没有合适的资源?快使用搜索试试~ 我知道了~
温馨提示
3D human pose estimation is a challenging but important research topic with abundant applications. As for discriminative human pose estimation, the main goal is to learn a nonlinear mapping from image descriptors to 3D human pose configurations, which is difficult due to the high-dimensionality of human pose space and the multimodality of the distribution. To address these problems, we propose a novel motionlet LLC coding on a discriminative framework. A motionlet consists of training examples c
资源推荐
资源详情
资源评论
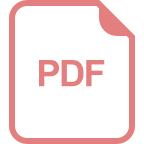
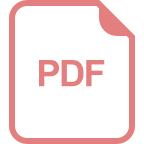
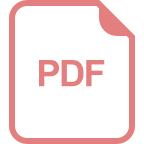
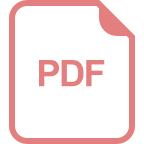
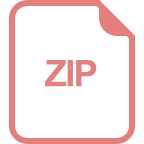
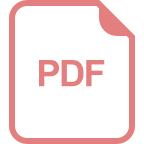
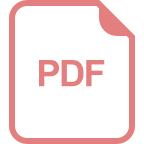
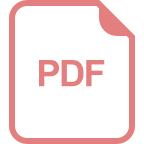
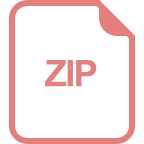
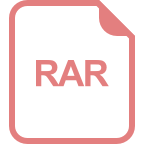
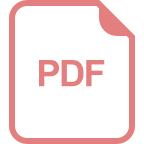
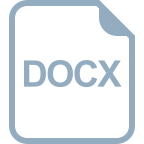
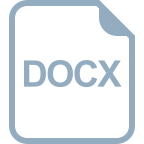
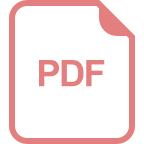
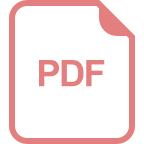
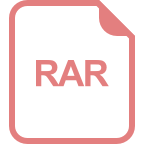
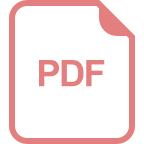
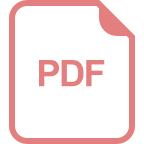
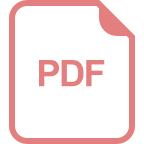
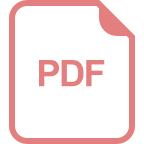
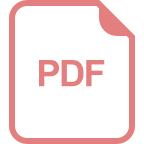
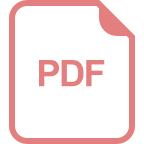
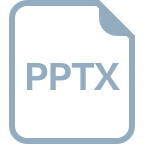
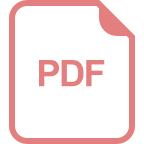
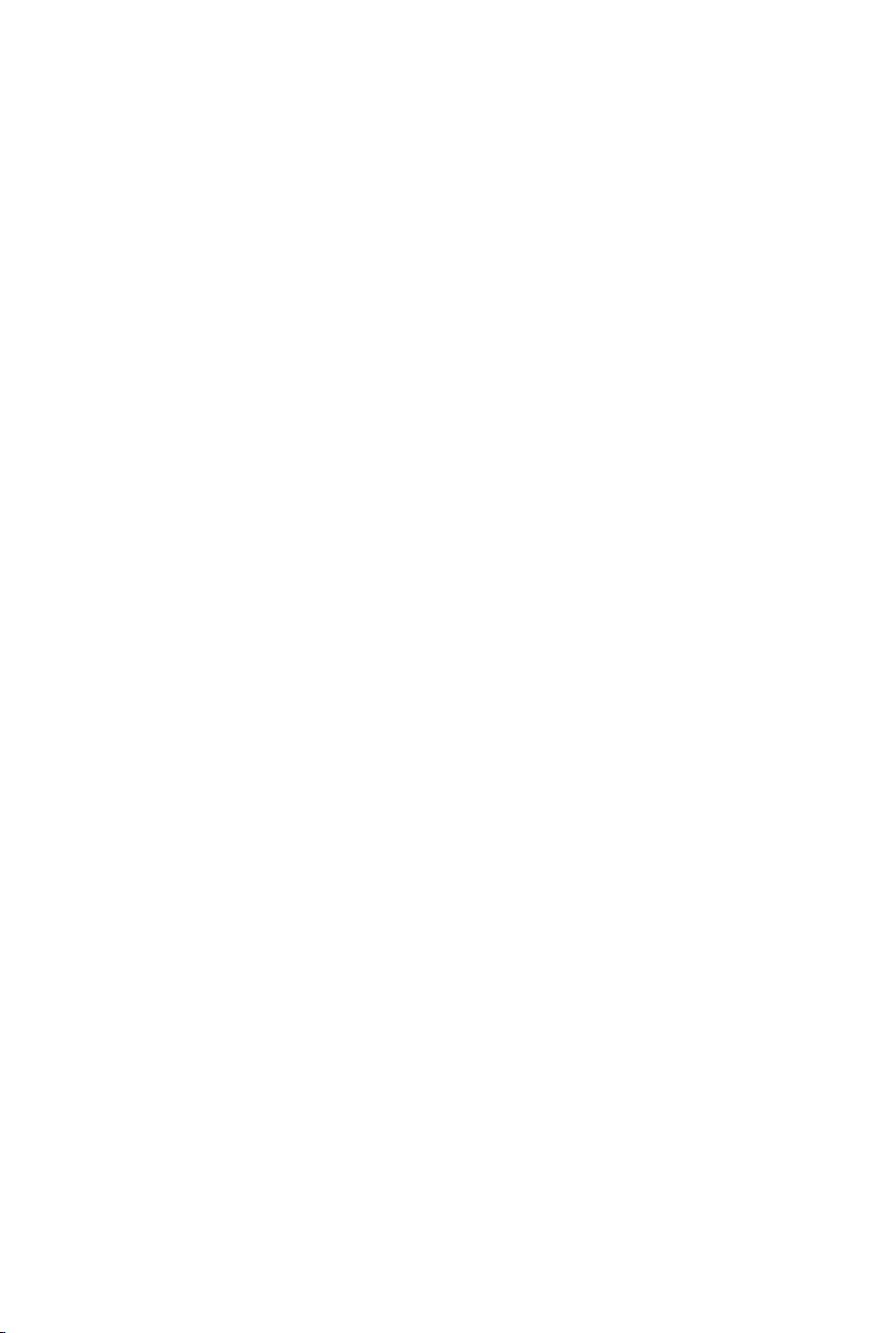
Pose Estimation with Motionlet LLC Coding
Li Sun, Mingli Song, Jiajun Bu, and Chun Chen
Zhejiang Provincial Key Laboratory of Service Robot,
College of Computer Science, Zhejiang University
{lsun,brooksong,bjj,chenc}@zju.edu.cn
Abstract. 3D human pose estimation is a challenging but important
research topic with abundant applications. As for discriminative human
pose estimation, the main goal is to learn a nonlinear mapping from im-
age descriptors to 3D human pose configurations, which is difficult due
to the high-dimensionality of human pose space and the multimodal-
ity of the distribution. To address these problems, we propose a novel
motionlet LLC coding on a discriminative framework. A motionlet con-
sists of training examples covering a local area in terms of image space,
pose space and time stream. We first group most informative and help-
ful training examples into motionlets, then perform LLC Coding to learn
the nonlinear mapping and get candidate poses, and finally choose the
most appropriate pose as the result estimate. To further eliminate am-
biguities and improve robustness, we extend our framework to incorpo-
rate multiviews. We conduct qualitative evaluation on our Taichi data
set and quantitative evaluation on HumanEva data set, which show that
our approach has gained the-state-of-the-art performance and significant
improvement against previous approaches.
Keywords: human pose estimation, multimodality, multiview, motion-
let, LLC coding.
1 Introduction
3D human pose estimation from images is a challenging but important research
topic with applications in many areas including Human-Computer Interaction,
robotics, surveillance, computer graphics and sport science. Recent approaches
to 3D human pose estimation can be roughly classified into two categories, gen-
erative and discriminative. Generative approaches explicitly model human body
appearance and kinematic constraints and usually concentrate on development
of efficient inference methods that are able to handle the high dimensionality of
human pose. Discriminative approaches directly learn the mapping from image
space to pose space.
Discriminative approaches are popular due to their flexibility of choosing
image descriptors, easy adaptation to different learning methods, no need for
initialization, and most importantly, the ability of fast inference in real-world
databases. The main goal of discriminative 3D human pose estimation is to
W. Lin et al. (Eds.): PCM 2012, LNCS 7674, pp. 435–443, 2012.
c
Springer-Verlag Berlin Heidelberg 2012
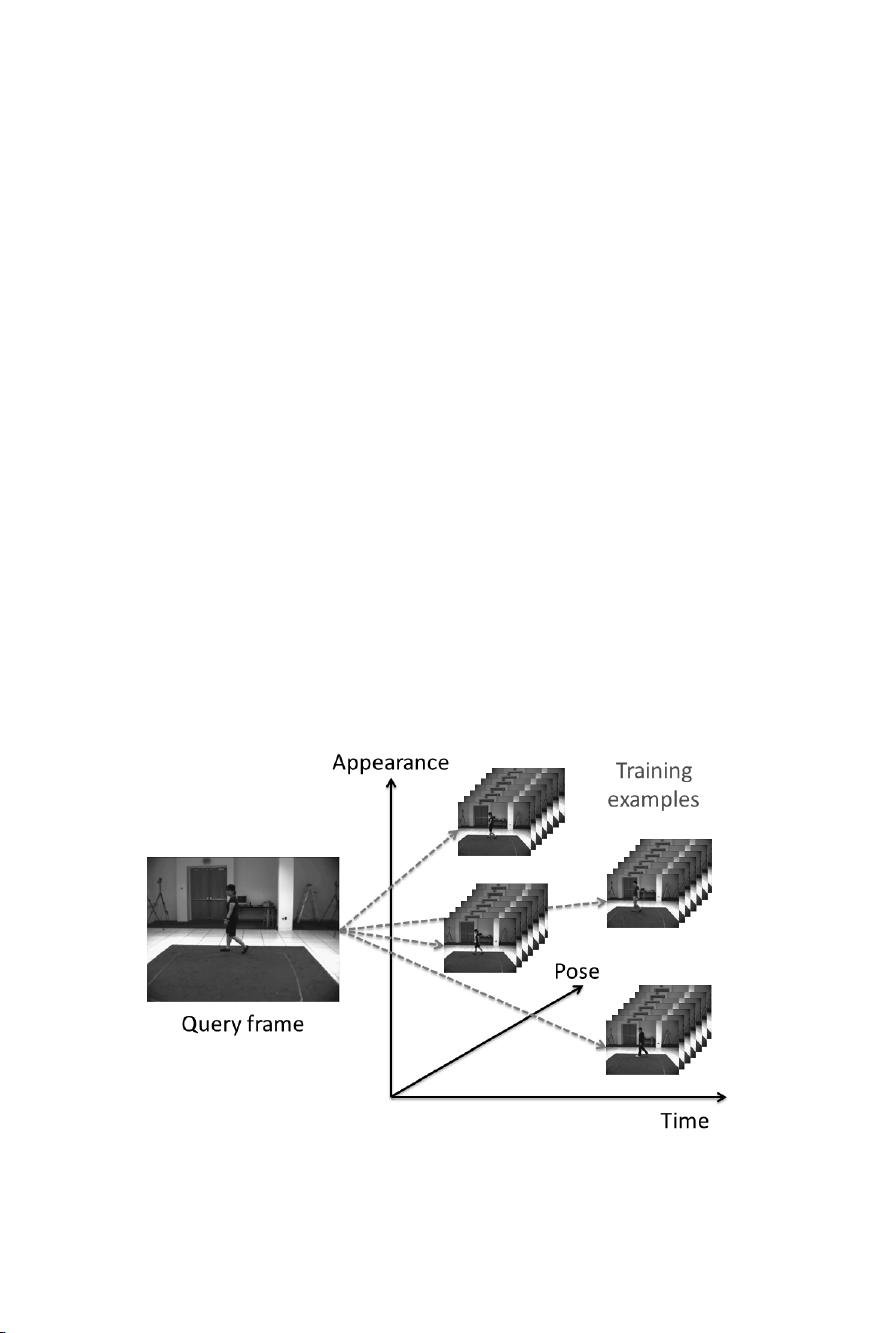
436 L. Sun et al.
learn a nonlinear mapping from image descriptors to 3D human pose configura-
tions. This is challenging due to high-dimensionality and multimodality of the
mapping. Moreover, the mapping is highly noisy because of image ambiguities
and subject variations.
In this paper we present a novel discriminative framework that can learn a
complex mapping from image descriptors to 3D human pose configurations. We
propose a local online approach to select most informative and helpful training
examples for the query frame, and then group them into motionlets. As depicted
by fig. 1, every motionlet consists of training examples that covers a local area
with respect to image space, pose space and time stream. The concept of mo-
tionlets is a natural embodiment of the local motion similarity of human motion,
which is the basis assumption of discriminative human pose estimation. We take
advantage of Locality-constrained Linear Coding (LLC) algorithm [7] to recon-
struct 3D human poses using motionlets as codebooks. LLC offers an efficient
local smooth sparse projection of an image descriptor into its local-coordinate
system with good reconstruction. Each motionlet contributes a candidate pose.
We handle the problem of multimodality through selecting the most appropri-
ate pose from these candidate poses. To further eliminate inference ambiguities,
we extend our framework to incorporate multiviews and retain an accurate and
robust inference from image descriptors to 3D human poses.
We review related work in the next section, and then present our online frame-
work of motionlet LLC coding. We define local neighborhoods for a query frame,
and then show that multimodality of the mapping is mainly caused by the multi-
ple instances of motionlets. We demonstrate how to choose from candidate poses
recovered by LLC coding and how to incorporate multiviews into our framework.
Fig. 1. Motionlets for a query frame. Each motionlet consists of training examples that
cover a local region in terms of appearance space, pose space and time stream.
剩余8页未读,继续阅读
资源评论
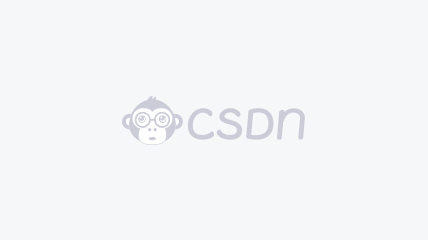

weixin_38746926
- 粉丝: 12
- 资源: 994
上传资源 快速赚钱
我的内容管理 展开
我的资源 快来上传第一个资源
我的收益
登录查看自己的收益我的积分 登录查看自己的积分
我的C币 登录后查看C币余额
我的收藏
我的下载
下载帮助

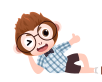
最新资源
- 新年倒计时网页基础教程
- Python编程初学者快速入门基础教程
- 新年倒计时编程基础教程
- 峰会报告自动化处理基础教程
- UE4UE5游戏开发基础教程:从零开始构建你的世界
- DataStructure-拓扑排序
- Front-end-learning-to-organize-notes-新年主题资源
- QPython Plus-Python资源
- baidulite-新年主题资源
- CnOCR-Python资源
- Golang_Puzzlers-新年主题资源
- Python开源扫雷游戏PyMine-Python资源
- Golang_Puzzlers-新年主题资源
- pyporter-Python资源
- Golang_Puzzlers-新年主题资源
- mulan-rework-Python资源
资源上传下载、课程学习等过程中有任何疑问或建议,欢迎提出宝贵意见哦~我们会及时处理!
点击此处反馈


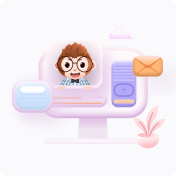
安全验证
文档复制为VIP权益,开通VIP直接复制
