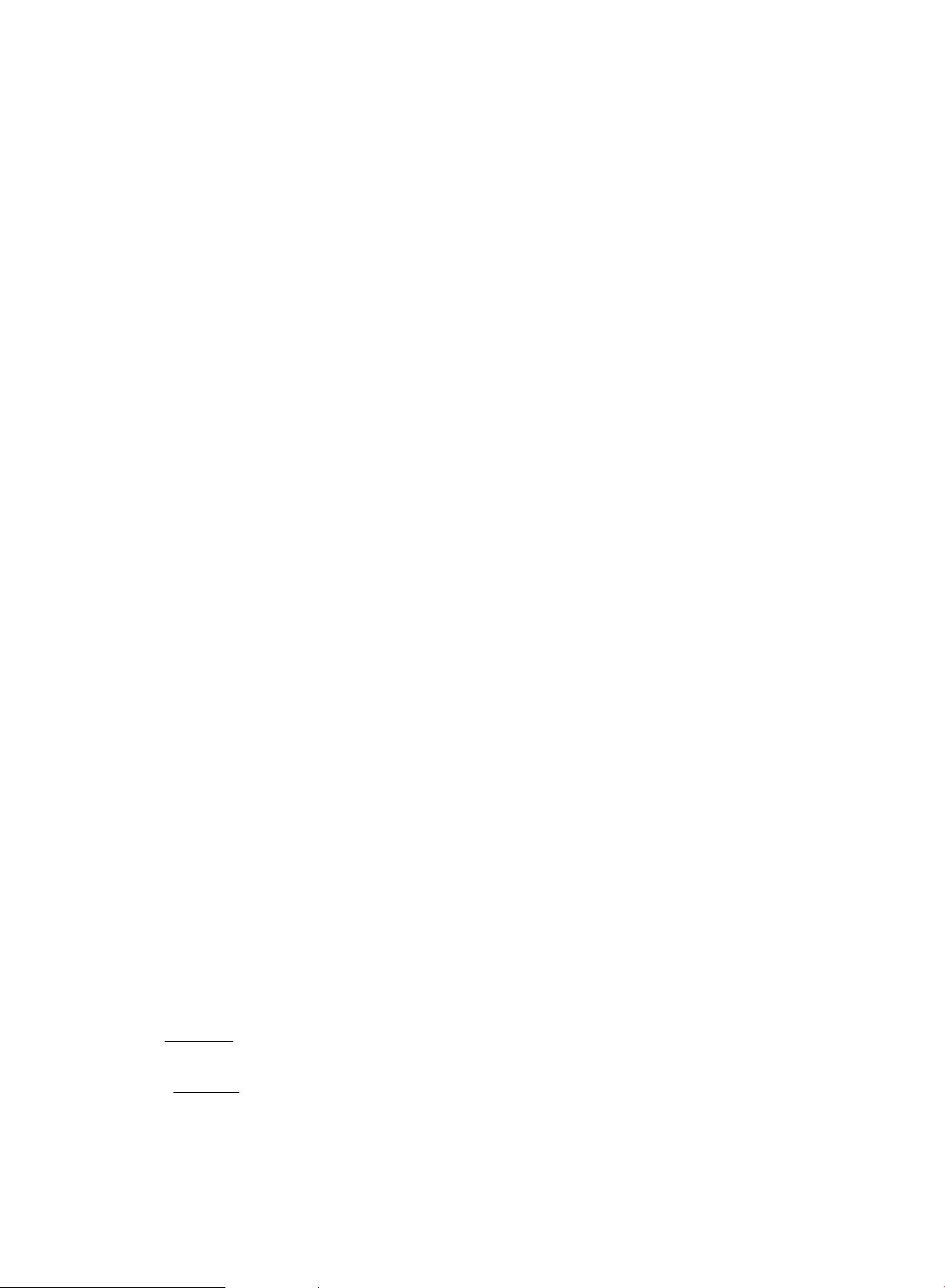
profits) of DMUs. In addition, since different subunits of a same organization are homogeneous and comparable [2], the
requirement for homogeneity of DMUs can be met in applying DEA to the cost or revenue allocation problem [3]. More-
over, since the DEA technique provides the possibility of considering the effect of feasible allocation plans on perfor-
mance evaluation based on the empirical characterization of a production possibility set [3], it has advantages over
other methods. Though DEA is very equitable to solve such allocation problems, up until now, there are few relevant
works from a DEA viewpoint. Based on two principles of efficiency invariance (a cost allocation satisfies the efficiency
invariance principle if the relative efficiency of each DMU remains unchanged after allocation) and input pareto-mini-
mality (a cost allocation is input pareto-minimal if no cost can be transferred from one DMU to another without violat-
ing the efficiency invariance principle), Cook and Kress [2] made the first attempt of designing a DEA-based approach to
fixed cost allocation. However, Jahanshahloo et al. [4] used a numerical example to show that the input pareto-minimal-
ity principle in Cook and Kress’s approach [2] had been violated, and provided a method in which without solving linear
programming problems only using simple formula, a fixed cost allocation can be achieved. Whereas, we will prove that
Jahanshahloo et al.’s method is short of practical acceptability in many applications. A similar shortcoming also exists in
the common revenue allocation method presented in [5]. Besides, in their revenue allocation method there still exist
other shortcomings which will be described later. Considering Cook and Kress’s approach only can be directly used to
determine the cost allocation in the case of single input and single output, Cook and Zhu [6] extended the approach
in [2] and provided a feasible (but not optimal) cost allocation in the general case of multiple inputs and multiple out-
puts. For Cook and Zhu’s approach probably has no feasible solution when some special constraints are attached in
application, Lin [7] put forward a new fixed cost allocation via improving their approach. On the other hand, Beasley
[8] provided a nonlinear DEA-based cost allocation by means of maximizing the average efficiency across all DMUs
and adding additional constraints to obtain a unique solution. By way of combining the efficiency invariance principle
of Cook and Kress [2] and the additional constraints of Beasley [8], Amirteimoori and Kordrostami [9] proposed an ap-
proach to get a unique allocation. Li et al. [3] treated the cost allocated to each DMU as a supplemental cost input and
designed an alternative DEA-based approach. Above allocation models, focusing on different key points, are applicable to
different application backgrounds accordingly. Decision makers can select an appropriate fixed cost allocation model
according to the specific application background.
This paper mainly aims to design new approaches to fixed cost allocation and common revenue allocation. We organize
the rest of this paper as follows. Section 2 briefly introduces the DEA theory and the allocation methods presented in [4,5] as
well as the shortcomings of the latter’s. Our DEA-based approaches for allocating fixed costs and common revenue are de-
scribed in Section 3. A numerical example is solved in Section 4. Conclusions and directions for future research are presented
in Section 5.
2. Background
Suppose that an organization has fixed costs or common revenue and it desires to allocate a proportion of this fixed
cost or common revenue to each subunit in an appropriate manner. This problem frequently occurs in any organization,
namely to split fixed costs or common revenue among various subunits. In this section we first introduce the output-ori-
ented CCR model and Jahanshahloo et al.’s fixed cost allocation method together with its shortcomings, and then give a
description of the input-oriented CCR model and Jahanshahloo et al.’s common revenue allocation method with its
defects.
2.1. Jahanshahloo et al.’s cost allocation method
Characterized by a multiple outputs and/or multiple inputs structure, the DEA technique is a widely used optimization
tool to measure the relative efficiencies of DMUs. Suppose that there is a set of n DMUs, j =1,...,n. For each DMU, there
are t outputs and m inputs. Let y
rj
(r =1,..., t) be the rth known output of DMU j,x
ij
(i =1,...,m) be the ith known input of
DMU j. The output-oriented DEA efficiency measure for a DMU is given by the ratio of weighted sum of inputs to weighted
sum of outputs. To measure the DEA efficiency of a target DMU p (p =1,...,n), the following output-oriented CCR model
needs to be solved.
min
f
v
ip
;u
rp
g
P
m
i¼1
v
ip
x
ip
P
t
r¼1
u
rp
y
rp
s:t:
P
m
i¼1
v
ip
x
ij
P
t
r¼1
u
rp
y
rj
P 1; j ¼ 1; ...; n;
u
rp
P 0; r ¼ 1; ...; t;
v
ip
P 0; i ¼ 1; ...; m;
ð1Þ
R. Lin / Applied Mathematics and Computation 218 (2011) 3680–3688
3681