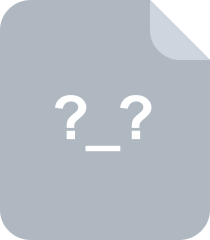
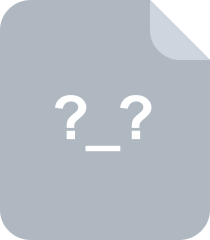
共 483 条
- 1
- 2
- 3
- 4
- 5
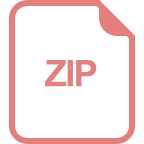
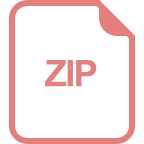
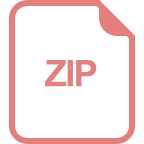
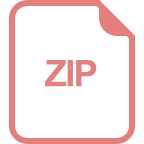
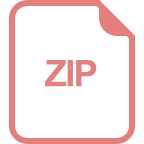
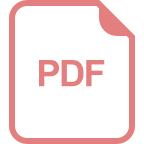
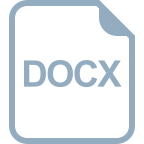
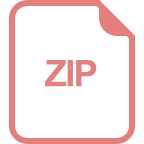
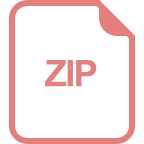
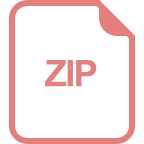
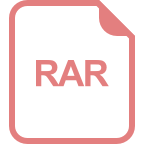
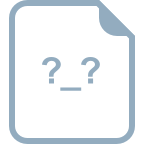
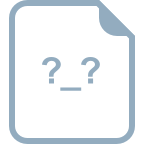
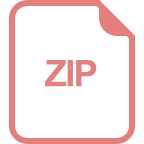
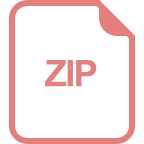
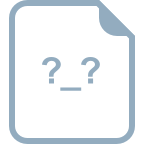
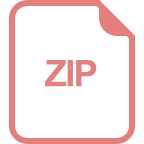
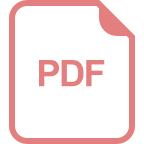
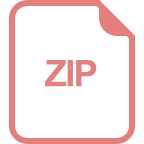
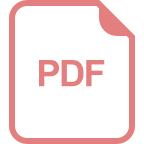
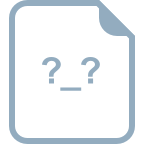

尽心致胜
- 粉丝: 23
- 资源: 4661
上传资源 快速赚钱
我的内容管理 展开
我的资源 快来上传第一个资源
我的收益
登录查看自己的收益我的积分 登录查看自己的积分
我的C币 登录后查看C币余额
我的收藏
我的下载
下载帮助

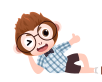
最新资源
- PCB原理图符号库(SchLib),电阻、电容、二极管、 三极管、变压器、继电器、芯片、电机驱动器、晶振等等
- 如果你用python3.12版本安装pygraphviz,需要安装如下的轮子 pygraphviz-1.12-cp312-cp3
- 世界地图+中国地图+各省(区市)地图
- 【java毕业设计】校园招聘网站的设计与实现源码(springboot+vue+mysql+说明文档+LW).zip
- 【java毕业设计】文物管理系统的设计与实现源码(springboot+vue+mysql+说明文档+LW).zip
- 12ba03d8a5035b51da096d1634834c66_1730793908707_1
- 如果你用python3.11版本安装pygraphviz,需要安装如下的轮子 pygraphviz-1.12-cp311
- ffmpeg-master-latest-win64-gpl-shared.zip
- boss直聘数据集,可以数据分析,选择合适的岗位
- ESAD1730793813076896NL88c917k17i394.zip
资源上传下载、课程学习等过程中有任何疑问或建议,欢迎提出宝贵意见哦~我们会及时处理!
点击此处反馈


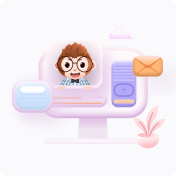
安全验证
文档复制为VIP权益,开通VIP直接复制

评论0