没有合适的资源?快使用搜索试试~ 我知道了~
资源推荐
资源详情
资源评论
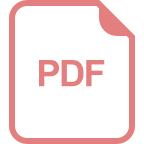
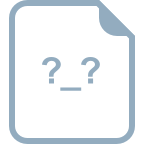
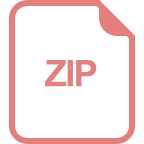
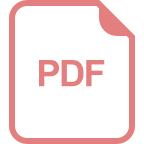
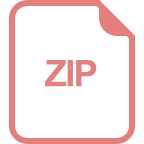
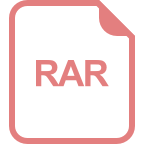
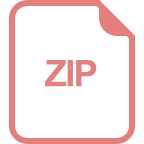
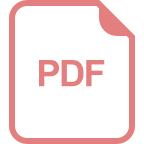
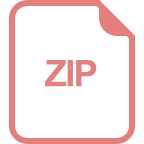
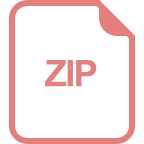
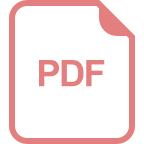
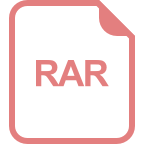
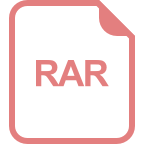
from tensorflow.examples.tutorials.mnist import input_data
import tensorflow as tf
import argparse
import numpy as np
import os
from tensorflow.python import pywrap_tensorflow
from PIL import Image
def weight_variable(shape, dtype, name):
initial = tf.truncated_normal(shape = shape, stddev = 0.1, dtype = dtype, name = name)
return tf.Variable(initial)
def bias_variable(shape, dtype, name):
initial = tf.constant(0.1, shape = shape, dtype = dtype, name = name)
return tf.Variable(initial)
def conv2d(x, W):
return tf.nn.conv2d(x, W, strides = [1, 1, 1, 1], padding = 'SAME')
def max_pool_2x2(x):
return tf.nn.max_pool(x, ksize = [1, 2, 2, 1], strides = [1, 2, 2, 1], padding = 'SAME')
mnist_data = input_data.read_data_sets("MNIST_data", one_hot = True)
x = tf.placeholder("float", [None, 784],name="x")
y = tf.placeholder("float", [None, 10],name="y")
x_image = tf.reshape(x, [-1, 28, 28, 1])
# convolution 1
weight_conv1 = weight_variable([5, 5, 1, 32], dtype = "float", name = 'weight_conv1')
import tensorflow as tf
import argparse
import numpy as np
import os
from tensorflow.python import pywrap_tensorflow
from PIL import Image
def weight_variable(shape, dtype, name):
initial = tf.truncated_normal(shape = shape, stddev = 0.1, dtype = dtype, name = name)
return tf.Variable(initial)
def bias_variable(shape, dtype, name):
initial = tf.constant(0.1, shape = shape, dtype = dtype, name = name)
return tf.Variable(initial)
def conv2d(x, W):
return tf.nn.conv2d(x, W, strides = [1, 1, 1, 1], padding = 'SAME')
def max_pool_2x2(x):
return tf.nn.max_pool(x, ksize = [1, 2, 2, 1], strides = [1, 2, 2, 1], padding = 'SAME')
mnist_data = input_data.read_data_sets("MNIST_data", one_hot = True)
x = tf.placeholder("float", [None, 784],name="x")
y = tf.placeholder("float", [None, 10],name="y")
x_image = tf.reshape(x, [-1, 28, 28, 1])
# convolution 1
weight_conv1 = weight_variable([5, 5, 1, 32], dtype = "float", name = 'weight_conv1')
bias_conv1 = bias_variable([32], dtype = "float", name = 'bias_conv1')
hidden_conv1 = tf.nn.relu(conv2d(x_image, weight_conv1) + bias_conv1)
hidden_pool1 = max_pool_2x2(hidden_conv1)
# convolution 2
weight_conv2 = weight_variable([5, 5, 32, 64], dtype = "float", name = 'weight_conv2')
bias_conv2 = bias_variable([64], dtype = "float", name = 'bias_conv2')
hidden_conv2 = tf.nn.relu(conv2d(hidden_pool1, weight_conv2) + bias_conv2)
hidden_pool2 = max_pool_2x2(hidden_conv2)
# function 1
hidden_pool2_flat = tf.reshape(hidden_pool2, [-1, 7 * 7 * 64])
weight_fc1 = weight_variable([7 * 7 * 64, 1024], dtype = "float", name = 'weight_fc1')
bias_fc1 = bias_variable([1024], dtype = "float", name = 'bias_fc1')
hidden_fc1 = tf.nn.relu(tf.matmul(hidden_pool2_flat, weight_fc1) + bias_fc1)
keep_prob = tf.placeholder("float" ,name="keep_prob")
hidden_fc1_dropout = tf.nn.dropout(hidden_fc1, keep_prob)
# function 2
weight_fc2 = weight_variable([1024, 10], dtype = "float", name = 'weight_fc2')
bias_fc2 = bias_variable([10], dtype = "float", name = 'weight_fc2')
y_fc2 = tf.nn.softmax(tf.matmul(hidden_fc1_dropout, weight_fc2) + bias_fc2 ,name="y_fc2")
cross_entropy = -tf.reduce_sum(y * tf.log(y_fc2))
optimize = tf.train.AdamOptimizer(0.0001)
train = optimize.minimize(cross_entropy)
correct_prediction = tf.equal(tf.argmax(y, 1), tf.argmax(y_fc2, 1))
accuracy = tf.reduce_mean(tf.cast(correct_prediction, "float"))
hidden_conv1 = tf.nn.relu(conv2d(x_image, weight_conv1) + bias_conv1)
hidden_pool1 = max_pool_2x2(hidden_conv1)
# convolution 2
weight_conv2 = weight_variable([5, 5, 32, 64], dtype = "float", name = 'weight_conv2')
bias_conv2 = bias_variable([64], dtype = "float", name = 'bias_conv2')
hidden_conv2 = tf.nn.relu(conv2d(hidden_pool1, weight_conv2) + bias_conv2)
hidden_pool2 = max_pool_2x2(hidden_conv2)
# function 1
hidden_pool2_flat = tf.reshape(hidden_pool2, [-1, 7 * 7 * 64])
weight_fc1 = weight_variable([7 * 7 * 64, 1024], dtype = "float", name = 'weight_fc1')
bias_fc1 = bias_variable([1024], dtype = "float", name = 'bias_fc1')
hidden_fc1 = tf.nn.relu(tf.matmul(hidden_pool2_flat, weight_fc1) + bias_fc1)
keep_prob = tf.placeholder("float" ,name="keep_prob")
hidden_fc1_dropout = tf.nn.dropout(hidden_fc1, keep_prob)
# function 2
weight_fc2 = weight_variable([1024, 10], dtype = "float", name = 'weight_fc2')
bias_fc2 = bias_variable([10], dtype = "float", name = 'weight_fc2')
y_fc2 = tf.nn.softmax(tf.matmul(hidden_fc1_dropout, weight_fc2) + bias_fc2 ,name="y_fc2")
cross_entropy = -tf.reduce_sum(y * tf.log(y_fc2))
optimize = tf.train.AdamOptimizer(0.0001)
train = optimize.minimize(cross_entropy)
correct_prediction = tf.equal(tf.argmax(y, 1), tf.argmax(y_fc2, 1))
accuracy = tf.reduce_mean(tf.cast(correct_prediction, "float"))
剩余6页未读,继续阅读
资源评论
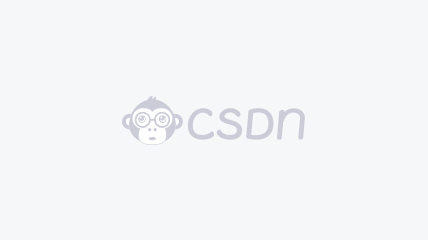

weixin_38833526
- 粉丝: 0
- 资源: 3
上传资源 快速赚钱
我的内容管理 展开
我的资源 快来上传第一个资源
我的收益
登录查看自己的收益我的积分 登录查看自己的积分
我的C币 登录后查看C币余额
我的收藏
我的下载
下载帮助

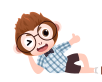
最新资源
- 数据库设计管理课程设计系统设计报告(powerdesign+sql+DreamweaverCS)超市管理系统设计与开发2
- Go 学习教程(入门到实践)
- 数据库设计管理课程设计系统设计报告(powerdesign+sql+DreamweaverCS)超市管理系统设计与开发
- 毕设新作-python基于深度学习(多种模型)的医学图像分割和诊断平台源码+模型+说明文档.zip
- 数据库设计管理课程设计系统设计报告(powerdesign+sql+DreamweaverCS)仓库管理系统设计与开发2
- 数据库设计管理课程设计系统设计报告(powerdesign+sql+DreamweaverCS)仓库管理系统设计与开发
- Visual Basic 学习教程(入门到实践)
- CocosCreator开发视频教程含源码跳一跳开发教程非Creator开发200M
- 随便写的仓库管理系统.zip,瞎看看就行
- Scratch 学习教程(入门到实践)
资源上传下载、课程学习等过程中有任何疑问或建议,欢迎提出宝贵意见哦~我们会及时处理!
点击此处反馈


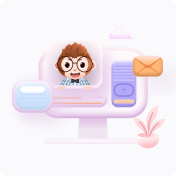
安全验证
文档复制为VIP权益,开通VIP直接复制
