没有合适的资源?快使用搜索试试~ 我知道了~
温馨提示
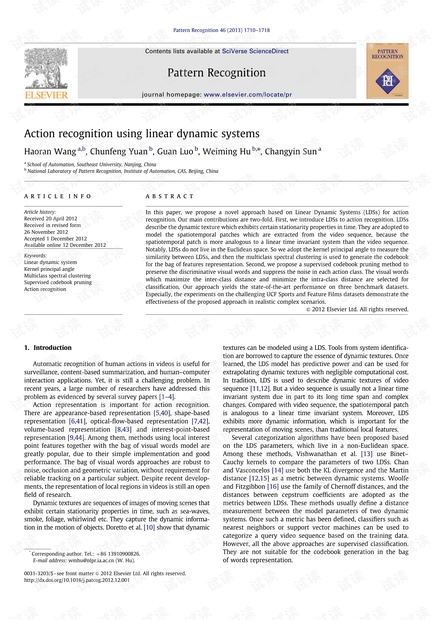

试读
9页
在本文中,我们提出了一种基于线性动态系统(LDS)的动作识别新方法。我们的主要贡献是双重的。首先,我们将LDS引入到动作识别中。 LDS描述了动态纹理,该纹理在时间上表现出一定的平稳性。由于时空补丁比视频序列更类似于线性时不变系统,因此采用它们来建模从视频序列中提取的时空补丁。值得注意的是,LDS不在欧几里德空间中生活。因此,我们采用核主角来度量LDS之间的相似度,然后使用多类谱聚类来生成特征表示袋的码本。其次,我们提出了一种监督式码本删减方法,以保留可区分的视觉单词并抑制每个动作类别中的噪声。选择使类间距离最大化而使类内距离最小化的视觉词进行分类。我们的方法在三个基准数据集上产生了最先进的性能。特别是,在具有挑战性的UCF体育和故事片数据集上进行的实验证明了该方法在逼真的复杂场景中的有效性。
资源推荐
资源评论
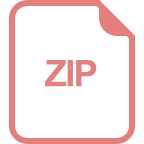
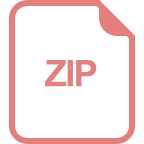
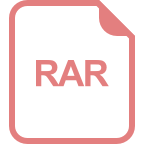
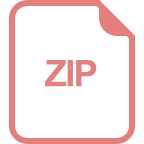
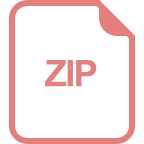
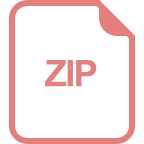
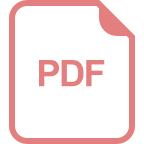
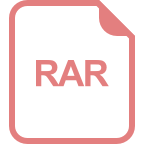
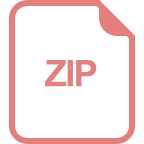
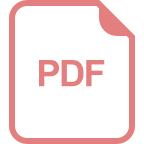
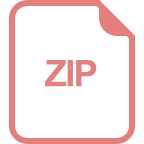
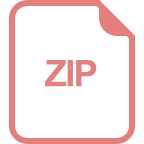
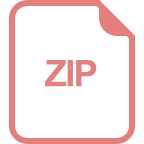
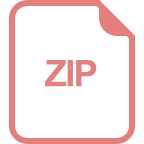
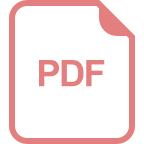
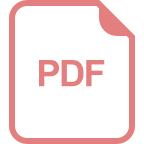
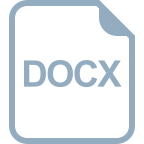
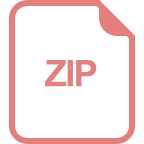
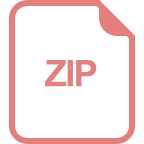
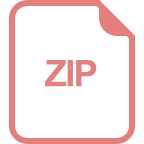
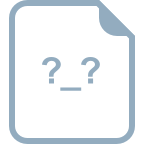
资源评论
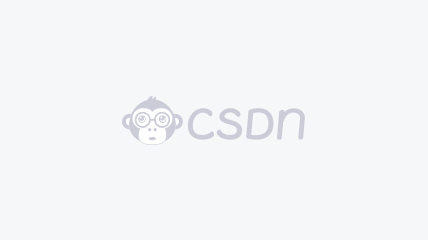

weixin_38655987
- 粉丝: 8
- 资源: 933
上传资源 快速赚钱
我的内容管理 展开
我的资源 快来上传第一个资源
我的收益
登录查看自己的收益我的积分 登录查看自己的积分
我的C币 登录后查看C币余额
我的收藏
我的下载
下载帮助

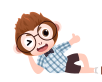
安全验证
文档复制为VIP权益,开通VIP直接复制
