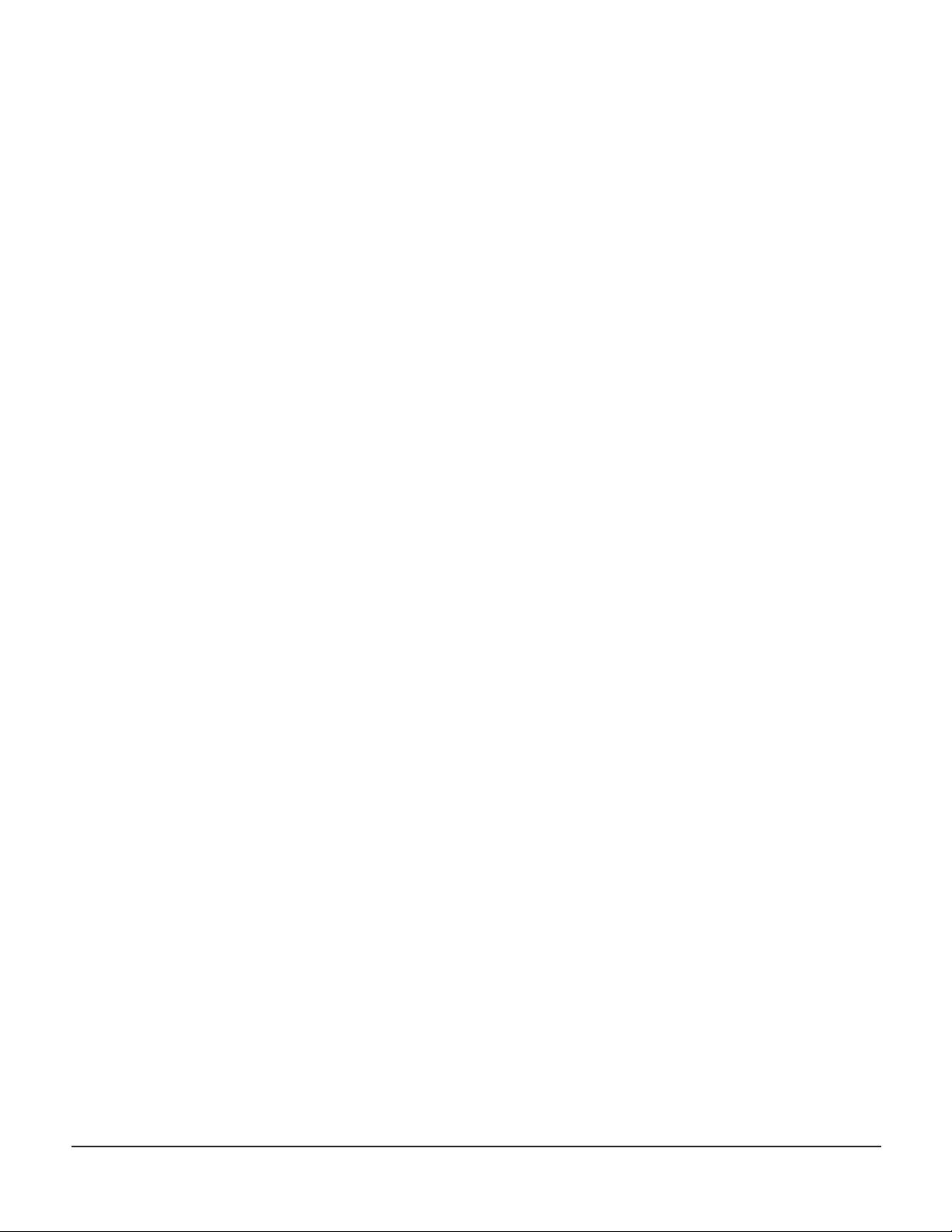
Oncotarget2
www.impactjournals.com/oncotarget
the curative ratio of NSCLC, we need to clearly know
more driver genes about the relationship between each
other, how to inuence NSCLC, and targeted therapies.
How to identify driver genes?
As we all known, identifying driver genes in a
typical tumor is critical to promote the development about
clinical therapeutics. Now, there have been two databases
to identify driver gene, Driver DB [11] (an exome
sequencing database for cancer driver gene identication)
and the Candidate Cancer Gene Database [12] (a database
of cancer driver gene from forward genetic screens in
mice), because of the advance of exome sequencing
[13, 14]. Driver DB provides the calculated results about
screening driver genes for a cancer by eight algorithms,
the explanation on relationships among driver genes (Gene
Oncology, Pathway and Protein/Genetics Interaction), the
different mutation information of per driver gene, Meta-
Analysis function, and so on. While the Candidate Cancer
Gene Databases (CCGD) includes a unied description
of candidate driver genes overall recently published and
the genomic locations, which are transposon common
insertion sites originated from transposon-based screens.
The arising of these databases have not only brought the
great convenience for the identication about driver genes
but also furthered the efciency of cancer research.
The prediction of driver genes
Accompanying with the arising of NGS and
extensive data sets derived from cancer omics, there
have been diversied methods to predict driver genes
in a special tumor, proling [15]. At present, two major
strategies based on the mutation frequency or functional
analysis of variant protein originated from gene mutation
apply to identify and predict driver genes. Generally,
the former infers whether one gene is driver gene by the
means of comparing the mutation frequency of single
locus or other loci between the same or similar cancer
[16, 17]. Whereas some researchers think that this method
on the strength of the pattern of mutation is superior
to the one based on mutation frequency. Extremely
characteristic, as well as nonrandom, is the patterns of
mutation about suppressor genes and oncogenes, which
was well studied. Thus, the patterns of mutation can
make us rapid to classify one driver gene as oncogene or
suppressor gene, contributing to the next step research.
However, how to distinguish oncogenes from suppressor
gene just according to the pattern of mutation remains to
be further studied [18].
While the latter predict driver genes through
inferring the function of variant protein generated from
genes mutation [19]. It is easy for the mut-driver genes
with high mutation frequency to identify through the
method based on mutation frequency, yet, which is
not suitable for the mut-driver genes that possess low
frequency and play a crucial role in the tumor genesis. This
problem is overcame by the method based on the function
analysis of variant protein. In fact, it is impossible for all
variant protein to confer the selective growth advantage,
which is the severe weakness of the functional analysis.
Similarly, there are a large of mutation genes with high
mutation frequency but helpless to the development of
tumor. In conclusion, the driver genes predicted by these
strategies remains subsequent analysis and experimental
verication.
As for Epi-driver gene, the further analysis of
differential expressed genes through comparing the
expression of genes between cancer tissues and normal
tissues of change is the dominating strategy to identify
the driver gene. The mutation of epi-driver genes often
occurred during the proliferation of cells because it is
the phase that DNA or chromatin is prone to be damaged
by DNA methylation, histone modication (histone
methylation and histone acetylation), and the DNA repair
dysregulation [29]. In addition, the genes expression
may be interrelated with ages of organisms, cell type,
and environmental factors besides regulatory factors.
Therefore, how to distinguish epi-driver genes from other
factors who result in the variation of genes expression is a
signicant challenge to identify driver genes.
Surely, there have been emerging many algorithms
to screen driver gene. For example, Lei Chen et proposed
a computational method to identify lung adenocarcinoma
drivers according to the methylation, mutation, microRNA,
and mRNA levels on the dysfunctional genes [20].
However, none of the existing algorithms, at present,
became the gold standard. Every algorism has own too
special stresses and weakness to easily make comparisons
about the results predicting driver genes by different
algorithms; that is, it is the best choice for these algorisms
to be used to screen driver genes in order to further analysis
but not identication. Now, some reviews and databases
display the outcomes of several algorithms and even form
a system that have a relatively higher accuracy on the
predicting driver genes for a specic cancer. DriverDBv2
have published bioinformatics algorithms dedicated to
driver gene or mutation identication; the ‘Cancer’ section
summarizes the calculated results about driver genes by 15
computational methods for a specic cancer type or dataset
and even provides three levels of biological interpretation
for realization of the relationships between driver genes.
Collin J. Tokeheim et al. compared eight algorithms
regarding overlap of the driver genes predicted by each
method, the discrepancy between the expected p-values and
the observed one, the number and consistencies predicting
driver genes, variability respectively in background
mutation number and in radiometric features, and
evaluating the evaluation of cancer driver genes. Although
these efforts have promoted the prediction of driver genes,
the accuracy remains to be increased.