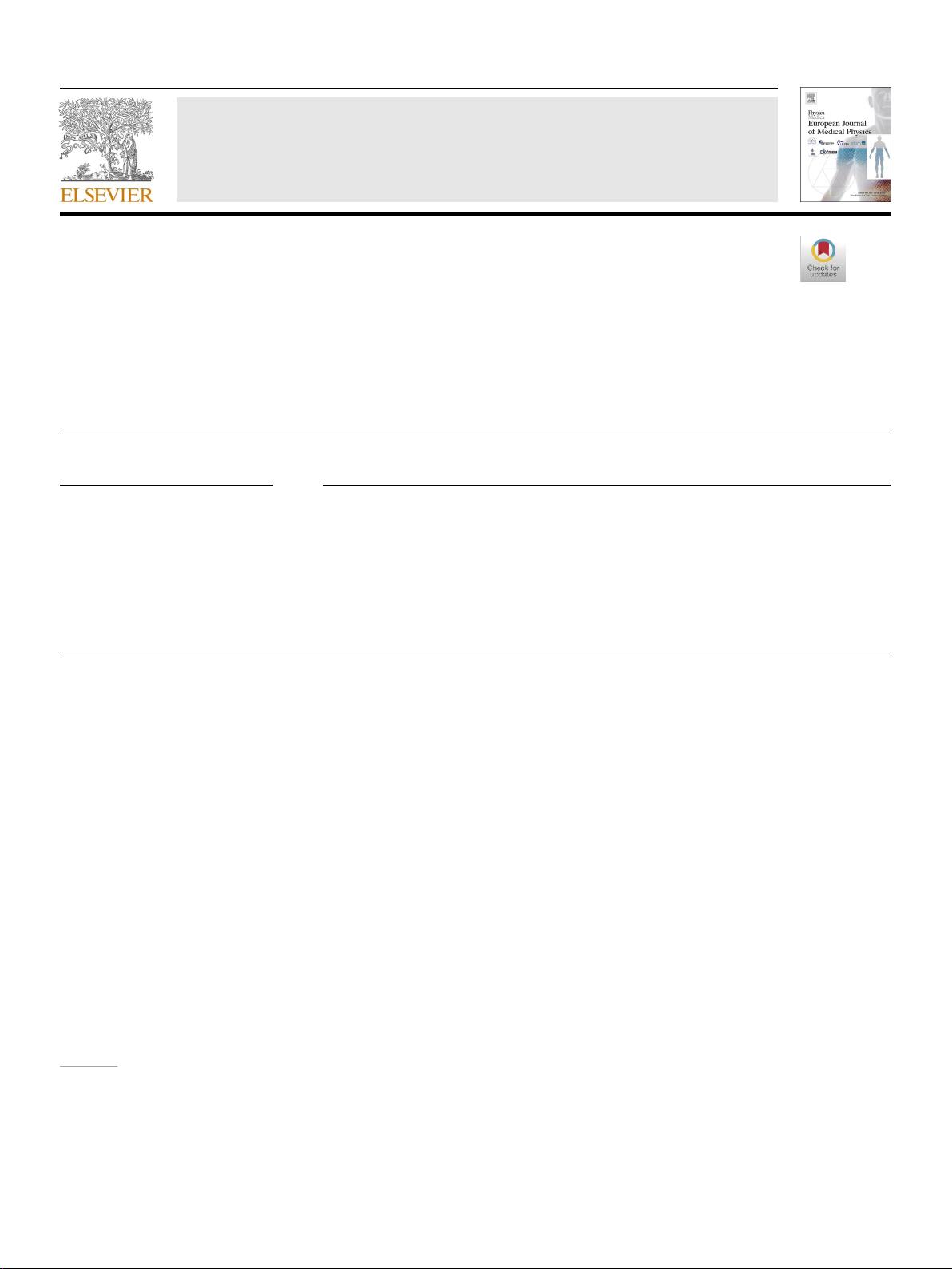
Physica Medica 83 (2021) 122–137
Original paper
The promise of artificial intelligence and deep learning in PET and
SPECT imaging
Hossein Arabi
a
, Azadeh AkhavanAllaf
a
, Amirhossein Sanaat
a
, Isaac Shiri
a
, Habib Zaidi
a,b,c,d,*
a
Division of Nuclear Medicine and Molecular Imaging, Geneva University Hospital, CH-1211 Geneva 4, Switzerland
b
Geneva University Neurocenter, Geneva University, CH-1205 Geneva, Switzerland
c
Department of Nuclear Medicine and Molecular Imaging, University of Groningen, University Medical Center Groningen, 9700 RB Groningen, Netherlands
d
Department of Nuclear Medicine, University of Southern Denmark, 500 Odense, Denmark
A R T I C L E I N F O
Keywords:
Molecular imaging
PET
SPECT
Artificial intelligence
Deep learning
A B S T R A C T
This review sets out to discuss the foremost applications of artificial intelligence (AI), particularly deep learning
(DL) algorithms, in single-photon emission computed tomography (SPECT) and positron emission tomography
(PET) imaging. To this end, the underlying limitations/challenges of these imaging modalities are briefly dis-
cussed followed by a description of AI-based solutions proposed to address these challenges. This review will
focus on mainstream generic fields, including instrumentation, image acquisition/formation, image recon-
struction and low-dose/fast scanning, quantitative imaging, image interpretation (computer-aided detection/
diagnosis/prognosis), as well as internal radiation dosimetry. A brief description of deep learning algorithms and
the fundamental architectures used for these applications is also provided. Finally, the challenges, opportunities,
and barriers to full-scale validation and adoption of AI-based solutions for improvement of image quality and
quantitative accuracy of PET and SPECT images in the clinic are discussed.
Introduction
Artificial intelligence (AI) approaches, particularly deep learning
(DL) techniques, have received tremendous attention during the last
decade owing to their remarkable success in offering novel solutions to
solve complex problems. Novel AI/DL-based solutions have created
opportunities in clinical and research settings to automate a number of
tasks deemed to depend on human cognition, and hence require his
intervention to facilitate the decision-making process [1]. State-of-the-
art AI/DL algorithms have exhibited exceptional learning capability
from high dimensional and/or highly complex data, accomplishing
daunting challenging tasks in image and data analysis/processing in
general and multimodality medical imaging in particular.
In the context of medical imaging, challenging tasks, such as image
segmentation/classification, data correction (such as noise or artifact
reduction), image interpretation (prognosis, diagnosis, and monitoring
of response to treatment), cross-modality image translation or synthesis,
and replacing computationally demanding algorithms (such as Monte
Carlo calculations) have been broadly revisited and evolved ever since
the adoption of deep learning approaches [2,3]. AI-based solutions have
been proposed to address the fundamental limitations/challenges faced
by image acquisition and analysis procedures on modern molecular
imaging technologies. Considering the superior performance of deep
learning approaches compared to conventional techniques, a paradigm
shift is expected to occur provided that task-specific pragmatic de-
velopments of these algorithms continue to evolve in the right direction.
Single-photon emission computed tomography (SPECT) and Positron
emission tomography (PET) imaging provide the in vivo radiotracer ac-
tivity distribution maps, representative of biochemical processes in
humans and animal species. The introduction of hybrid imaging
combining functional and anatomical imaging modalities in the form of
combined PET/CT and PET/MRI systems has remarkably thrived the
widespread adoption and proliferation of these modalities in clinical
practice. In this light, AI-based algorithms/solutions are developed to
overcome the major shortcomings or to enhance the current function-
ality of these modalities.
The applications of AI-based algorithms in PET and SPECT imaging
ranges from low-level electronic signal formation/processing to high-
level internal dosimetry and diagnostic/prognostic modeling. For de-
velopments in instrumentation, deep learning approaches have been
mostly employed to improve the timing resolution and localization ac-
curacy of the incident photons aiming at enhancing the overall spatial
* Corresponding author.
E-mail address: habib.zaidi@hcuge.ch (H. Zaidi).
https://doi.org/10.1016/j.ejmp.2021.03.008
Received 27 December 2020; Received in revised form 18 February 2021; Accepted 3 March 2021
Available online 22 March 2021
1120-1797/© 2021 Associazione Italiana di Fisica Medica. Published by Elsevier Ltd. This is an open access article under the CC BY-NC-ND license
(http://creativecommons.org/licenses/by-nc-nd/4.0/).
Contents lists available at ScienceDirect
Physica Medica
journal homepage: www.elsevier.com/locate/ejmp