import os
import torch
import cv2
import numpy as np
from PIL import Image
import torch.nn.functional as F
import torch.nn as nn
from torch.utils.data import Dataset,DataLoader
import matplotlib.pyplot as plt
import torch.nn.functional as F
import torch.optim as optim
from torchvision import datasets, transforms
from torch.autograd import Variable
plt.rcParams['font.sans-serif'] = ['SimHei']
import gzip
import warnings
warnings.filterwarnings('ignore')
def load_data(data_folder, data_name, label_name):
with gzip.open(os.path.join(data_folder, label_name), 'rb') as lbpath: # rb表示的是读取二进制数据
y_train = np.frombuffer(lbpath.read(), np.uint8, offset=8)
with gzip.open(os.path.join(data_folder, data_name), 'rb') as imgpath:
x_train = np.frombuffer(
imgpath.read(), np.uint8, offset=16).reshape(len(y_train), 28, 28)
return (x_train, y_train)
class MyDataset(Dataset):
def __init__(self, folder, data_name, label_name, transform=None):
(train_set, train_labels) = load_data(folder, data_name,
label_name) # 其实也可以直接使用torch.load(),读取之后的结果为torch.Tensor形式
self.train_set = train_set
self.train_labels = train_labels
self.transform = transform
def __getitem__(self, index):
img, target = self.train_set[index], int(self.train_labels[index])
if self.transform is not None:
img = self.transform(img)
return img, target
def __len__(self):
return len(self.train_set)
#文件路径
file_path = dict()
train_file = dict()
test_file = dict()
train_file['fea'] = 'train-images-idx3-ubyte.gz'
train_file['label']='train-labels-idx1-ubyte.gz'
test_file['fea'] = 't10k-images-idx3-ubyte.gz'
test_file['label']='t10k-labels-idx1-ubyte.gz'
file_path['train'] = train_file.copy()
file_path['test'] = test_file.copy()
trainDataset = MyDataset('raw/',file_path['train']['fea'],file_path['train']['label'],transform=transforms.ToTensor())
testDataset = MyDataset('raw/',file_path['test']['fea'],file_path['test']['label'],transform=transforms.ToTensor())
train_loader = DataLoader(dataset=trainDataset,batch_size=100,shuffle=True,)
test_loader = DataLoader(dataset=testDataset,batch_size=100,shuffle=True,)
class NeuralNetwork(nn.Module):
def __init__(self,input_num,output_num):
super(NeuralNetwork, self).__init__()
self.layers = nn.ModuleDict({
'layer1': nn.Linear(input_num,512),
'layer2':nn.Linear(300,500),
'layer3':nn.Linear(500,200),
'layer4':nn.Linear(512,output_num)
})
self.activates = nn.ModuleDict({
'relu':nn.ReLU(),
'sigmoid':nn.Sigmoid()
})
def forward(self, x):
x = self.layers['layer1'](x)
x = self.activates['relu'](x)
# x = self.layers['layer2'](x)
# x = self.activates['relu'](x)
# x = self.layers['layer3'](x)
# x = self.activates['relu'](x)
x = self.layers['layer4'](x)
return x
# 参数初始化
epoches = 15
lr = 0.001
input_num = 784
output_num = 10
device = torch.device("cuda" if torch.cuda.is_available() else "cpu")
# 产生训练模型对象以及定义损失函数和优化函数
model = NeuralNetwork(input_num, output_num)
model.to(device)
criterion = nn.CrossEntropyLoss() # 使用交叉熵作为损失函数
optimizer = optim.SGD(model.parameters(), lr=lr)
# 开始循环训练
for epoch in range(epoches): # 一个epoch可以认为是一次训练循环
for i, data in enumerate(train_loader):
(images, labels) = data
images = images.reshape(-1, 28*28).to(device)
images = images.to(device)
labels = labels.to(device)
output = model(images) # 经过模型对象就产生了输出
loss = criterion(output, labels.long()) # 传入的参数: 输出值(预测值), 实际值(标签)
optimizer.zero_grad() # 梯度清零
loss.backward()
optimizer.step()
if (i+1) % 300 == 0: # i表示样本的编号
print('Epoch [{}/{}], Loss: {:.4f}'.format(epoch + 1, epoches, loss.item())) # {}里面是后面需要传入的变量# loss.item
# 测试
with torch.no_grad():
correct = 0
total = 0
for images, labels in test_loader:
images = images.reshape(-1, 28 * 28).to(device)
labels = labels.to(device)
output = model(images)
_, predicted = torch.max(output, 1)
total += labels.size(0)
correct += (predicted == labels).sum().item()
print("The accuracy of total {} images: {}%".format(total, 100 * correct / total))
img = cv2.imread('./777.jpg')
GrayImage=cv2.cvtColor(img,cv2.COLOR_BGR2GRAY)
# 中值滤波
GrayImage= cv2.medianBlur(GrayImage,5)
ret,th1 = cv2.threshold(GrayImage,130,255,cv2.THRESH_BINARY_INV )
img_test2 = cv2.resize(th1, (28, 28), interpolation=cv2.INTER_AREA)
test = torch.Tensor(img_test2)
images=test.reshape(-1, 28*28)
y_pred = model(images)
_, pred = torch.max(y_pred,1)
print('预测结果源数据:')
print('分割线'.center(100,'*'))
print(y_pred)
print('分割线'.center(100,'*'))
print('预测结果数字:')
print(int(pred[0]))
print('分割线'.center(100,'*'))
_ = plt.imshow(img)
plt.title("预测数字为: {}".format(pred[0]))
plt.show()
没有合适的资源?快使用搜索试试~ 我知道了~
手写数字识别,包含代码和数据
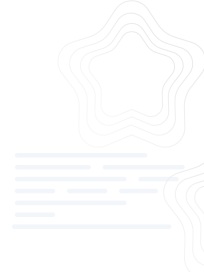
共27个文件
jpg:9个
xml:5个
gz:4个

需积分: 12 0 下载量 76 浏览量
2022-12-28
10:34:10
上传
评论
收藏 21.97MB ZIP 举报
温馨提示
手写数字识别,包含代码和数据
资源推荐
资源详情
资源评论
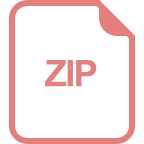
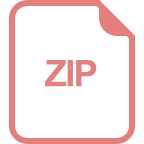
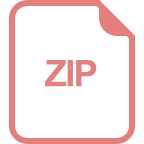
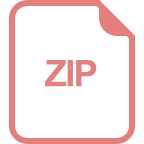
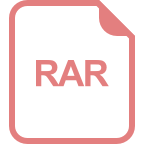
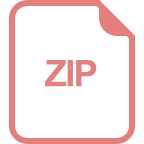
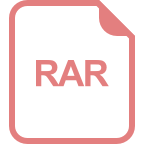
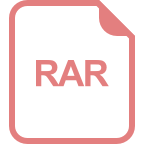
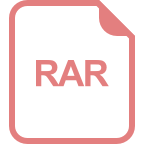
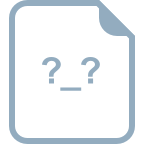
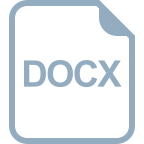
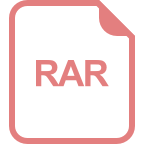
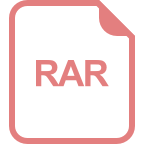
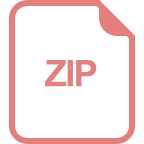
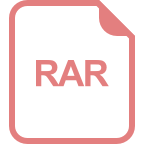
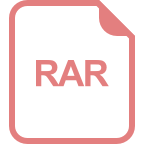
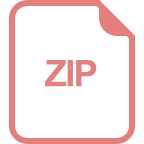
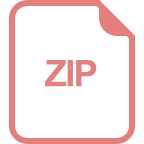
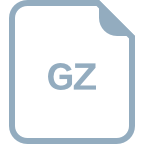
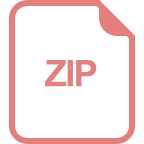
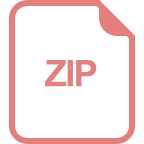
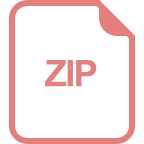
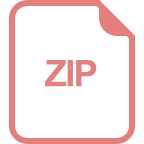
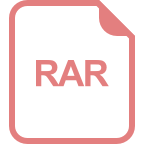
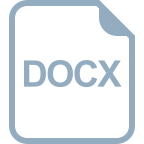
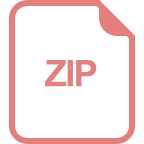
收起资源包目录


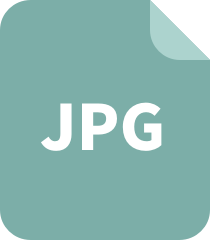
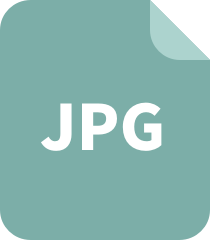
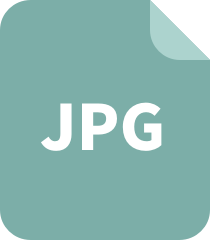
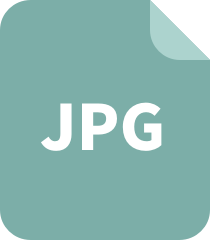
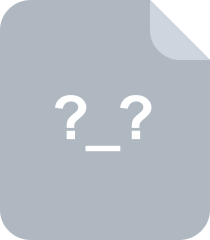
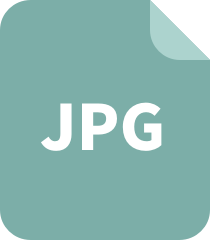

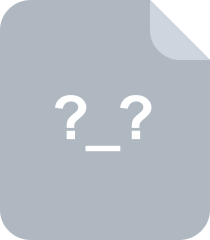
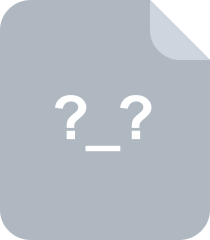
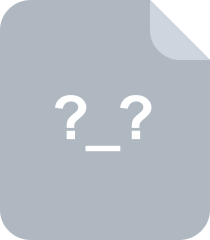

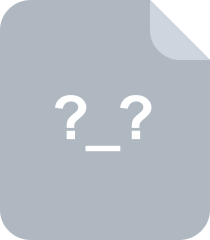
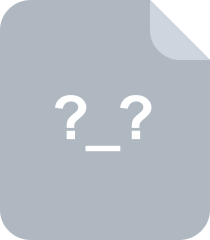
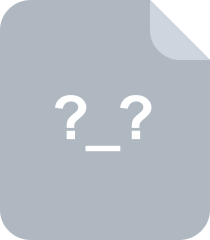
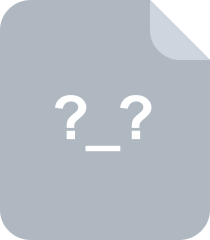
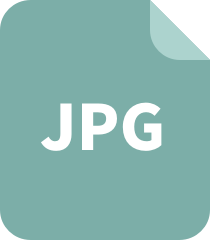
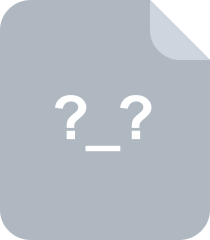
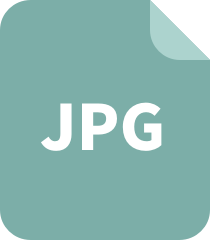
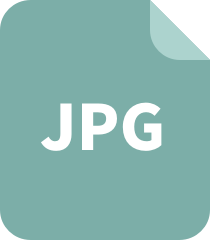

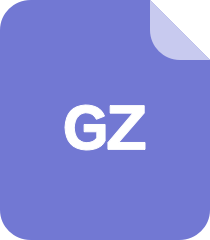
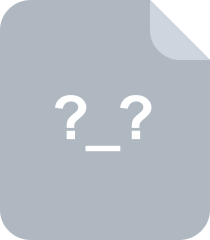
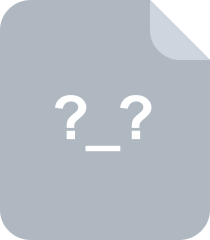
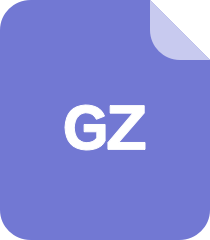
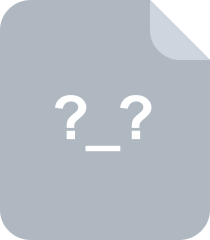
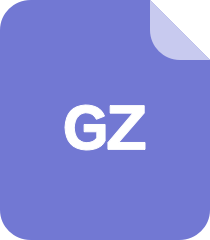
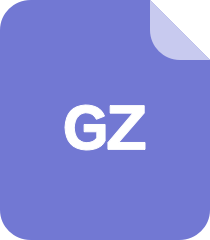
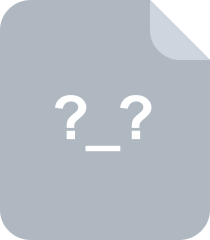
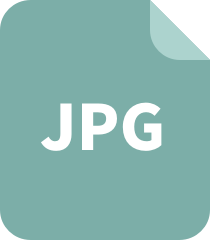
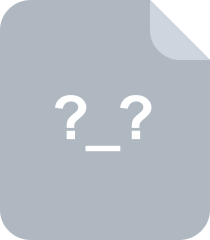
共 27 条
- 1
资源评论
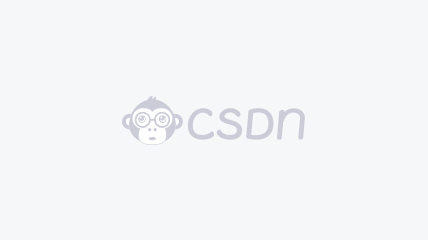

远方的旅行者
- 粉丝: 261
- 资源: 16
上传资源 快速赚钱
我的内容管理 展开
我的资源 快来上传第一个资源
我的收益
登录查看自己的收益我的积分 登录查看自己的积分
我的C币 登录后查看C币余额
我的收藏
我的下载
下载帮助

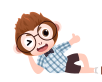
最新资源
- 柯尼卡美能达Bizhub C364e打印机驱动下载
- CMake 入门实战的源代码
- c7383c5d0009dfc59e9edf595bb0bcd0.zip
- 柯尼卡美能达Bizhub C266打印机驱动下载
- java游戏之我当皇帝那些年.zip开发资料
- 基于Matlab的汉明码(Hamming Code)纠错传输以及交织编码(Interleaved coding)仿真.zip
- 中国省级新质生产力发展指数数据(任宇新版本)2010-2023年.txt
- 基于Matlab的2Q-FSK移频键控通信系统仿真.zip
- 使用C++实现的常见算法
- travel-web-springboot【程序员VIP专用】.zip
资源上传下载、课程学习等过程中有任何疑问或建议,欢迎提出宝贵意见哦~我们会及时处理!
点击此处反馈


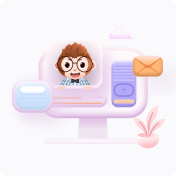
安全验证
文档复制为VIP权益,开通VIP直接复制
