没有合适的资源?快使用搜索试试~ 我知道了~
互信息(MI)是用于图像配准的一种流行的相似性度量,通过最大化联合直方图中簇的紧密度可以实现良好的配准。 但是,MI对出现在一个图像中的“物体异常”敏感,而对另一图像中的物体则不敏感,并且还遭受局部最大值和有偏最大值的影响。 我们提出了一种新颖的联合显着图(JSM)以突出显示两个图像中的相应显着结构,并着重将这些显着结构分组到加权联合直方图中的平滑紧实簇中。 该策略可以解决离群值和局部最大值问题。 实验结果表明,基于JSM-MI的算法不仅具有精确性,而且对于具有异常值的挑战性图像对的配准也具有鲁棒性。
资源推荐
资源详情
资源评论
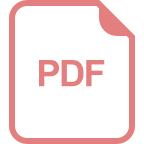
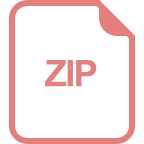
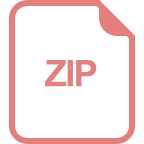
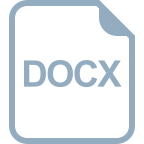
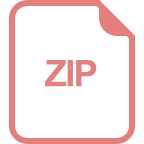
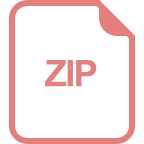
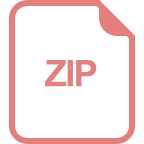
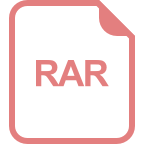
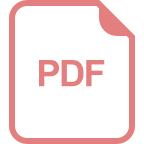
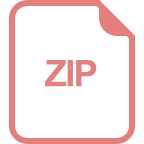
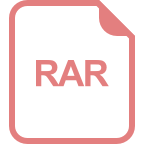
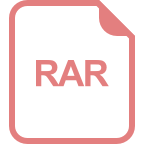
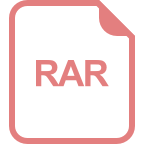
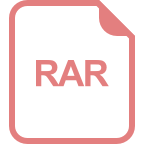
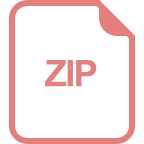
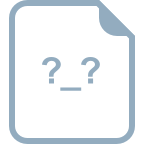
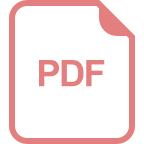
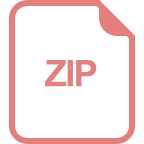
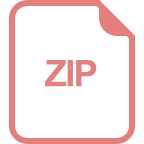
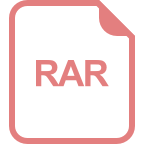
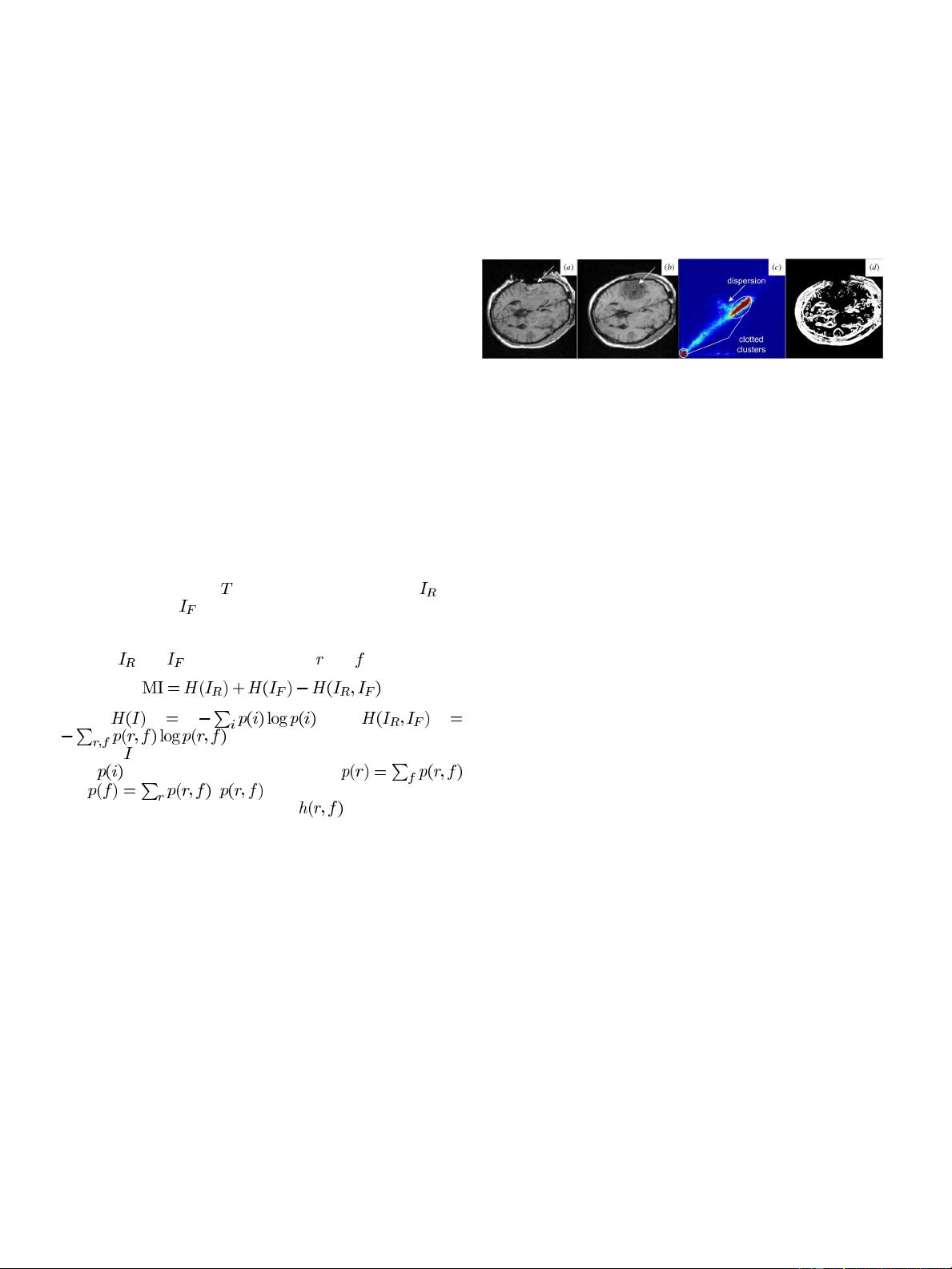
IEEE SIGNAL PROCESSING LETTERS, VOL. 17, NO. 1, JANUARY 2010 91
Registration of Images With Outliers
Using Joint Saliency Map
Binjie Qin, Member, IEEE, Zhijun Gu, Xianjun Sun, and Yisong Lv
Abstract—Mutual information (MI) is a popular similarity
measure for image registration, whereby good registration can
be achieved by maximizing the compactness of the clusters in the
joint histogram. However, MI is sensitive to the “outlier” objects
that appear in one image but not the other, and also suffers from
local and biased maxima. We propose a novel joint saliency map
(JSM) to highlight the corresponding salient structures in the
two images, and emphatically group those salient structures into
the smoothed compact clusters in the weighted joint histogram.
This strategy could solve both the outlier and the local maxima
problems. Experimental results show that the JSM-MI based
algorithm is not only accurate but also robust for registration of
challenging image pairs with outliers.
Index Terms—Image registration, joint saliency map, mutual in-
formation, outliers, weighted joint histogram.
I. INTRODUCTION
I
MAGE registration can be considered as finding the op-
timal transformation
between the reference image and
the floating image to maximize a defined similarity measure
such as mutual information (MI). Since 1995 [1], [2], MI has
been proved to be very effective in image registration. The MI
between
and (with intensity bins and ) is defined as:
(1)
where
and
are the entropy of the intensities
of image
and the entropy of the joint intensities of two im-
ages,
is the intensity probabilities with
and , is the joint intensity probabili-
ties estimated by the joint histogram
.
MI-based registration methods take advantage of the fact that
properly registered images usually correspond to compactly-
clustered joint histograms [3]. They measure the joint histogram
dispersion by computing the entropy of the joint intensity prob-
abilities. When the images become misregistered, the compact
Manuscript received August 08, 2009; revised September 27, 2009. First
published October 06, 2009; current version published October 28, 2009. This
work was supported in part by the NSFC (60872102), NBRPC (973 Program
2010CB834303), the Science Foundation of Shanghai Municipal Science &
Technology Commission (04JC14060), Shanghai Municipal Health Bureau
(2008115), and the Small Animal Imaging Project (06-545). The associate ed-
itor coordinating the review of this manuscript and approving it for publication
was Prof. H. Vicky Zhao.
B. Qin, Z. Gu, and X. Sun are with the Department of Biomedical Engi-
neering, School of Life Sciences & Biotechnology, Shanghai Jiao Tong Univer-
sity, Shanghai 200240, China (e-mail: bjqin@sjtu.edu.cn; gzj0126@gmail.com;
sxj_sun@sjtu.edu.cn).
Y. Lv is with the Department of Mathematics, Shanghai Jiao Tong University,
Shanghai 200240, China (e-mail: yslv@sjtu.edu.cn).
Color versions of one or more of the figures in this paper are available online
at http://ieeexplore.ieee.org.
Digital Object Identifier 10.1109/LSP.2009.2033728
Fig. 1. (a)–(b) Intra-operative and pre-operative MR image with a large tumor
resection. (c) Joint histogram dispersion with two clotted clusters (dark red in
pseudo color). (d) Joint saliency map for (a) and (b).
clusters become disperse sets of points in the joint histogram and
the entropy of the joint intensity probabilities increases. Making
no assumptions about the form of the intensity mapping between
the two images, MI is sensitive to the unmatchable outliers, e.g.
the tumor resection in the intra- and pre-operative brain im-
ages [see Fig. 1(a)–(b)]. To reject the outliers, some approaches
are proposed including consistency test [4], intensity transfor-
mation [5], gradient-based asymmetric multifeature MI [6] and
graph-based multifeature MI [7]. However, all these methods do
not emphasize the corresponding salient structures in the two
images to suppress the outliers. Furthermore, MI likely suffers
from local and biased maxima [8] which are caused by the am-
biguities in defining structure correspondence.
Spatial information, i.e. the dependence of the intensities of
neighboring pixels, has been included in MI [9]–[12] to im-
prove registration. Nevertheless, almost all MI-based methods
equally treat each overlapping pixel pair as a separate point in
the overlap area to calculate the joint histogram. This could
raise three issues: 1) when we equally consider the outlier
pixel pairs, the noncorresponding structures overlap and the
histogram will show certain clusters for the grey values of
the outliers. These clusters easily introduce the histogram
dispersion [see Fig. 1(c)] with increasing misregistration; 2)
while registration can be achieved by maximizing the com-
pactness of the histogram, the undesired clotted clusters [see
Fig. 1(c)] related to many noisy pixel pairs in the structureless
regions, such as background and white matter in the brain
image, increase the MI ambiguities and the local maxima [8]
(Fig. 5(c) shows that the normalized MI [1], [20] is in a biased
global maximum when the whole background areas in the two
endoscopic images are exactly aligned); 3) when we group
the intensity pairs as separate points into the histogram, the
independence of the neighboring bins could increase the MI
ambiguities and the local maxima. To solve this problem, joint
histogram smoothing (or blurring) [5], [8] has been used to
increase the dependence of the neighboring histogram bins. We
address these issues above as follows.
In fact, image registration is to match the corresponding
salient structures in both images. To suppress the outliers and
the homogeneous pixel pairs, the corresponding pixel pairs in
the corresponding salient structures should contribute more to
the joint histogram. For example, the corresponding salient
1070-9908/$26.00 © 2009 IEEE
Authorized licensed use limited to: Shanghai Jiao Tong University. Downloaded on November 6, 2009 at 23:03 from IEEE Xplore. Restrictions apply.
资源评论
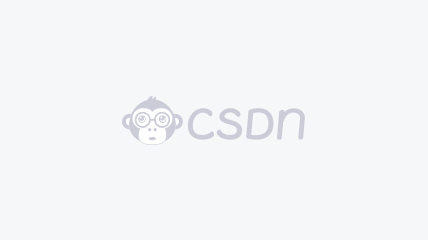

weixin_38720762
- 粉丝: 5
- 资源: 943
上传资源 快速赚钱
我的内容管理 展开
我的资源 快来上传第一个资源
我的收益
登录查看自己的收益我的积分 登录查看自己的积分
我的C币 登录后查看C币余额
我的收藏
我的下载
下载帮助

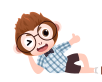
最新资源
- 精选毕设项目-优惠券卡卷小程序.zip
- 精选毕设项目-鱼缸表盘系统小程序.zip
- 精选毕设项目-阅享小程序(阅读评价类).zip
- 精选毕设项目-有住网(装修小程序).zip
- 精选毕设项目-悦读神器.zip
- 精选毕设项目-悦跑圈.zip
- 精选毕设项目-云文档.zip
- 精选毕设项目-运动荟小程序.zip
- 精选毕设项目-找电影视频小程序.zip
- 精选毕设项目-咫尺商圈多商家小程序.zip
- 精选毕设项目-智能用电.zip
- 精选毕设项目-装修预约小程序.zip
- 精选毕设项目-祝福话.zip
- FPGA 硬件电流环 基于FPGA的永磁同步伺服控制系统的设计,在FPGA实现了伺服电机的矢量控制 有坐标变,电流环,速度环,ad7606采样,电机正交编码器反馈接口,SVPWM,pi运算等等模块
- 数据库理论知识详解:涵盖模式结构、关系模型与MySQL数据定义语句
- 基于蒙特卡洛法的概率潮流 以IEEE33节点的电网为研究对象 建立了光伏和风电的概率出力模型 采用蒙特卡洛法进行随机抽样 之后基于抽样序列进行概率潮流计算 最后得到电网的电压概率出力曲线
资源上传下载、课程学习等过程中有任何疑问或建议,欢迎提出宝贵意见哦~我们会及时处理!
点击此处反馈


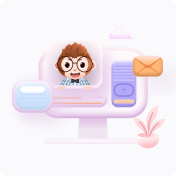
安全验证
文档复制为VIP权益,开通VIP直接复制
