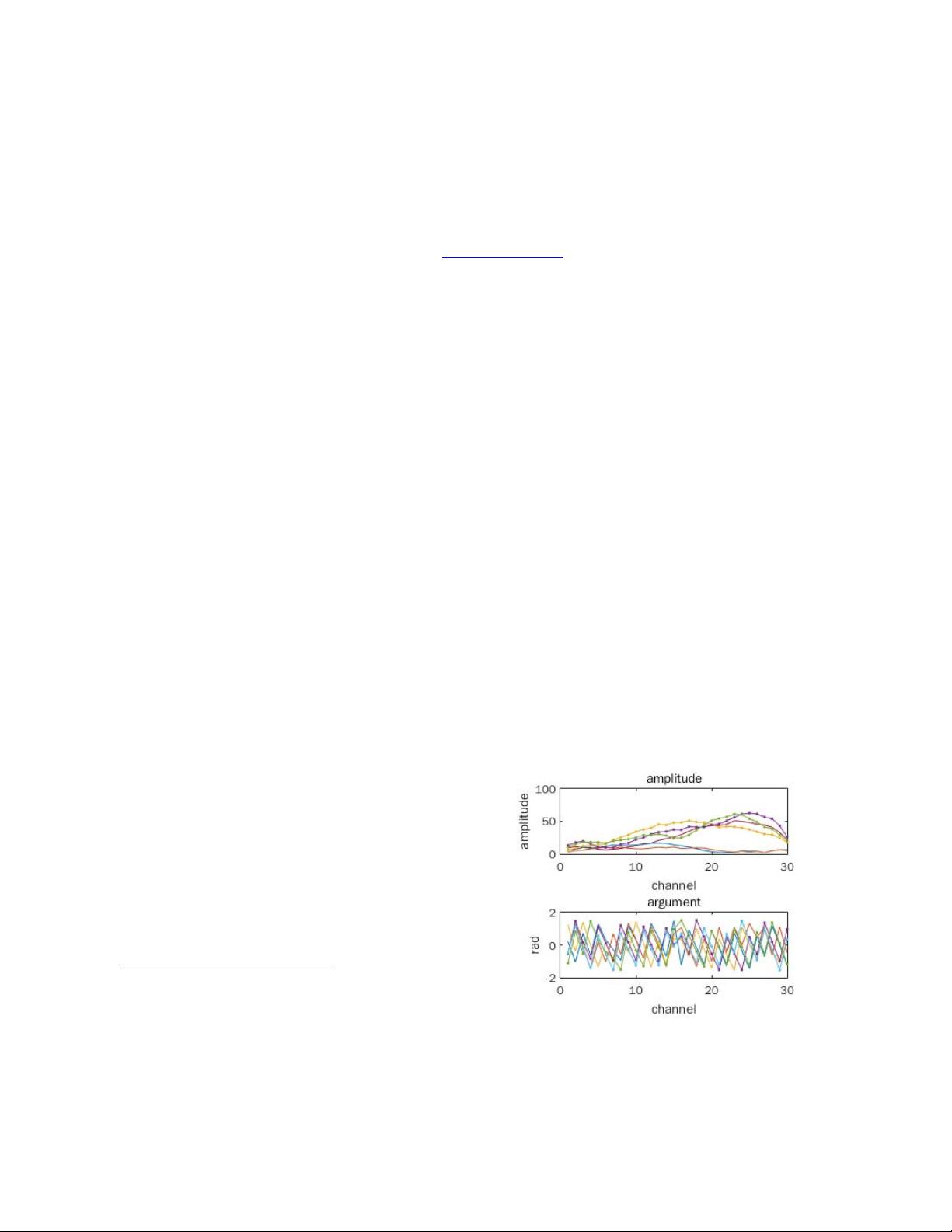
1
INTRODUCTION
Localization is becoming increasingly important in daily
life, for location-based services can be one of the most
indispensable techniques for mobile applications. With the
popularity of lots of new techniques such as somatosensory
game and home automation, a good plan for indoor
localization calls an emergency. Unfortunately, there are
few plans that concentrate on indoor localization, and most
of those are not good enough to come into the public.
For example, the Global Positioning System (GPS) does
not perform effectively in indoor environment because it is
hard for GPS signals to penetrate buildings, besides, most
of current systems such as GPS system, infrared, ultrasonic
and radio frequency (RF) share an identical requirement
that special hardware must be carried such as smart phone
or radio-frequency identification (RFID) [1]. Nevertheless,
such device-based systems are deficient in satisfying
people’s growing demands. Therefore, device-free indoor
localization problem has been drawing much attention from
a large range.
The majority of previous localization approaches are based
on received signal strength indicator (RSSI), which
measures the radio frequency (RF) signal strength at the
receiver. It is able to detect the position of object in the
This work is supported by National Natural Science Foundation of
China (No. 61273078, 61471110), China Postdoctoral Science Special
Foundation (No.2014T70263), China Postdoctoral Science Foundation
(No.2012M511164), Chinese Universities Scientific Foundation
(N13040402 3, N110804004, N140404014), Liaoning Doctoral Startup
Foundation (No.20121004), Foundation of Liaoning Educational
Commission (L2014090).
interested area by distinctive changes instead of directly
localizing the tracked object with the carrying hardware.
And it is easy to calculate the distance between the signal
emitter and the receiver according to propagation loss
model. From the comparison between RSSI and the
approaches mentioned above, we can see that RSSI has
already overcome the above difficulties.
But nowadays people argue that there are two significant
reasons why RSSI is not appropriate for indoor localization.
First, it is hard to acquire accurate RSSI value for per
packet and RSSI may fluctuate inherently which will
reduce sensitivity to environmental change caused by
object. Second, RSSI is vulnerable to multipath effect
which creates inestimable errors.
Channel State Information
Fig 1.Channel State Information, it includes huge information including
intensity and phase, so it’s better than RSSI.
CSI-based Autoencoder Classification for Wi-Fi Indoor Localization
Chengcheng Xu
1
, Zixi Jia
1*
, Pan Chen
1
, Bo Wang
2
1. College of Information Science and Engineering
2. School of Metallurgy
Northeastern University, NO. 3-11 Wenhua Road Heping District, Shenyang 110819, China
* Corresponding author
E-mail: jiazixi@ise.neu.edu.cn
Abstract: Recently, indoor localization problem has drawn a wide range of attention. However, there are few researches
that can keep balance between accuracy and expense, and few plans can achieve both device-free and accuracy. To solve
this problem, the scheme of CSI-based autoencoder classification for Wi-Fi indoor localization is proposed. Only one
wireless router and one computer are placed as signal emitter and receiver respectively. With so few ordinary devices,
expenses have been decreased to a large extent. Device-free is achieved by performing localization based on Wi-Fi
signal. Channel State Information (CSI) is measured and calculated to decrease the multipath effect, which reaches a
higher accuracy. With the use of CSI, a mass of data are obtained. Machine learning including autoencoder and BP
network are utilized owing to their advantage of processing mass data. In our experiment, this plan achieves 2-dim
localizing with an accuracy of 50 cm.
Key Words: Channel State Information, Indoor Localization, Autoencoder, Classification, Machine Learning
6523
978-1-4673-9714-8/16/$31.00
c
2016 IEEE