没有合适的资源?快使用搜索试试~ 我知道了~
具有IQI的大规模MIMO的宽线性预编码:算法和性能分析
需积分: 5 0 下载量 76 浏览量
2021-04-12
09:30:50
上传
评论
收藏 1.41MB PDF 举报
温馨提示
在本文中,我们研究了广泛的线性预编码技术,以减轻大规模多输入多输出(MIMO)系统下行链路中的同相/正交相位(IQ)不平衡(IQI)。 我们采用实值信号模型,该模型考虑了发射机处的IQI,然后开发了宽线性迫零(WL-ZF),宽线性匹配滤波器,宽线性最小均方误差和宽线性块-适用于单天线和多天线用户的对角线化(WL-BD)类型的预编码算法。 我们还介绍了WL-ZF和WL-BD的性能分析。 事实证明,在没有IQI的情况下,WL-ZF的复用增益和功率偏移与ZF完全相同,而当存在IQI时,WL-ZF可以实现具有理想IQ分支的ZF相同的复用增益,但功耗很小,这与系统规模和IQ参数有关。 我们还比较了WL-BD和BD的性能。 分析表明,通过理想的IQ分支,WL-BD具有与BD相同的数据速率,而当存在IQI时,WL-BD可获得与BD相同的复用增益,而没有IQ失衡。 数值结果验证了分析结果,结果表明,所提出的广泛线性类型预编码方法明显优于传统的IQI方法,并接近具有理想IQ分支的方法。
资源推荐
资源详情
资源评论
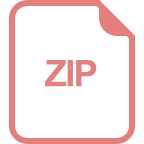
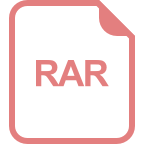
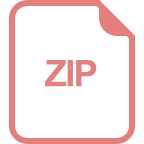
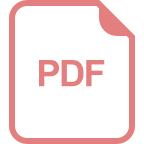
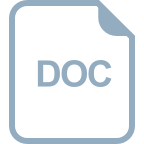
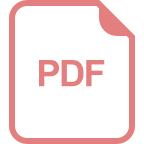
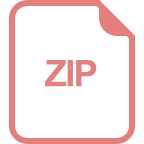
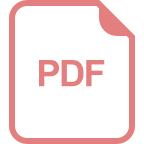
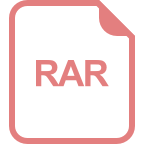
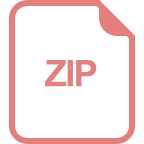
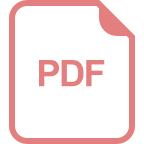
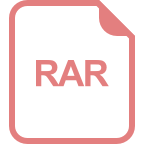
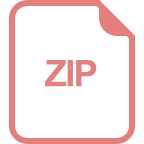
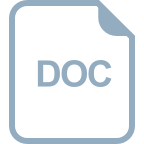
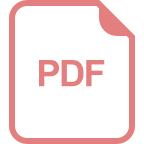
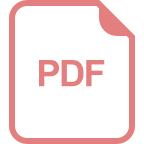
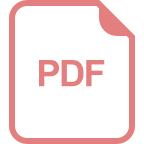
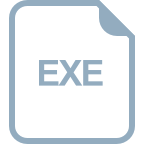
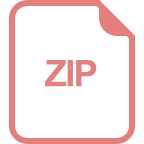
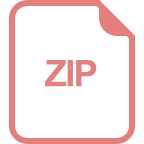
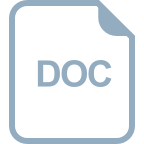
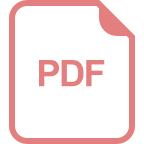
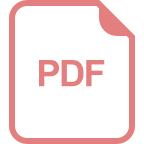
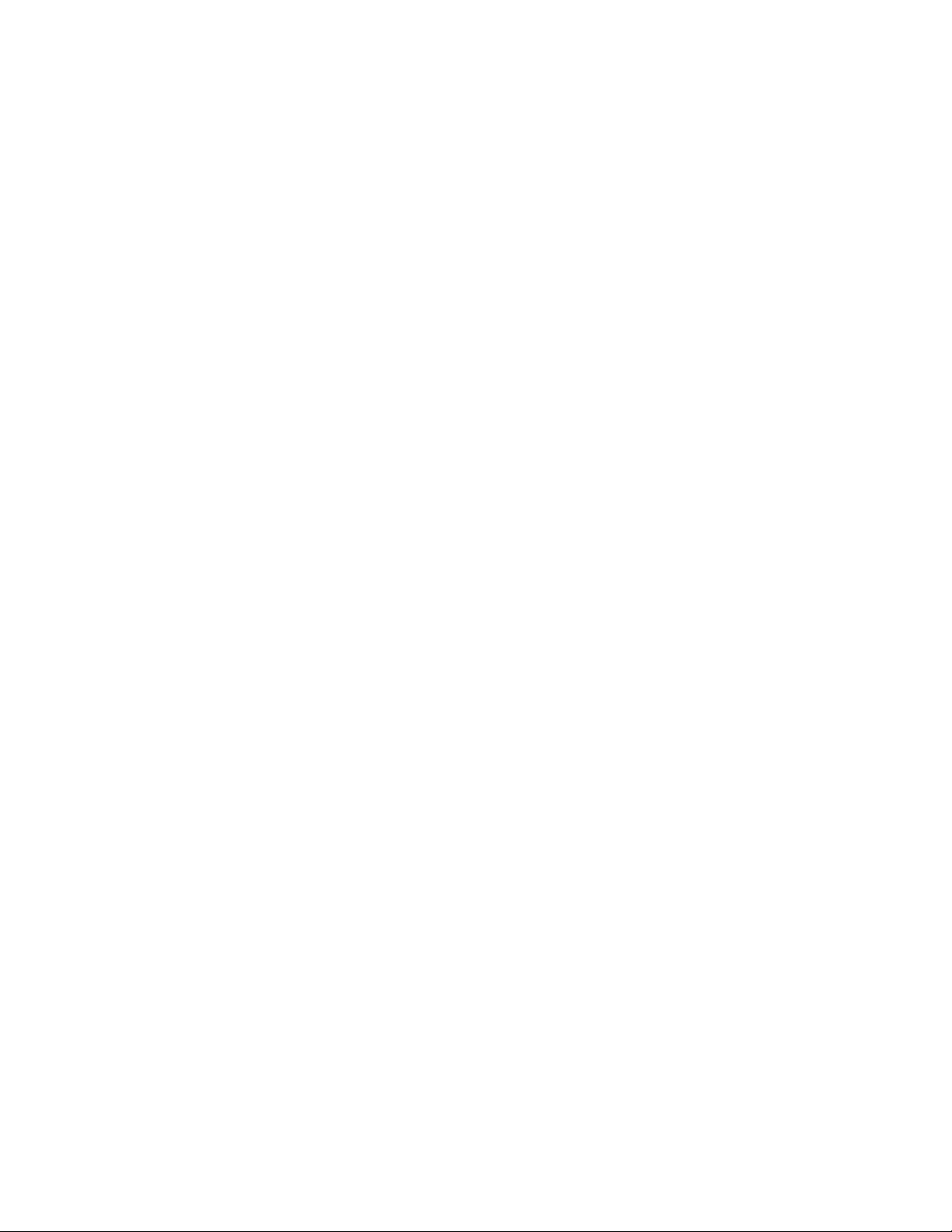
3298 IEEE TRANSACTIONS ON WIRELESS COMMUNICATIONS, VOL. 16, NO. 5, MAY 2017
Widely Linear Precoding for Large-Scale MIMO
with IQI: Algorithms and Performance Analysis
Wence Zhang, Rodrigo C. de Lamare, Senior Member, IEEE, Cunhua Pan,
Ming Chen, Jianxin Dai, Bingyang Wu, and Xu Bao
Abstract—In this paper, we study widely linear precoding
techniques to mitigate in-phase/quadrature-phase (IQ) imbal-
ance (IQI) in the downlink of large-scale multiple-input multiple-
output (MIMO) systems. We adopt a real-valued signal model,
which considers the IQI at the transmitter, and then develop
widely linear zero-forcing (WL-ZF), widely linear matched filter,
widely linear minimum mean-squared error, and widely linear
block-diagonalization (WL-BD) type precoding algorithms for
both single- and multiple-antenna users. We also present a
performance analysis of WL-ZF and WL-BD. It is proved that
without IQI, WL-ZF has exactly the same multiplexing gain and
power offset as ZF, while when IQI exists, WL-ZF achieves the
same multiplexing gain as ZF with ideal IQ branches, but with a
minor power loss, which is related to the system scale and the IQ
parameters. We also compare the performance of WL-BD with
BD. The analysis shows that with ideal IQ branches, WL-BD
has the same data rate as BD, while when IQI exists, WL-BD
achieves the same multiplexing gain as BD without IQ imbalance.
Numerical results verify the analysis and show that the proposed
widely linear type precoding methods significantly outperform
their conventional counterparts with IQI and approach those
with ideal IQ branches.
Index Terms—IQ imbalance, large-scale MIMO, widely-linear
signal processing, downlink precoding.
Manuscript received August 29, 2016; revised December 29, 2016; accepted
February 26, 2017. Date of publication March 20, 2017; date of current
version May 8, 2017. This work was supported in part by the Research
Foundation for Advanced Talents of Jiangsu University under
Grant 16JDG065, in part by the China Scholarship Council, in part by
CNPq, in part by FAPERJ, in part by PUC-Rio, in part by the University
of York, in part by the National Nature Science Foundation of China
under Grant 61221002, Grant 61571125, Grant 61401399, Grant 61372106,
Grant 61502210, and Grant 61571211, in part by the National Science
and Technology Major Project under Grant 2016ZX03001016-003, in part
by the Postdoctoral Research Funding Plan in Jiangsu Province under
Grant 1501073B, in part by the Natural Science Foundation of Nanjing Uni-
versity of Posts and Telecommunications under Grant NY214108, and in part
by the China Postdoctoral Science Foundation under Grant 2015M570484.
Part of this paper was presented in Eusipco 2014 and ICC 2015. The associate
editor coordinating the review of this paper and approving it for publication
was R. Brown. (Corresponding author: Cunhua Pan.)
W. Zhang is with Jiangsu University, Zhenjiang 212013, China. He
was with CETUC, PUC-Rio, Rio de Janeiro 22451-900, Brazil. (e-mail:
wencezhang@ujs.edu.cn).
R. C. de Lamare is with the University of York, York YO10 5DD, U.K.,
and also with PUC-Rio, Rio de Janeiro, Brazil (e-mail: delamare@cetuc.
puc-rio.br).
C. Pan is with the School of Electronic Engineering and Computer
Science, Queen Mary University of London, London, U.K. (e-mail:
c.pan@qmul.ac.uk).
M. Chen and B. Wu are with the National Mobile Communications Research
Laboratory, Southeast University, Nanjing 210096, China (e-mail: chenming@
seu.edu.cn; wubingyang@seu.edu.cn).
J. Dai is with the School of Science, Nanjing University of Posts and
Telecommunications, Nanjing 210003, China (e-mail: daijx@njupt.edu.cn).
X. Bao is with Jiangsu University, Zhenjiang 212013, China (e-mail:
xbao@ujs.edu.cn).
Color versions of one or more of the figures in this paper are available
online at http://ieeexplore.ieee.org.
Digital Object Identifier 10.1109/TWC.2017.2679706
I. INTRODUCTION
W
IRELESS communications systems have undergone
tremendous development during the past decades.
In order to meet the increasing demands for data services,
many new techniques have been proposed, among which
multiple-input multiple-output (MIMO) techniques play an
important role. In the 5th generation (5G) of mobile commu-
nication systems, one of the key techniques will be large-scale
MIMO, which employs a large number of antennas at the base
station (BS) with centralized or distributed antenna systems to
provide extremely high data rates with improved quality of
service (QoS) [1], [2].
One of the main performance constraints of large-scale
MIMO systems comes from the impairments resulting from
hardware [3], [4]. Since large-scale MIMO systems employ a
large number of antennas, cheaper hardware is preferable in
order to reduce the cost, which may cause severe hardware
imperfection, e.g., the in-phase and quadrature-phase (IQ)
imbalance (IQI) [5]. Modern transceivers usually use the direct
conversion structure which contains two branches to process
the real and imaginary components of the baseband signals,
i.e., the in-phase (I) branch and the quadrature-phase (Q)
branch. The IQI exists when there is a gain difference between
the two branches and/or the phase difference is not exactly 90
◦
.
The IQI can be present at both the transmitter and the receiver,
according to many studies [6]–[8].
One way of handling IQI is to estimate the IQ parameters
and compensate for them (see [8]–[10] and references therein).
However, the IQ parameters are usually mingled with the
channel coefficients, and thus are difficult to obtain, especially
for large-scale MIMO systems, where the number of IQ
parameters is proportional to the system size and thus the esti-
mation and compensation for IQI can be very computationally
expensive.
Widely-linear approaches have long been used for
non-circular signal processing in MIMO systems [11]–[13]
and have been recently adopted to deal with IQI [5], [14]–[22].
In the uplink, the impact of IQI on the response pattern of large
antenna arrays is studied in [15]. The work in [16] describes an
equivalent interference model to study the impact on orthog-
onal frequency division multiple access (OFDMA) large-scale
MIMO systems and devised a receiver based on widely-linear
signal processing, which is extended in [22] to scenarios with
external interference. Kolomvakis et al. [20] investigated the
impact of IQI on the performance of uplink Massive MIMO
systems with maximum-ratio combining (MRC) receivers, and
showed that IQI can substantially degrade the performance
of MRC receivers. The study in [20] also proposed a low-
1536-1276 © 2017 IEEE. Personal use is permitted, but republication/redistribution requires IEEE permission.
See http://www.ieee.org/publications_standards/publications/rights/index.html for more information.
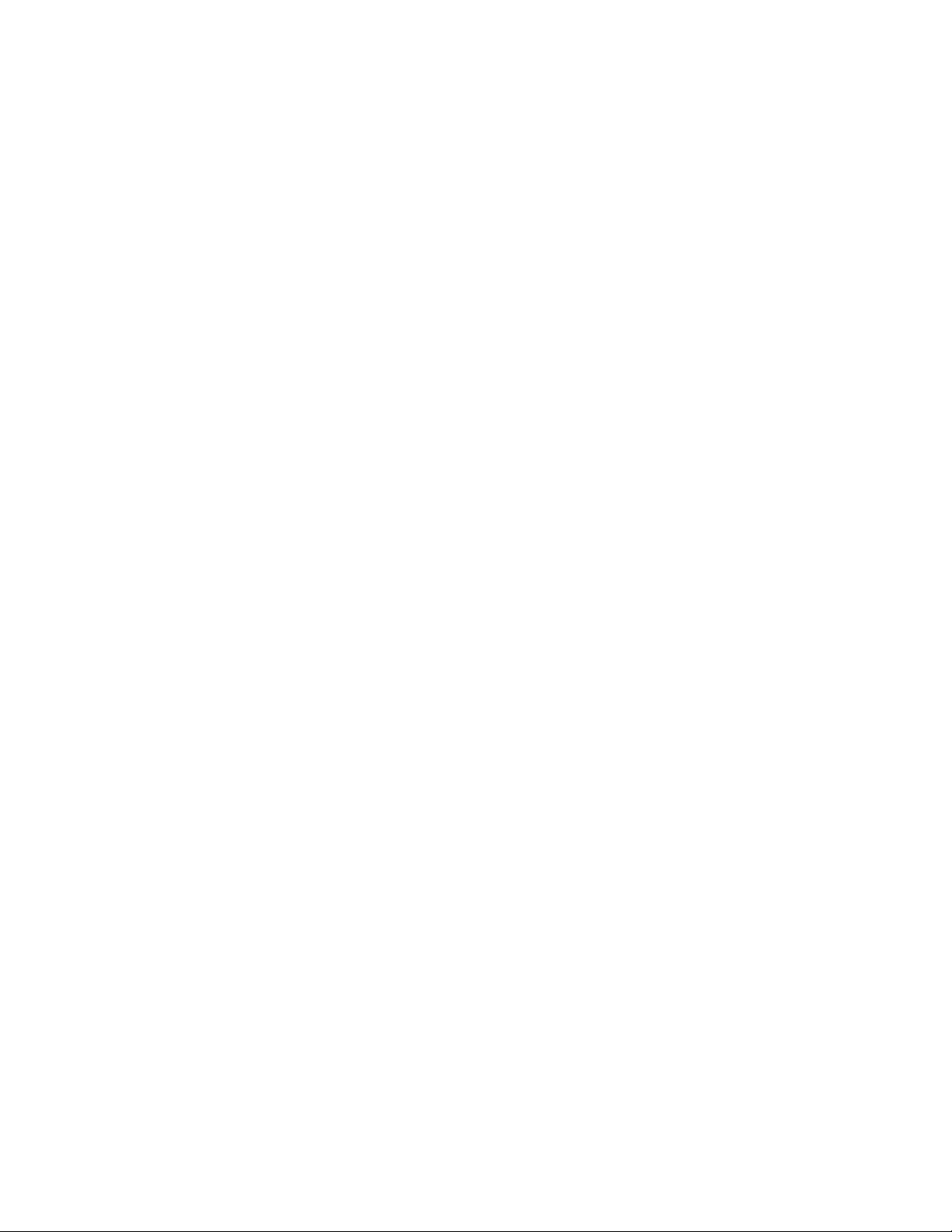
ZHANG et al.: WIDELY LINEAR PRECODING FOR LARGE-SCALE MIMO WITH IQI 3299
complexity IQI compensation scheme. In order to suppress
the impact of IQI, a data-aided widely-linear minimum mean
square error (MMSE) receiver is proposed in [23], and an
IQI aware receiver was designed in [5] for the large-scale
MIMO uplink based on the minimum variance distortionless
response (MVDR) criterion. In [21], a widely-linear MMSE
receiver is proposed, the performance of which is shown to
be close to the linear MMSE receiver in an ideal system
without IQI.
To the best of the authors’ knowledge, there are only
a few related works in the downlink [14], [17]–[19]. The
study in [14] uses an augmented representation to maxi-
mize the power of the desired transmit signal when IQI is
present. Reduced-rank widely-linear precoders were devised
in [17] for single-antenna users to alleviate the impact
of IQI as well as to reduce the computational complex-
ity. A similar work was reported later in [18], which
studied the impact of IQI and proposed a widely-linear
regularized zero-forcing (RZF) precoding scheme. In [19],
we extended our previous work in [17] to scenarios with
multiple-antenna users in the large-scale MIMO downlink
and developed novel widely-linear block diagonal (BD) type
precoders.
In this paper, our previous work [17], [19] is extended
to give a comprehensive study on widely-linear precoding
algorithms for the large-scale MIMO downlink with IQI for
users with both single and multiple antennas. For large-
scale MIMO systems with single-antenna users, non-linear
precoding schemes, e.g., vector perturbation (VP) precoding
[24] and Tomlinson-Harashima precoding [25], [26], usually
have better performance than linear precoding schemes. How-
ever, linear precoding schemes, such as matched filter (MF)
(also referred to as maximum ratio transmission [27]), zero-
forcing (ZF) and MMSE [28], have much lower complexity
compared with the nonlinear precoding schemes and thus draw
great research interest [1], [2]. Generally, ZF and MMSE
perform better than MF, but with a comparatively higher
computational cost due to the matrix inversion involved.
Most current studies on large-scale MIMO downlink have
considered single-antenna users. However, it is well known
that with more receive antennas at the user equipment (UE),
the quality of service (QoS) of each user can be signifi-
cantly improved. In fact, the long term evolution (LTE) and
LTE-Advanced (LTE-A) standards can support UEs with mul-
tiple antennas [29]. In terms of downlink precoding schemes,
block-diagonalization (BD) type precoding has been widely
considered for parallel transmission of multiple data streams
for users with multiple antennas [30]–[34]. In [32], the
regularized BD (RBD) precoding has been proposed which
outperforms conventional BD in [30] and [31], by taking into
account both the inter-user interference and noise. To reduce
the complexity, the work in [33] has devised the generalized
MMSE channel inversion (GMI) by replacing the singular
value decomposition (SVD) operation in BD and RBD with
a matrix inversion and QR decomposition. This scheme has
been further modified to obtain the simplified GMI (S-GMI)
technique in [34].
In contrast to [5], [15], [16], and [20]–[23], in this work
we study the design and performance analysis for down-
link precoding in large-scale MIMO systems with transmitter
IQI, for both single- and multiple-antenna users. Although
duality exists in the uplink and downlink [35], these results
are different fort the case considered here in the down-
link. We first adopt a useful mapping function reported
in [36] and [37], which transforms complex-valued vectors
and matrices into real-valued expressions and helps to set
up an equivalent real-valued signal model with consideration
of IQI. Based on this real-valued signal model, we develop
widely-linear ZF (WL-ZF) and widely-linear MF (WL-MF)
and widely-linear MMSE (WL-MMSE) precoders, which are
suited for single-antenna terminals. Unlike [14], [17], and [18]
where only single-antenna users are considered, we propose
widely-linear BD (WL-BD) type precoding algorithms, i.e.,
WL-BD, widely-linear RBD (WL-RBD) and widely-linear
S-GMI (WL-S-GMI), for users equipped with multiple anten-
nas. A performance analysis is carried out for the ZF and
BD versions of these proposed precoding schemes, which
captures the essential advantages of widely-linear precoding
approaches. By utilizing an affine approximation of the sum
data rate developed in [38], the mathematical expression
for the sum data rate of WL-ZF is derived. Moreover, we
also compare ZF and WL-ZF in terms of both multiplex-
ing gain and power offset, where the IQ parameters are
treated as random variables in the analysis, which is different
from [18], [21], and [22], where the IQ parameters are
fixed. For WL-BD, the sum data rate and multiplexing gain
are derived and compared with those of BD. We also give
simulation results to show the impact of IQI in large-scale
MIMO systems, as well as the performance of the proposed
widely-linear precoding schemes.
The main contributions of this paper are summarized as
follows:
• We extend previous work of WL-ZF, WL-MF and
WL-MMSE precoding for large-scale MIMO systems
with IQI and single-antenna users in [17] to cases with
multiple-antenna users and propose WL-BD, WL-RBD
and WL-S-GMI algorithms based on an equivalent
real-valued signal model.
• For WL-ZF, we show that it has the same multiplexing
gain as that of ZF with ideal IQ branches. The achieved
multiplexing gain equals the number of users. Compared
with ZF without IQI, WL-ZF has a power offset loss
around log
2
[1+4σ
2
g
β],whereβ is the ratio of the number
of users to that of the transmit antennas and σ
2
g
is the
variance of the gain difference between I and Q branches.
• For WL-BD, we prove that when there is no IQI,
it achieves the same sum data rate as BD, while the
WL-BD precoder in the presence of IQI has the same
multiplexing gain as that of BD with ideal IQ branches.
Moreover, compared with BD the increased complexity
of WL-BD is very small.
This paper is organized as follows. The system model is
described in Section II. In Section III, the proposed widely-
linear precoding algorithms are introduced. In Section IV, the
performance analysis of WL-ZF and WL-BD is carried out.
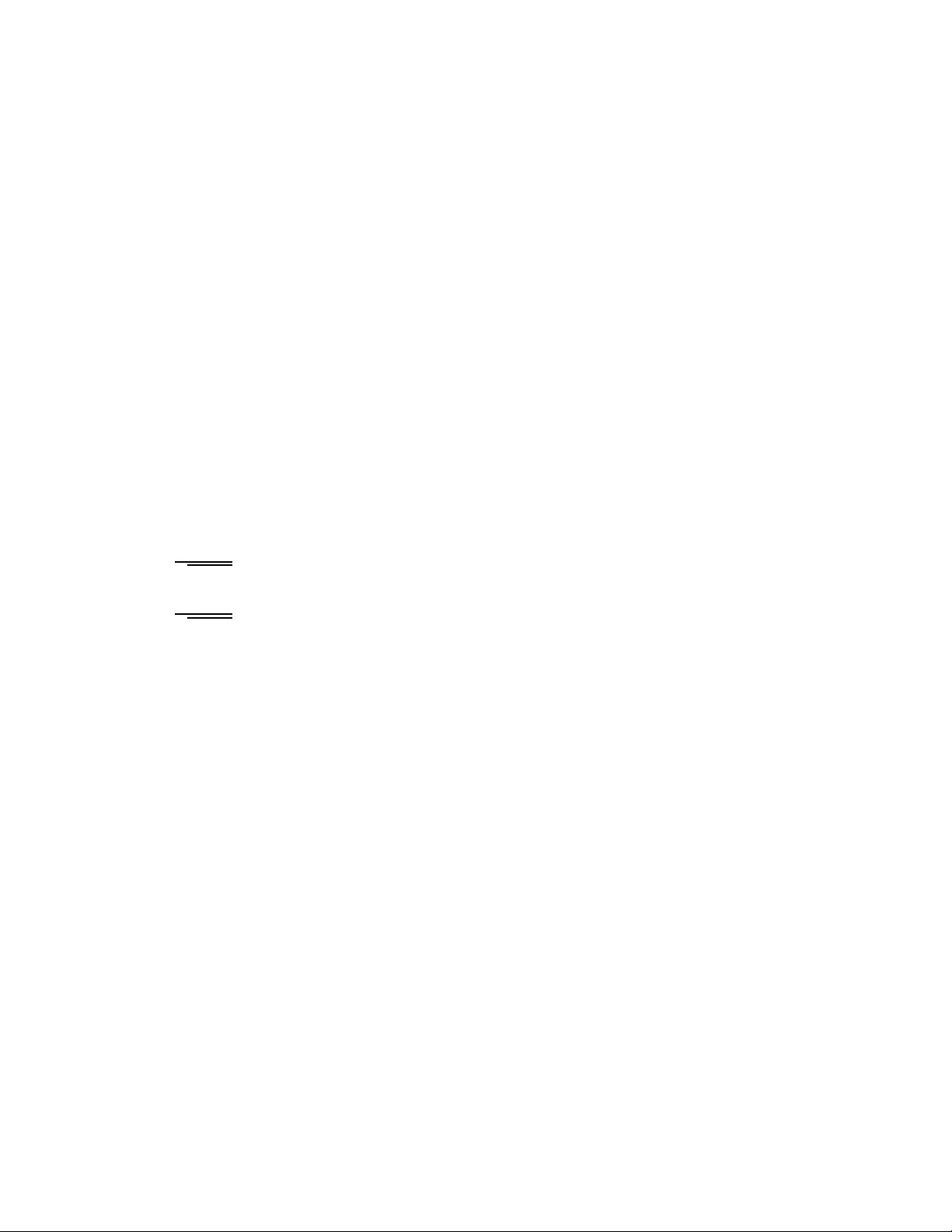
3300 IEEE TRANSACTIONS ON WIRELESS COMMUNICATIONS, VOL. 16, NO. 5, MAY 2017
The numerical results are given in Section V and conclusions
are drawn in Section VI.
Notation: C
N
and C
N×M
denote the sets of N-dimensional
complex vectors and N × M complex matrices, respectively;
R
N
and R
N×M
denote the sets of N-dimensional real vectors
and N × M real matrices, respectively; (·)
∗
is the complex
conjugate; I
N
denotes an N × N identity matrix; CN (θ , )
denotes circularly symmetric complex Gaussian distribution
with mean θ and covariance ; U(a, b) denotes the uniform
distribution and N (a, b) denotes the Gaussian distribution,
where a and b are the mean and variance, respectively;
diag{a
1
, ···, a
K
} denotes a diagonal matrix with diagonal
entries given by a
1
, ···, a
K
; E{·} denotes the mathematical
expectation and Tr[·] denotes the trace of a matrix A.
II. S
YSTEM MODEL
Consider the downlink of a large-scale MIMO system with
one BS and K users. The BS is equipped with N antennas, and
there are M
k
antennas at the k-th user. Define M =
K
k=1
M
k
as the total number of antennas at all the users. Consider
the IQI at all the N transmit antennas at BS,
1
and for the
n-th antenna, the transmit symbol x
n
is corrupted by IQI as
a
n1
x
n
+ a
n2
x
∗
n
,wherea
n1
and a
n2
are the IQ parameters of
the n-th antenna that are modeled as [6]:
a
n1
=
1
1 + σ
2
g
[cos(θ
n
/2) + jg
n
sin(θ
n
/2)],
a
n2
=
1
1 + σ
2
g
[g
n
cos(θ
n
/2) − jsin(θ
n
/2)], (1)
where θ
n
∼ U(0,σ
2
θ
) and g
n
∼ N (0,σ
2
g
) are the relative
phase and gain mismatches between the IQ branches of the
n-th transmit antenna, respectively. The IQ parameters are
normalized so that they do not change the average signal
power. A proof of the selection of the normalization factor
is given in Appendix A. In (1), θ
n
= 0
◦
and g
n
= 0represent
the ideal case with no IQI. Note that although the Gaussian
and uniform distributions are considered for modeling IQ
parameters, the proposed algorithms and the performance
analysis are valid for other distributions.
If we consider the IQI at BS, the received signal y
k
∈ C
M
k
at the k-th user is given by
y
k
= H
k
A
1
x + H
k
A
2
x
∗
+ n
k
, (2)
where H
k
∈ C
M
k
×N
is the downlink channel matrix of
the k-th user, the elements of which are independent and
identically distributed (i.i.d.) Gaussian random variables with
zero-mean and unit variance; A
1
= diag{a
11
, ···, a
N1
} and
A
2
= diag{a
12
, ···, a
N2
}; n
k
∼ CN (0,σ
2
n
I
M
k
) is the noise
vector at the receiver; x ∈ C
N
is the transmit signal vector
after precoding. Here we consider a narrow-band single-
carrier system for simplicity and the extension to multi-carrier
systems remains open for future work.
1
The IQI at the user’s receiver only degrades its own signal and can be
addressed individually by IQI compensation techniques [8]. In contrast, the
IQI at the BS affects all the users and is severe in large-scale MIMO systems
due to the potential use of cheap hardware for cost issues. Therefore, we only
consider the IQI at the BS in this paper.
Let L
k
be the number of data streams of user k and
P
k
∈ C
N×L
k
, s
k
∈ C
L
k
be the precoder and the transmit
signal vector for the k-th user, respectively. Denote P =
[P
1
, ···, P
K
], s =[s
T
1
, ···, s
T
K
]
T
, and we have
x = Ps.
Note that in contrast to single-antenna users using ZF, MMSE
or MF, in the case of multiple-antenna users using BD type
precoders, a receive filter matrix is generally required to
decode the multiple streams which is designed together with
the precoder, as will be detailed later in Section III.
In this paper, we assume that the transmitter has perfect
channel state information and the transmit signals for different
users are i.i.d circularly symmetric Gaussian random variables
with zero-mean and unit variance, i.e., ∀k = j, E{s
k
s
H
j
}=0,
and E{s
k
s
H
k
}=I
L
k
. We also assume there is a transmit power
constraint, i.e.,
E{Ps
2
}=P
T
. (3)
It can be seen from (2) that the transmit signal vector is
corrupted by its complex conjugate. In the frequency domain,
a mirror frequency component is introduced due to the IQI.
One possible way of handling such IQI resorts to estimation
of the corresponding IQ parameters and pre-compensation
for the IQI [6], [7]. Since the signal model of (2) gives
rise to non-circular data which can be exploited by widely-
linear processing, IQI can also be tackled by widely-linear
approaches [5], [17].
In what follows, we will devise and carry out a performance
analysis of widely-linear precoding schemes, which are able
to mitigate the IQI without significantly increasing the com-
putational complexity.
III. P
ROPOSED WIDELY-LINEAR
PRECODING ALGORITHMS
In this section, we employ a useful transformation,
i.e., the T -transform from [36] and [37], which represents
complex-valued matrices and vectors using their real-valued
equivalents. Then we employ the T -transform to develop an
equivalent real-valued signal model, which helps to design
widely-linear precoding schemes. Several widely-linear pre-
coding algorithms such as WL-ZF, WL-MMSE, WL-BD,
WL-RBD and WL-S-GMI are then developed.
A. Real-Valued Signal Model
A mapping function of C
n
→ R
2n
and C
m×p
→ R
2m×2p
,
namely the T -transform, is defined as:
T (x) =
Re(x)
Im(x)
, T (X) =
Re(X) −Im(X)
Im(X) Re(X)
, (4)
where Re(·) and Im(·) represent the real and imaginary parts
of a vector or a matrix, respectively. The T -transform sets
up a relationship between the complex-valued matrices and
their real-valued counterparts. It is very useful for design
and performance analysis of widely-linear precoders. Some
properties of the T -transform are summarized in Lemma 1,
剩余14页未读,继续阅读
资源评论
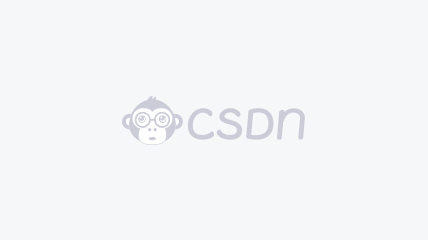

weixin_38628990
- 粉丝: 5
- 资源: 933
上传资源 快速赚钱
我的内容管理 展开
我的资源 快来上传第一个资源
我的收益
登录查看自己的收益我的积分 登录查看自己的积分
我的C币 登录后查看C币余额
我的收藏
我的下载
下载帮助

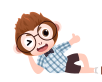
最新资源
- cyuyan语言的基础学习
- 录屏软件亲测可用哦,非常好用
- 前端分析-2023071100789
- STM32无源蜂鸣器播放音乐《千与千寻》
- 前端分析-2023071100789
- 计算机网络基础知识与核心技术详析
- 模电课设(GKS-18光控声光循环灯说明书).doc
- HCIA笔记一些网络历史,TCP/IP,osi七层模型等等
- 语音助手多任务音频文本共享模型(AT-AT)用于提升端到端口语理解系统性能及零样本预测能力
- 【锂电池剩余寿命预测】CNN卷积神经网络锂电池剩余寿命预测,马里兰大学锂电池数据集(Pytorch完整源码和数据)
- 康熙字典mysql数据库表
- 同时语音翻译领域中大规模语言模型的应用与突破-CLASI系统研究
- 华为认证HCIA笔记(第一二天)
- 【锂电池剩余寿命预测】GRU门控循环单元锂电池剩余寿命预测,马里兰大学锂电池数据集(Pytorch完整源码和数据)
- 【锂电池剩余寿命预测】LSTM长短期记忆神经网络锂电池剩余寿命预测,马里兰大学锂电池数据集(Pytorch完整源码和数据)
- 西南交通大学数电实验考试资料
资源上传下载、课程学习等过程中有任何疑问或建议,欢迎提出宝贵意见哦~我们会及时处理!
点击此处反馈


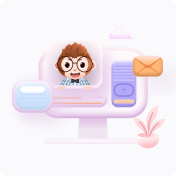
安全验证
文档复制为VIP权益,开通VIP直接复制
