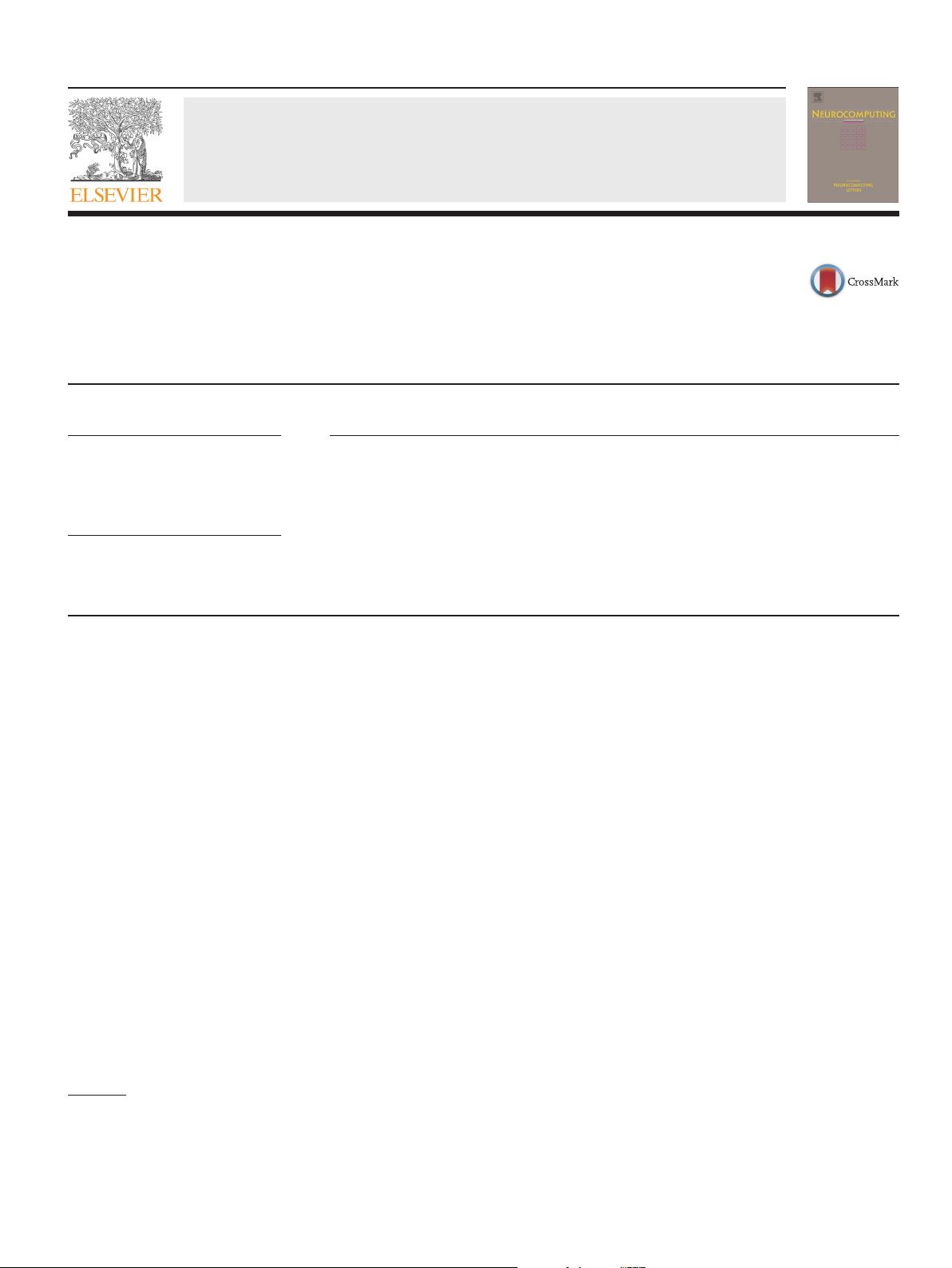
Short communication
Improved passivity analysis for neural networks with Markovian
jumping parameters and interval time-varying delays
Guoliang Chen, Jianwei Xia
n
, Guangming Zhuang
School of Mathematics Science, Liaocheng University, Liaocheng 252000, PR China
article info
Article history:
Received 4 October 2014
Received in revised form
24 November 2014
Accepted 13 December 2014
Communicated by Hongyi Li
Available online 20 December 2014
Keywords:
Neural networks
Passivity
Interval time-varying delays
Markovian jumping parameters
abstract
The problem of passivity analysis for neural networks with Markovian jumping parameters and interval
time-varying delays is investigated in this paper. By constructing a novel Lyapunov–Krasovskii functional
based on the complete delay-decomposing idea and using reciprocally convex technique, some
improved delay-dependent passivity criteria are established in terms of linear matrix inequalities.
Numerical examples are also given to show the effectiveness of the proposed methods.
& 2014 Elsevier B.V. All rights reserved.
1. Introduction
The problem of neural networks has been widely investigat ed in
the last few decades due to their potential applications in many areas
such as pattern recognition, static imag e processing, associative mem-
ory and combinatorial optimization [1,2]. It is known that stability is
one of the most important properties for the designed neural net-
works. Meanwhile, time delays are inevitable encountered in many
practical systems and usually the main reason resulting in instability,
therefore, increasing attention has been focused on the problem of
stability analysis for neural networks with time delays, and a great deal
of related delay-independent and delay-dependent criteria have been
reported in recent liter ature, see, e.g., [3–13] and the references therein.
Furthermore, as a powerful t ool for analyzing the stability of systems,
passivity theory has also considerable backgroun ds in man y control
fields, for example, chaos control and synchronizatio n [14], fuzzy
control [15] and signal processing [1 6]. Naturally , increasing att ention
has been focused on the passivity analysis for neural networks with
time-vary ing delay s [17–21] in recent years.
On the other hand, as an important kind of hybrid systems, Mar-
kovian jump sy stems were firstly introduced by Krasovskii and Lidskii
in [22]. These class systems have significant adv antage in modeling
man y practical dynamic systems, such as manufacturing systems, net-
worked control systems, economics systems, and fault-tolerant control
systems. And a great of work has been report ed for Markovian jumping
systems in recent literature [23,24]. Especially , a novel fault-tolerant
control method for Markovian jump stochastic systems was introduced
in [24] and the results can be well applied to the real application
syst ems. At the same time, consider able effort has been focused on the
dynamics analysis for neural networks with Markovian jump para-
meters in recent years, and many research results have been proposed
in this brief. For example, the problem of stability analysis and passivity
analysis has been investigated in [25–31] and [32–36] for different
neural networks with Markovian jump parameters and time delays,
respectiv ely . It is worth mentioning that, although there are already
many works to deal with the problem of dynamic analysis to those
neural networks, they are still conservati ve to some e xtent, for exa-
mple, the techniq ue to deal with the cr oss products in most of those
works was Jensen inequality , it will lead to some conservativeness of
the achieved results, this motivates us to carry out this work.
In this paper , our researc h efforts are focused on developing a new
approach t o analyze the passivity of neural networ ks with Markovian
jumping parameters and interv al time-varying delays. T o obtain
impro ved delay-depend ent sufficient conditions for the mentioned
systems, novel Lyapunov–Krasovskii functionals based on the idea of
decomposing the delays in all integral terms and the reciprocally
conve x approach dealing with the integral cross terms are introduced.
At last, numerical ex amples are provided to show the effectiveness and
the less conserv atism of the obtained passivity criteria.
Notation: Throughout this paper, for symmetric matrices X and
Y, the notation X Z Y (respectively, X 4 Y
) means that the matrix
X Y is positive semi-definite (respectively, positive definite); I is
the identity matrix with appropriate dimension; M
T
represents the
transpose of the matrix M; R
n
denotes the n-dimensional Euclidean
Contents lists available at ScienceDirect
journal h omepage: www.el sevier.com/locate/neu com
Neurocomputing
http://dx.doi.org/10.1016/j.neucom.2014.12.023
0925-2312/& 2014 Elsevier B.V. All rights reserved.
n
Corresponding author.
E-mail addresses: Chenguoliang3936@126.com (G. Chen),
njustxjw@126.com (J. Xia), zgmtsg@126.com (G. Zhuang).
Neurocomputing 155 (2015) 253–260