没有合适的资源?快使用搜索试试~ 我知道了~
识别癌症基因组之间的互斥性:发现遗传相互作用并揭示肿瘤易感性的计算方法
2 下载量 24 浏览量
2021-04-02
18:14:01
上传
评论
收藏 1.25MB PDF 举报
温馨提示
癌症基因组的系统测序显示出普遍的异质性,患者具有多种遗传改变的组合模式。 特别是,一组基因表现出互斥模式的现象已在整个癌症中广泛传播,涵盖了广泛的关键癌症途径。 最近,有大量证据表明,互斥性反映了肿瘤发生和发展中的替代功能,或暗示了它们并发的不利影响。 鉴于其重要性,已提出了许多计算方法来单独使用基因组图谱或通过整合网络和表型来研究相互排他性。 其中一些已被常规用于探索遗传关联,从而导致对致癌机理的更深入了解,并揭示出意料之外的肿瘤脆弱性。 在这里,我们从癌症基因组的角度介绍了互斥性。 我们描述了互斥性的共同假设,总结了重要互斥模式的识别策略,从模拟数据集中比较了代表性算法的性能,并讨论了它们的共同混杂因素
资源推荐
资源详情
资源评论
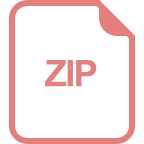
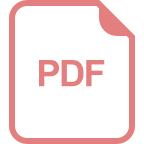
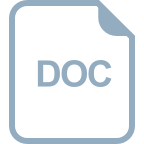
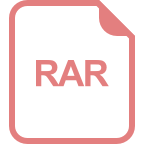
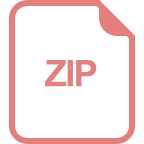
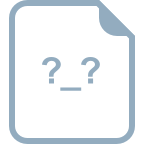
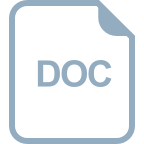
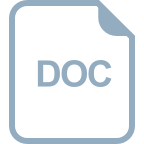
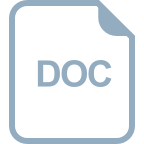
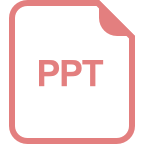
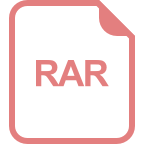
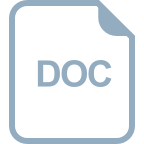
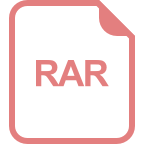
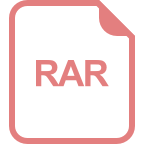
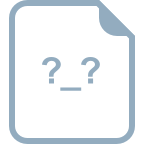
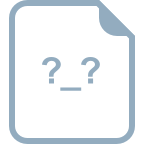
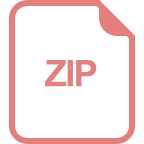
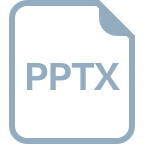
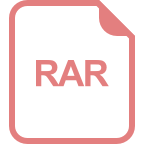
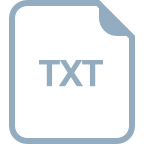
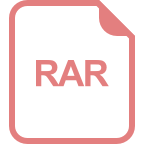
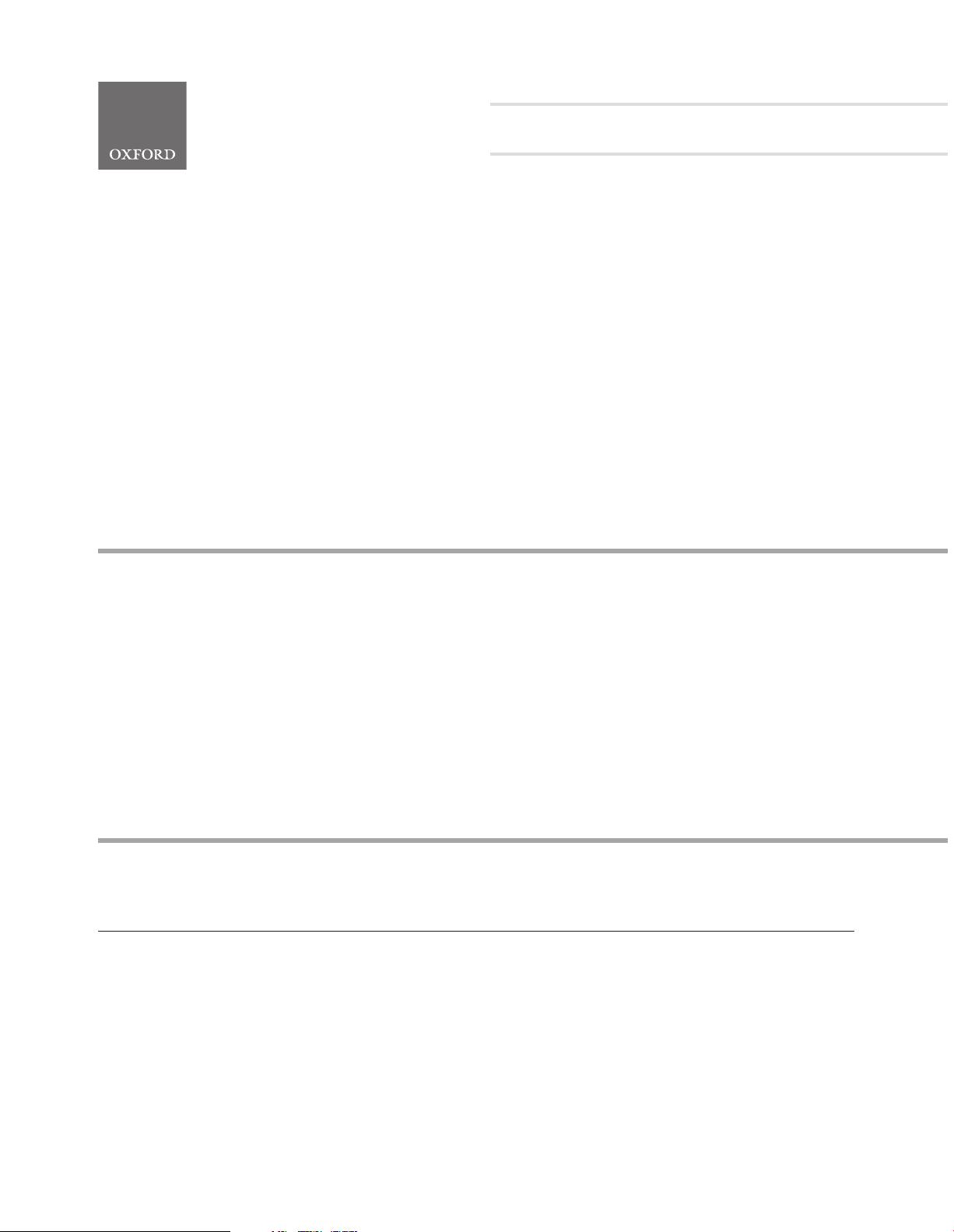
Identifying mutual exclusivity across cancer genomes:
computational approaches to discover genetic
interaction and reveal tumor vulnerability
Yulan Deng*, Shangyi Luo*, Chunyu Deng*, Tao Luo, Wenkang Yin,
Hongyi Zhang, Yong Zhang, Xinxin Zhang, Yujia Lan, Yanyan Ping,
Yun Xiao and Xia Li
Corresponding authors: Xia Li, College of Bioinformatics Science and Technology, Harbin Medical University, Harbin, Heilongjiang 150081, China. Tel.: 86-
451-86615922; Fax: 86-451-86615922; E-mail: lixia@hrbmu.edu.cn; Yun Xiao, College of Bioinformatics Science and Technology, Harbin Medical University,
Harbin, Heilongjiang 150081, China. Tel.: 86-451-86615922; Fax: 86-451-86615922; E-mail: xiaoyun@ems.hrbmu.edu.cn
*These authors contributed equally to this work.
Abstract
Systematic sequencing of cancer genomes has revealed prevalent heterogeneity, with patients harboring various combina-
torial patterns of genetic alteration. In particular, a phenomenon that a group of genes exhibits mutually exclusive patterns
has been widespread across cancers, covering a broad spectrum of crucial cancer pathways. Recently, there is considerable
evidence showing that, mutual exclusivity reflects alternative functions in tumor initiation and progression, or suggests
adverse effects of their concurrence. Given its importance, numerous computational approaches have been proposed to
study mutual exclusivity using genomic profiles alone, or by integrating networks and phenotypes. Some of them have
been routinely used to explore genetic associations, which lead to a deeper understanding of carcinogenic mechanisms and
reveals unexpected tumor vulnerabilities. Here, we present an overview of mutual exclusivity from the perspective of
cancer genome. We describe the common hypothesis underlying mutual exclusivity, summarize the strategies for the
identification of significant mutually exclusive patterns, compare the performance of representative algorithms from
simulated data sets and discuss their common confounders.
Key words: genomics; mutual exclusivity; functional redundancy; synthetic lethality; integration analysis
Yulan Deng is a PhD student in College of Bioinformatics Science and Technology at Harbin Medical University.
Shangyi Luo is an MS student in College of Bioinformatics Science and Technology at Harbin Medical University.
Chunyu Deng is an MS student in College of Bioinformatics Science and Technology at Harbin Medical University.
Tao Luo is an MS student in College of Bioinformatics Science and Technology at Harbin Medical University.
Wenkang Yin is an MS student in College of Bioinformatics Science and Technology at Harbin Medical University.
Hongyi Zhang is a PhD student in College of Bioinformatics Science and Technology at Harbin Medical University.
Yong Zhang is an MS student in College of Bioinformatics Science and Technology at Harbin Medical University.
Xinxin Zhang is an MS student in College of Bioinformatics Science and Technology at Harbin Medical University.
Yujia Lan is a PhD student in College of Bioinformatics Science and Technology at Harbin Medical University.
Yanyan Ping is an associate professor in College of Bioinformatics Science and Technology at Harbin Medical University.
Yun Xiao is an associate professor in College of Bioinformatics Science and Technology at Harbin Medical University.
Xia Li is a professor in College of Bioinformatics Science and Technology at Harbin Medical University.
Submitted: 23 May 2017; Received (in revised form): 7 July 2017
V
C
The Author 2017. Published by Oxford University Press. All rights reserved. For Permissions, please email: journals.permissions@oup.com
1
Briefings in Bioinformatics, 2017, 1–13
doi: 10.1093/bib/bbx109
Paper
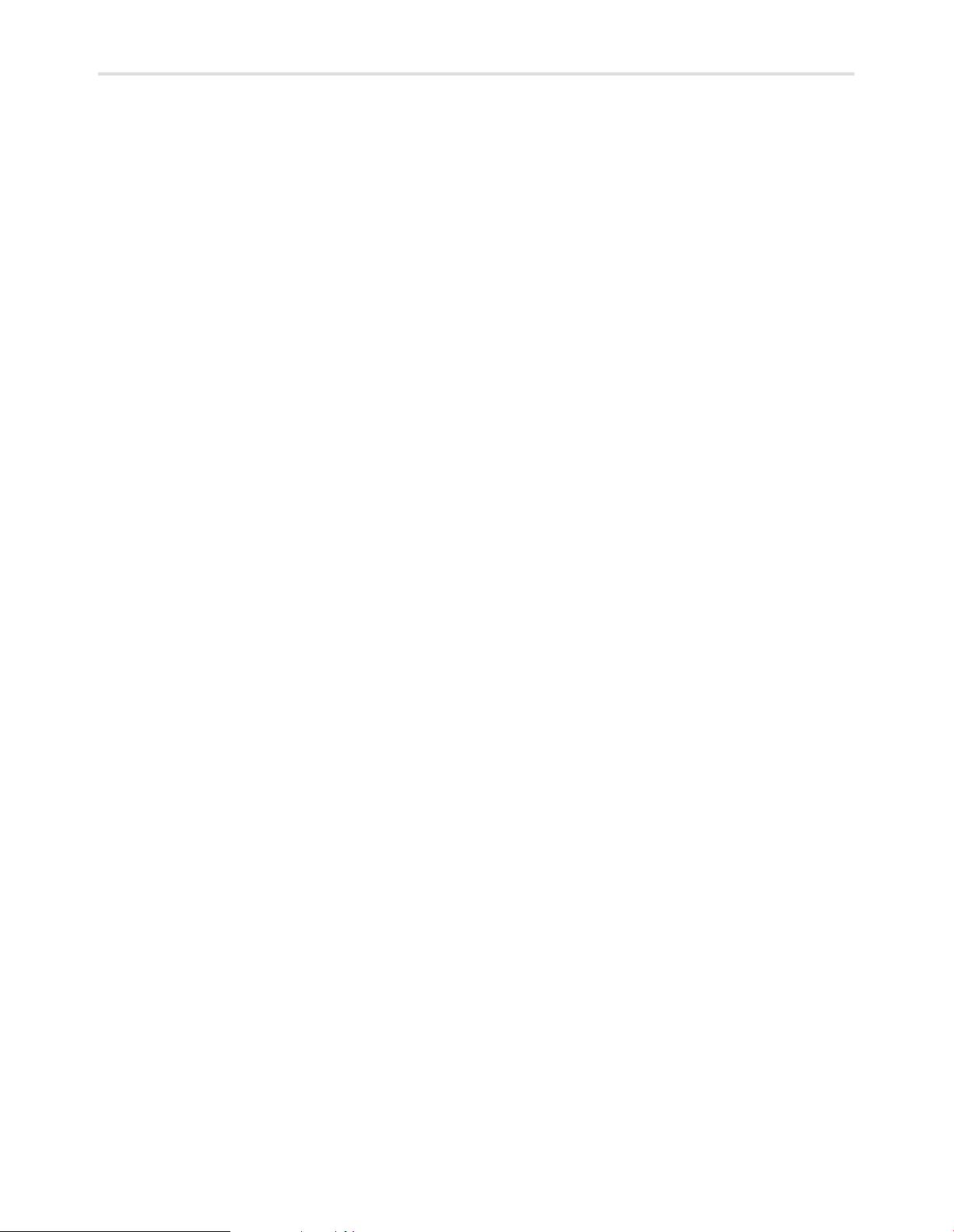
Introduction
Cancer is driven by accumulating somatic alterations during an
individual’s lifetime. Thanks to large-scale cancer sequencing
projects [1, 2], tens of thousands of tumor genomes have been
released, charting the landscapes of genetic alteration across
multiple cancers [1–7]. Some genes were found to contribute to
cancerogenesis through conferring cell growth advantage,
referred to as drivers [8–10]. However, patients seldom harbor
exactly the same driving genetic alterations, thus showing ex-
tensive genetic heterogeneity [11–14].
Mutual exclusivity refers to the phenomenon that genetic al-
teration of a group of genes does not tend to occur in the same
sample, which has been widely observed in different cancer co-
horts [15, 16]. More than one quarter of well-known cancer
genes are involved in mutual exclusivity (see Supplementary
Materials)[17], covering a broad spectrum of cancer pathways,
such as RTK/RAS/PI(3) K pathway, TP53 pathway and Wnt path-
way. For example, BRAF and NRAS, two members of MAPK path-
way, undergo genetic alterations in 40 and 25% of melanoma
patients, respectively, while only a few patients harbor both
genetic alterations [18]. They exhibit a significant mutual exclu-
sivity. Such mutual exclusivity between BRAF and NRAS has
also been reported in other cancer types, such as papillary thy-
roid carcinomas [19], myeloma [20] and colorectal cancer [21].
Similarly, KRAS and EGFR mutations are mutually exclusive in
lung adenocarcinoma, which, respectively, account for 30 and
15% lung adenocarcinoma patients [22]. Forced expression of
both oncogenic mutations causes a proliferation and/or survival
disadvantage in cancer cells [23].
Therefore, several computational approaches are developed
to investigate mutually exclusive patterns in cancer genome.
Toward a better understanding of mutual exclusivity and asso-
ciated approaches, we present an overview of mutual exclusiv-
ity from the perspective of cancer genome. First of all, we briefly
describe possible hypotheses underlying mutual exclusivity.
Then, we overview strategies that have been adopted to identify
mutually exclusive modules, and compare the performance of
representative algorithms from simulated data sets at different
parameters. Finally, we comment on common confounders,
and provide recommendations to minimize some of them.
Common hypothesis of mutual exclusivity
Recently, two biologically plausible hypotheses of mutual exclu-
sivity have been proposed (Figure 1A, Table 1). The first hypoth-
esis is that mutually exclusive genomic events are functionally
linked in a common biological pathway (Figure 1B)[15, 24–27].
These mutually exclusive genes are supposed to affect the same
downstream effectors, thus showing functional redundancy.
Therefore, it is assumed that they share selection bonus, that is
the aberration of one gene is enough to completely disrupt their
common pathways, eliminating the selective pressure for alter-
ation of the others [28]. For example, FGFR3 and RAS mutations
were mutually exclusive in bladder cancer, and simultaneous
alteration of them could not further increase proliferation
phenotype [29]. The functional redundancy hypothesis of mu-
tual exclusivity can be indicative for aberrant functional status
of cancer genome, and can help reveal functional roles un-
known genetic alterations [24, 30, 31].
The second hypothesis is that the co-occurrence of these mu-
tually exclusive genetic alterations is detrimental to cancer cell
survival [32, 33]; therefore, the cells with such co-occurrence are
eliminated from the cancer cell population (Figure 1C). Particularly,
for a pair of mutually exclusive genes, if genetic alteration of either
alone is compatible with cell survival but alteration of both will
result in cell death, these two genes are synthetic lethal [23].
For example, BRCA1 mutation and CCNE1 amplification were mu-
tually exclusive in ovarian cancer [33]. Suppression of BRCA1 in
CCNE1-amplified cells can result in synthetic lethality. A possible
explanation is that mutation of BRCA1 destroys intact homolo-
gous recombination function that is necessary for the survival of
CCNE1-amplified cells, which already show genomic instability.
Notably, synthetic lethality can improve effectiveness of target
therapy in a context-dependent manner, which can provide new
insights into therapeutic design [34]. For example, inactivation of
PARP1/2 was mutually exclusive with BRCA1/2 mutation [35].
Adding the PARP1/2 inhibitor to BRCA1 or BRCA2 deficient cells
led to favorable responses in several clinical trials and retrospect-
ive analyses [36]. Similar cases include KRAS and EGFR in lung
adenocarcinomas [22], and WT1 and TET2 in acute myeloid leuke-
mia [37].
Computational methods for identifying
mutual exclusivity
At present, numerous computational methods have been de-
veloped to identify mutual exclusivity (Table 2). These methods
can be broadly divided into two categories: de novo methods and
knowledge-based methods (Figure 2). The de novo methods only
use genomic data to explore nonrandom mutually exclusive
patterns, while the knowledge-based methods integrate other
information, such as signaling pathways, protein–protein inter-
action networks and/or phenotype data. Below, we discuss sev-
eral representative computational methods, and comment on
their advantages and disadvantages.
De novo identification of mu tual exclusivity
Pairwise test for mutual exclusivity
The simplest way to identify mutual exclusivity is to perform
pairwise statistical analysis. As an initial study, Yeang et al. [38]
used Fisher’s exact test and likelihood ratio methods (Figure 2Aa)
to explore combinatorial patterns of somatic mutations and
found tissue-specific mutually exclusive patterns. However, both
of these two methods assumed that genetic alterations are
evenly distributed across samples, which has proved to be prob-
lematic [12, 31, 39, 40]. To address this problem, Kim et al.[41]
developed WeSME. WeSME estimates the null distribution of the
mutation profile by weighted sampling, which was proportional
to the observed mutation frequency. By comparing the number
of patients covered by a pair of exclusive genes with those from
the null distribution, the significance of the mutual exclusivity is
determined.
Combinatorial score for mutually exclusive modules
Indeed, mutual exclusivity may involve more than a pair of
genes, forming mutually exclusive modules [42]. The pairwise
test methods are not suitable for identifying mutually exclusive
modules because they may be too strict to find some biologically
significant mutually exclusive pairs [43]. Instead, combinatorial
score methods are developed to identify mutually exclusive gene
modules (Figure 2Ab) [44–46]. In principle, in a mutually exclu-
sive module, the alterations of member genes should cover more
samples (called high coverage), most samples should be covered
by exactly one module member (called high exclusivity), and few
2|Deng et al.
剩余12页未读,继续阅读
资源评论
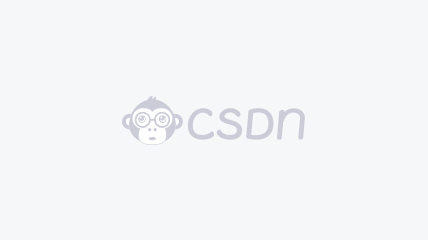

weixin_38640794
- 粉丝: 4
- 资源: 942
上传资源 快速赚钱
我的内容管理 展开
我的资源 快来上传第一个资源
我的收益
登录查看自己的收益我的积分 登录查看自己的积分
我的C币 登录后查看C币余额
我的收藏
我的下载
下载帮助

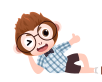
安全验证
文档复制为VIP权益,开通VIP直接复制
