时滞随机奇异模糊系统的H-∞滤波
198 浏览量
2021-03-19
18:34:58
上传
评论
收藏 539KB PDF 举报

weixin_38690508
- 粉丝: 5
- 资源: 925
最新资源
- MyBatisCodeHelperPro 3.3.2-2322 2023.2-2024.1
- 基于Python的简单的学生成绩管理程序设计(课程设计)
- jdk-8u20-windows-x64安装版本-jdk-8u301-linux-x64解压版
- 植物大战僵尸杂交版 修改阳光和冷却
- html css js网页设计ntion-model-for-开发笔记
- 数据库课程设计-processing开发笔记
- matlab画图sic-开发demo
- 数据库原理课程设计-图书馆数据库管理系统
- IEC101Sim IEC870-5-101协议仿真主站、子站 (含源码 C++Builder)
- 2212503007陈熙炜跨境2202作业2.docx
资源上传下载、课程学习等过程中有任何疑问或建议,欢迎提出宝贵意见哦~我们会及时处理!
点击此处反馈


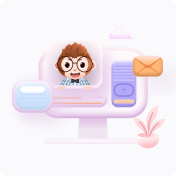