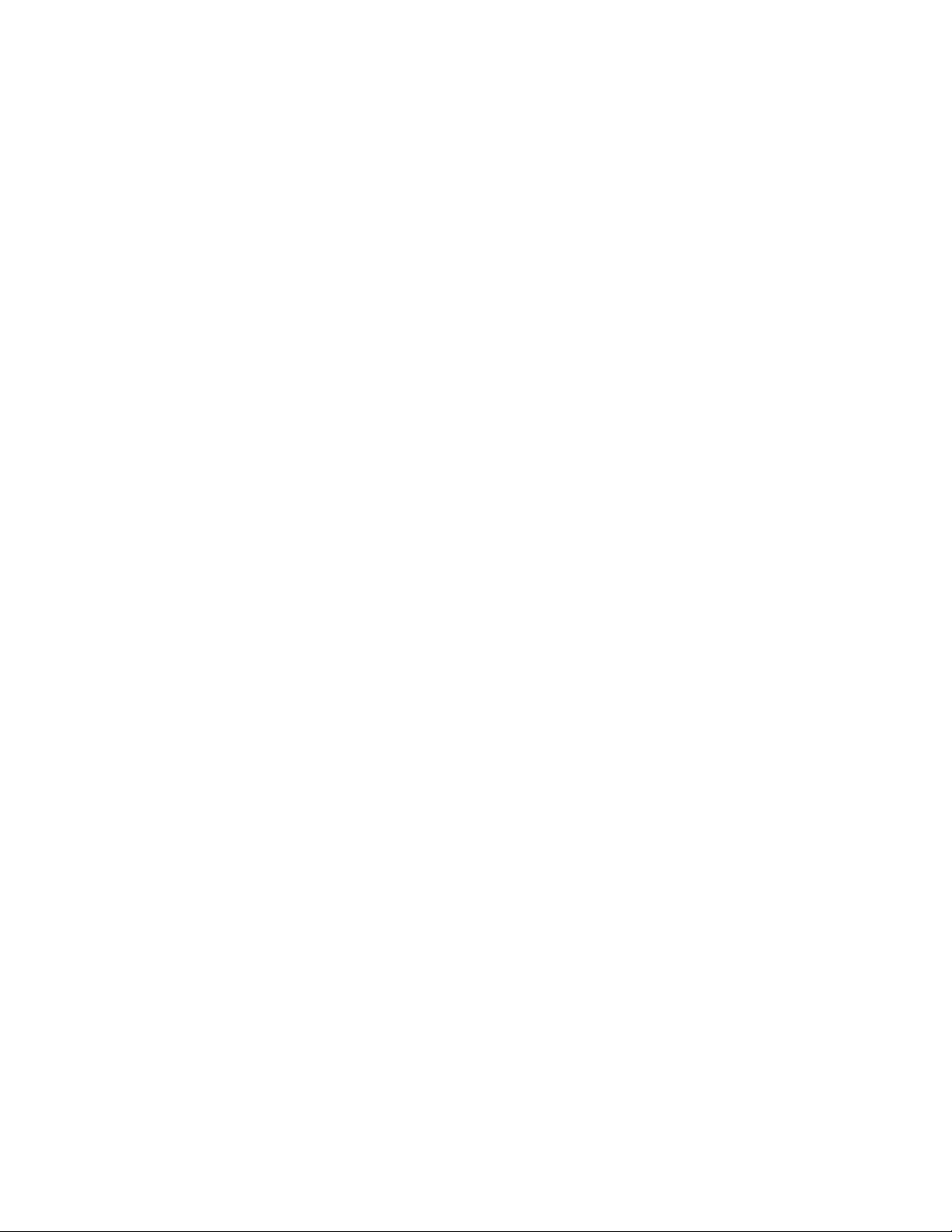
Sparse Channel Estimation Based on Compressed
Sensing for Massive MIMO Systems
Chenhao Qi, Yongming Huang, Shi Jin and Lenan Wu
School of Information Science and Engineering, Southeast University, Nanjing 210096, China
Email: qch@seu.edu.cn
Abstract—The sparse channel estimation which sufficiently
exploits the inherent sparsity of wireless channels, is capable
of improving the channel estimation performance with less pilot
overhead. To reduce the pilot overhead in massive MIMO system-
s, sparse channel estimation exploring the joint channel sparsity
is first proposed, where the channel estimation is modeled as
a joint sparse recovery problem. Then the block coherence of
MIMO channels is analyzed for the proposed model, which shows
that as the number of antennas at the base station grows, the
probability of joint recovery of the positions of nonzero chan-
nel entries will increase. Furthermore, an improved algorithm
named block optimized orthogonal matching pursuit (BOOMP)
is also proposed to obtain an accurate channel estimate for the
model. Simulation results verify our analysis and show that the
proposed scheme exploring joint channel sparsity substantially
outperforms the existing methods using individual sparse channel
estimation.
Index Terms—Compressed sensing (CS); sparse channel esti-
mation; massive MIMO; large-scale MIMO;
I. Introduction
In order to improve the data rate as well as the reliability
of wireless systems, the multi-antenna technology, termed as
multiple-input multiple-output (MIMO), has been extensively
investigated in the last two decades. In a typical multi-user
MIMO system, a base station (BS) equipped with some
antennas simultaneously communicates with several users each
equipped with a single antenna. Recently, it has been shown
that as the number of BS antennas grows to be infinity, the
effect of additive noise and rayleigh fading is negligible and
a very high data rate can be achieved [1]. Therefore, we may
construct a massive MIMO or large-scale MIMO system by
equipping the BS with orders of magnitude more antennas,
e.g., 128, which is even larger than the number of users that
the BS serves [2]. In this way, the BS can sufficiently exploit
the spatial degree of freedom to simultaneously communicate
with several users using the same temporal and frequency
resource [3]. The researchers from Rice University establish
a BS equipped with 64 antennas serving 15 users, which is
demonstrated to achieve up to 6.7 fold capacity gains while
using a mere 1/64th of transmission power [4].
One of the challenges in massive MIMO systems is the pilot
overhead that grows linearly with the number of channels to be
estimated. In massive MIMO systems, the number of wireless
links and channels is very large, leading to the proliferation
of pilot overhead and thus the reduced resource for data. To
reduce the pilot overhead, one potential choice is to explore
the inherent sparsity of wireless channels and to use the sparse
channel estimation [5], [6], [7]. Wireless channel is essentially
sparse, where only a small number of channel coefficients are
nonzero. By applying recently emerged compressed sensing
(CS) technique, sparse channel estimation can be used to
estimate the channel impulse response (CIR) based on the
received and transmitted pilot symbols. Compared to the
least squares (LS) and minimum mean square error (MMSE)
methods, sparse channel estimation is capable of improving
the channel estimation performance and reducing the pilot
overhead [8], [9], [10], [11]. In [5], distributed compressive
channel estimation and feedback schemes are considered for
frequency-division duplex (FDD) massive MIMO Systems.
In [12], superimposed pilot design for downlink FDD massive
MIMO systems is proposed based on structured CS. In [13],
sparse channel estimation with structured CS is proposed for
multi-input single-output (MISO) systems. In [14], based on
the idea that the degree of freedom of the channel matrix
is smaller than the number of free parameters, a low-rank
matrix approximation is proposed and solved via semidefi-
nite programming (SDP). In [15], uplink channel estimation
exploring joint channel sparsity is investigated for massive
MIMO systems.
It is shown in [16] that the CIR from different BS antennas
to the same user antenna shares a common support, because
the time of arrival (ToA) is similar while the paths amplitudes
and phases are distinct. In other words, the nonzero positions
of different CIRs are the same, exhibiting the joint sparsity.
So it is beneficial to exploit the joint sparsity so that the
number of pilots for channel estimation can be substantially
reduced. In this paper, we first consider the sparse channel
estimation exploring the joint channel sparsity, where the
channel estimation is modeled as a joint sparse recovery
problem. Then the block coherence of MIMO channels is
analyzed for the proposed model, which shows that as the
number of BS antennas grows, the probability of joint recovery
of the positions of nonzero channel entries will increase.
Furthermore, an algorithm named block optimized orthogonal
matching pursuit (BOOMP) is also proposed to obtain a
reliable solution to this model.
The remainder of this paper is organized as follows. Sec-
tion II provides the system model for sparse channel es-
timation exploiting joint sparsity, which is then formulated
as a joint sparse recovery problem. Section III analyzes the
block coherence for the proposed model. In Section IV, an
algorithm named BOOMP is proposed to get a solution to the
IEEE ICC 2015 - Signal Processing for Communications Symposium
978-1-4673-6432-4/15/$31.00 ©2015 IEEE 4558