没有合适的资源?快使用搜索试试~ 我知道了~
Placement Optimization for UAV-Enabled Wireless Networks with Mu...
1 下载量 102 浏览量
2021-01-20
03:53:47
上传
评论
收藏 324KB PDF 举报
温馨提示
Unmanned aerial vehicles (UAVs) have emerged as a promising solution to provide wireless data access for ground users in various applications (e. g. , in emergency situations). This paper considers a UAVenabled wireless network, in which multiple UAVs are deployed as aerial base stations to serve users distributed on the ground. Different from prior works that ignore UAVs’backhaul connections, we practically consider that these UAVs are connected to the core network through a ground gateway node
资源推荐
资源详情
资源评论
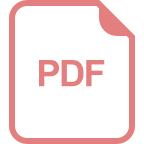
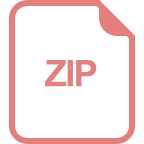
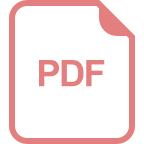
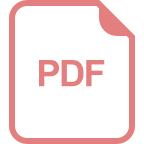
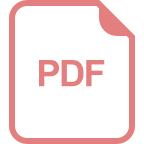
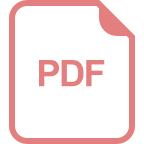
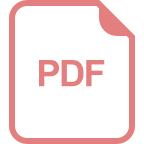
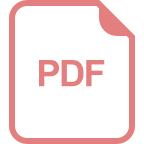
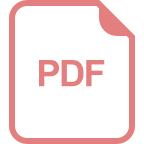
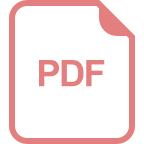
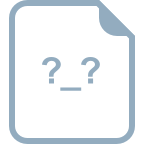
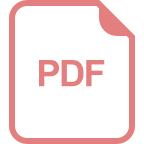
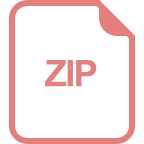
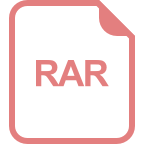
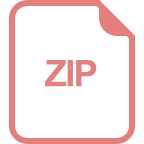
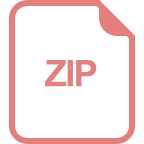
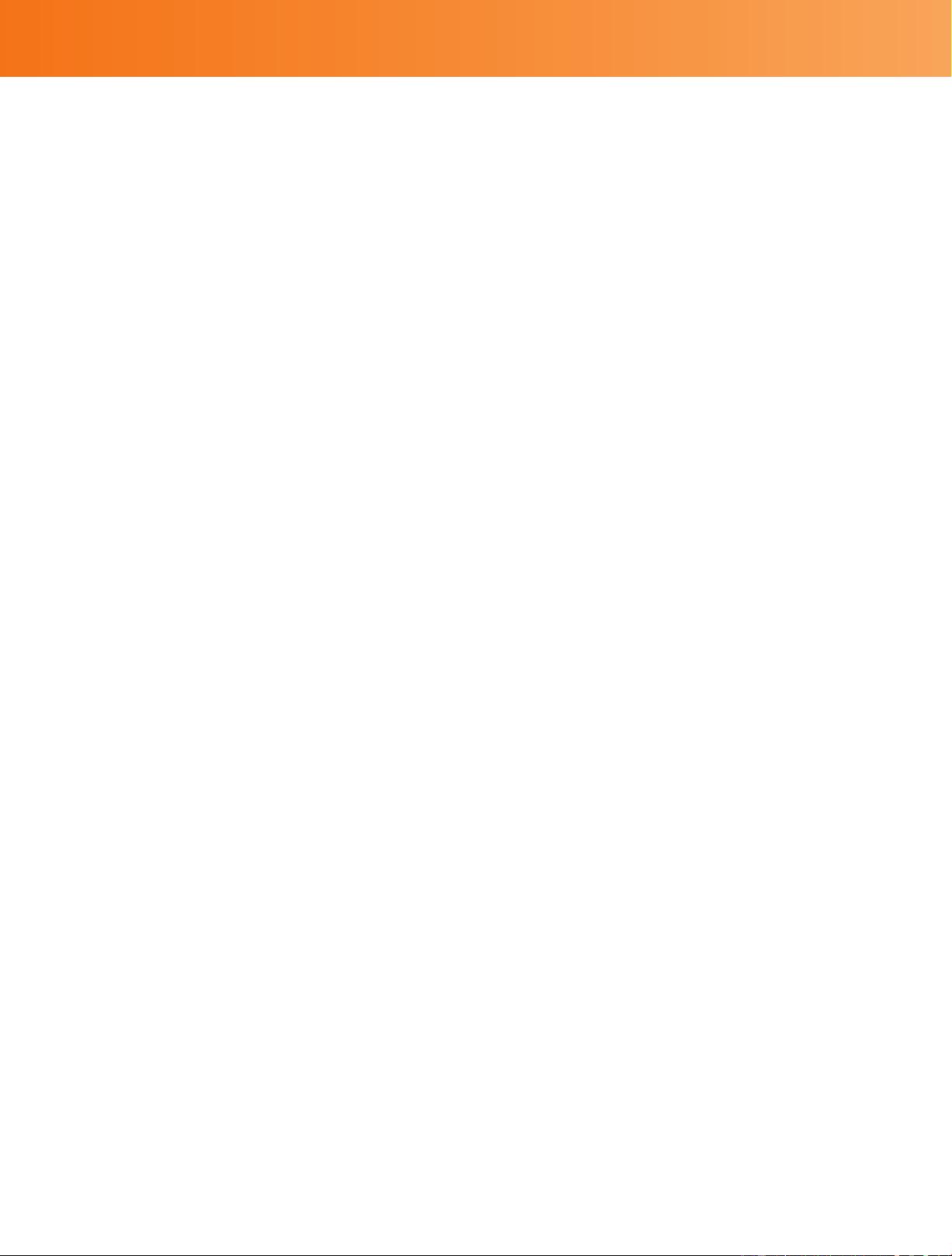
Journal of Communications and Information Networks, Vol.3, No.4, Dec. 2018
DOI: 10.1007/s41650-018-0040-3 Research paper
Placement Optimization for UAV-Enabled Wireless
Networks with Multi-Hop Backhauls
Peiming Li, Jie Xu
Abstract—Unmanned aerial vehicles (UAVs) have
emerged as a promising solution to provide wireless data
access for ground users in various applications (e.g.,
in emergency situations). This paper considers a UAV-
enabled wireless network, in which multiple UAVs are
deployed as aerial base stations to serve users distributed
on the ground. Different from prior works that ignore
UAVs’ backhaul connections, we practically consider that
these UAVs are connected to the core network through a
ground gateway node via rate-limited multi-hop wireless
backhauls. We also consider that the air-to-ground access
links from UAVs to users and the air-to-air backhaul links
among UAVs are operated over orthogonal frequency
bands. Under this setup, we aim to maximize the common
(or minimum) throughput among all the ground users
in the downlink of this network subject to the flow
conservation constraints at the UAVs, by optimizing the
UAVs’ deployment locations, jointly with the bandwidth
and power allocation of both the access and backhaul
links. However, the common throughput maximization
is a non-convex optimization problem that is difficult
to be solved optimally. To tackle this issue, we use the
techniques of alternating optimization and successive
convex programming to obtain a locally optimal solu-
tion. Numerical results show that the proposed design
significantly improves the common throughput among all
ground users as compared to other benchmark schemes.
Keywords—UAV, wireless networks, multi-hop back-
hauls, deployment optimization, bandwidth and power al-
location
Manuscript received Sept. 13, 2018; revised Nov. 30, 2018; accepted Dec.
10, 2018. This work was supported by the National Natural Science Founda-
tion of China (No. 61871137). The associate editor coordinating the review
of this paper and approving it for publication was W. Zhang.
P. M. Li, J. Xu. School of Information Engineering, Guangdong
University of Technology, Guangzhou 510006, China (e-mail: peimin-
glee@outlook.com; jiexu@gdut.edu.cn).
Part of this paper was presented at the IEEE International Conference on
Communication Systems (ICCS), Chengdu, China, Dec. 19-21, 2018
[1]
.
I. INTRODUCTION
U
nmanned aerial vehicles (UAVs), also known as (a.k.a.)
drones, have found a wide range of applications in, e.g.,
cargo delivery, aerial inspection, precision agriculture, and
traffic monitoring. Among others, employing UAVs as aerial
communication platforms to assist terrestrial wireless commu-
nications has recently emerged as one of the key technologies
for the fifth-generation (5G) cellular networks, which has at-
tracted a lot of interests from both academia and industry
[2,3]
.
On the one hand, UAVs can be used as aerial base stations
(BSs) to provide basic wireless data access for remote ar-
eas and in emergency situations (e.g., after natural disasters),
as well as to enhance the network capacity in terrestrial hot
spots
[4-8]
. On the other hand, UAVs can be utilized as aerial re-
lays to help far-apart ground users exchange information
[9,10]
,
and as access points in the sky for information dissemina-
tion and data collection with ground nodes (e.g., sensors and
actuators in Internet-of-Things networks)
[11]
. Besides wire-
less communications, UAVs can also serve as aerial plat-
forms for wireless power transfer (WPT)
[12,13]
, wireless pow-
ered communication networks (WPCN)
[14]
, and mobile edge
computing (MEC)
[15]
. In the industry, various companies
have launched their UAV-assisted wireless communication
projects, and some preliminary prototypes include Facebook’s
Aquila and Nokia’s flying-cell (F-Cell).
As compared to conventional terrestrial wireless communi-
cations, UAV-assisted wireless communications have the fol-
lowing advantages. First, UAV-enabled aerial communica-
tion platforms can be quickly deployed on demand, and thus
are cost-effective and suitable for emergency scenarios, e.g.,
when the terrestrial wireless infrastructures are damaged on
account of natural disasters. Next, the air-to-ground (A2G)
wireless channels between UAVs and ground nodes gener-
ally have much stronger line-of-sight (LoS) links than conven-
tional ground-to-ground (G2G) wireless channels; as a result,
the aerial BSs are expected to provide better wireless cover-
age and higher communication throughput than ground BSs.
Furthermore, due to the fully controllable mobility in three-
dimensional (3D) airspace, UAVs can adaptively change their
locations over time for reducing the distances with intended
ground users, so as to further improve the communication per-
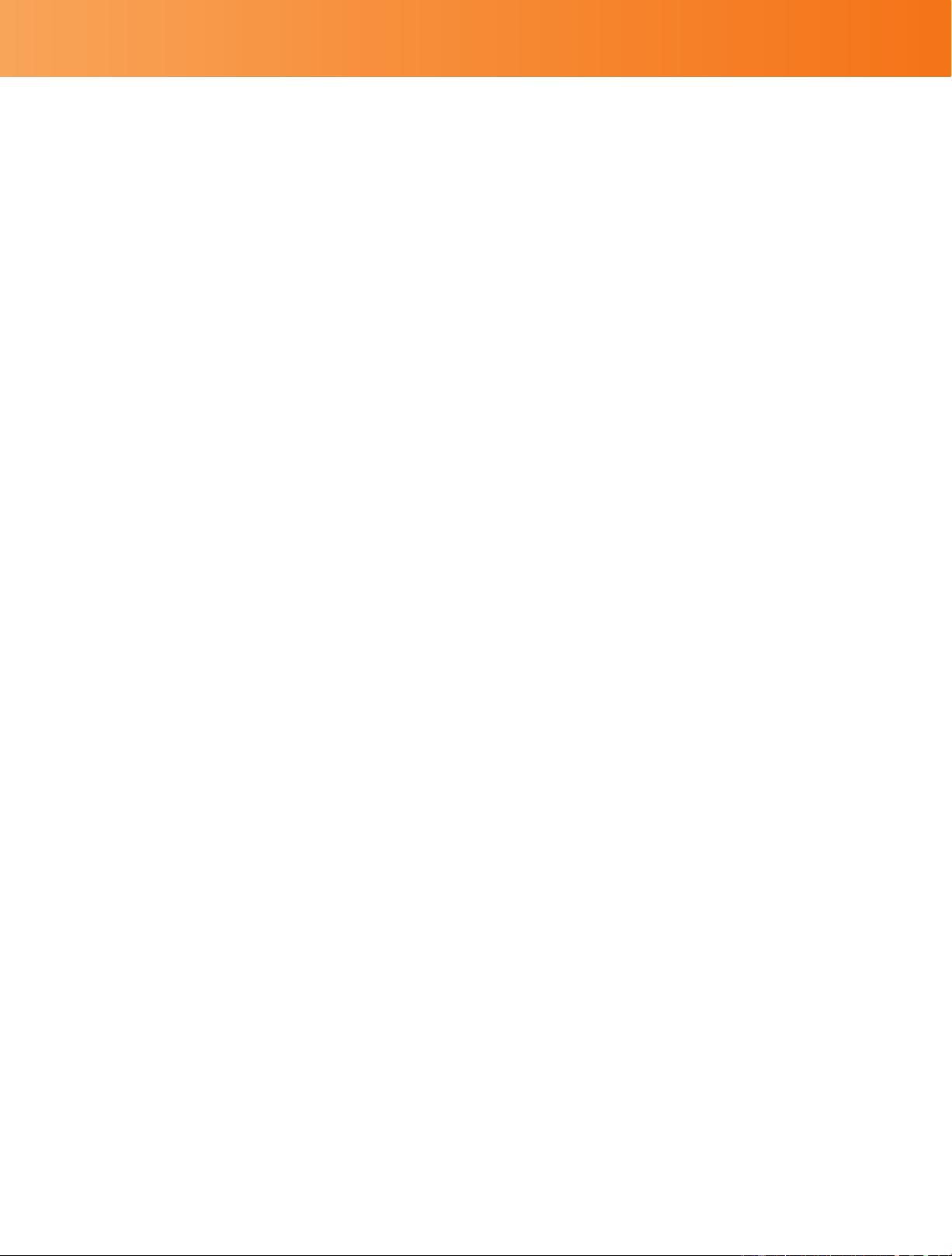
Placement Optimization for UAV-Enabled Wireless Networks with Multi-Hop Backhauls
65
formance.
In general, UAV-enabled aerial wireless communication
platforms can be classified into two categories depending on
whether UAVs are quasi-stationary or fully mobile. For quasi-
stationary UAVs, prior works [5-8,10,16,17] focused on the
optimization of their deployment locations for communication
performance improvement. For instance, Refs. [7] and [16]
optimized the two-dimensional (2D)/3D placement of UAVs
to maximize the network revenue (i.e., the number of users
served by UAVs under given quality-of-service requirement
at each user) and minimize the number of UAVs for maintain-
ing wireless coverage, respectively. The authors in Ref. [8]
optimized the UAVs’ flying altitude to maximize the com-
munication throughput for multiuser unicasting and multicas-
ting communications by considering directional antennas at
UAVs with adjustable beamwidth. Moreover, Refs. [5] and [6]
analyzed the average performance of quasi-stationary UAV-
enabled wireless networks via the stochastic geometry the-
ory. For fully mobile UAVs, existing works [4,18,21] pro-
posed to dynamically control their locations over time, a.k.a.
trajectories, to improve the communication rates with differ-
ent users along with time. For instance, Refs. [18] and [21]
studied the UAV-enabled mobile relaying networks, where a
UAV-mounted relay node can adaptively control its trajectory
jointly with the wireless resource allocation to maximize the
end-to-end communication rate from the source node to the
destination node. In Ref. [4], the authors proposed a new
cyclical multiple access scheme that schedules the multiuser
communication based on the UAV’s trajectory, in which an
interesting throughput-delay tradeoff is revealed. The authors
in Refs. [10] and [17] used the machine learning techniques to
construct radio maps for A2G wireless channels, and accord-
ingly optimized the UAVs’ trajectory for communication rate
maximization. Furthermore, Refs. [12] and [13] optimized
the UAV’s trajectory for maximizing the energy transfer effi-
ciency towards multiple ground nodes in a UAV-enabled WPT
system. It is worth noting that both quasi-stationary and mo-
bile UAVs have advantages and disadvantages. In particular,
mobile UAVs can exploit the UAVs’ fully-controllable mobil-
ity to achieve higher communication performance than quasi-
stationary UAVs, but they need more sophisticated trajectory
control over time with non-causal information (e.g., channel
state information) required in general. By contrast, quasi-
stationary UAVs only need to a priori determine the UAVs’
deployment locations over a certain period of time, and thus
may have much lower complexity for practical implementa-
tion. In this paper, we focus our study on the deployment op-
timization problem for quasi-stationary UAV-enabled wireless
networks.
In particular, this paper considers a scenario when multiple
UAVs are employed as quasi-stationary aerial BSs to serve
multiple users distributed on the ground. In practice, this net-
work faces various technical challenges. For instance, how to
provide UAVs with reliable backhaul connections to the core
network is a challenging task to be tackled, as UAVs in the sky
are difficult to have wireline backhauls as conventional terres-
trial BSs. In the literature, although there are some prior works
studying the UAV deployment optimization in UAV-enabled
wireless networks (e.g., Refs. [5-8,10,16,17]), they only fo-
cused on the A2G access links between UAVs and ground
users by ignoring backhaul connections. This thus motivates
our investigation in this work to fill such a research gap.
We consider a UAV-enabled wireless network where dif-
ferent UAVs are connected via wireless multi-hop backhauls
with the core network. Under this setup, we aim to optimize
the UAVs’ deployment locations for network performance op-
timization, subject to rate-constrained wireless backhauls. We
suppose that the A2G access links from UAVs to users and
the air-to-air (A2A) backhaul links among UAVs are operated
over orthogonal frequency bands to avoid severe co-channel
interference. Due to the multi-hop connection, each UAV is
subject to the so-called flow conservation constraints, i.e., the
total outgoing flow from each UAV (to ground users through
A2G links or to other UAVs through A2A links) should be no
larger than the total incoming flow to that UAV (from the gate-
way node or from other UAVs). In this case, the communica-
tion rates of those ground users are fundamentally constrained
by the limited rates of A2A backhaul links. Therefore, it is
crucial to properly balance the rate tradeoff between the A2G
access links versus the A2A backhaul links via efficient joint
UAV deployment optimization and wireless resource alloca-
tion.
In particular, we maximize the common (or minimum)
throughput among all ground users in the downlink of this net-
work, by jointly optimizing the UAVs’ deployment locations
and the transmit power and bandwidth allocation in both A2A
backhaul and A2G access links, subject to the individual trans-
mit power constraints and the flow conservation constraints at
the UAVs, as well as the total bandwidth constraints. How-
ever, as the flow conservation constraints are non-convex, the
common throughput maximization is a non-convex optimiza-
tion problem that is generally difficult to be solved optimally.
To tackle this issue, we employ the techniques of alternat-
ing optimization and successive convex programming (SCP)
to optimize the UAVs’ deployment locations and the wire-
less resource allocation in an alternating manner for obtaining
a locally optimal solution. Numerical results show that the
proposed design significantly improves the common through-
put among all ground users as compared to other benchmark
schemes.
Notice that the UAV deployment optimization problem
with wireless backhaul constraints has not been investigated
in the literature yet. To our best knowledge, there is only one
related work
[22]
that used UAVs to provide multi-hop wire-
剩余9页未读,继续阅读
资源评论
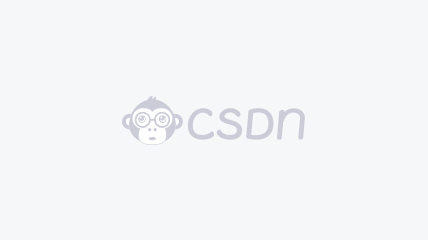

weixin_38714370
- 粉丝: 2
- 资源: 905
上传资源 快速赚钱
我的内容管理 展开
我的资源 快来上传第一个资源
我的收益
登录查看自己的收益我的积分 登录查看自己的积分
我的C币 登录后查看C币余额
我的收藏
我的下载
下载帮助

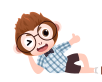
最新资源
资源上传下载、课程学习等过程中有任何疑问或建议,欢迎提出宝贵意见哦~我们会及时处理!
点击此处反馈


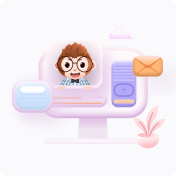
安全验证
文档复制为VIP权益,开通VIP直接复制
