没有合适的资源?快使用搜索试试~ 我知道了~
零空间分集Fisher判别分析用于人脸识别
1 下载量 92 浏览量
2021-03-12
16:06:37
上传
评论 1
收藏 303KB PDF 举报
温馨提示
试图将原始数据投影到低维特征空间中的特征提取算法引起了很多关注。 本文基于增强型Fisher判别准则(EFDC),提出了一种新的特征提取方法,称为零空间多样性Fisher判别分析(NSDFDA),用于人脸识别。 提出了基于新优化准则的NSDFDA,这意味着可以在类内散点的零空间中计算所有判别向量。 此外,所提出的算法能够提取特征空间中的正交判别矢量,并且同时不存在样本量小的问题,这是许多模式分析应用所希望的。 在Yale数据库上的实验结果证明了该方法的有效性。
资源推荐
资源详情
资源评论
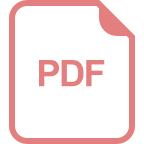
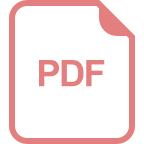
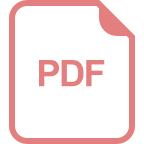
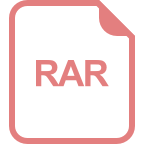
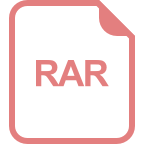
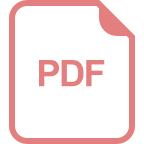
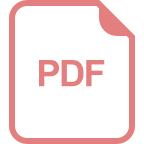
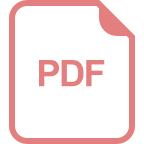
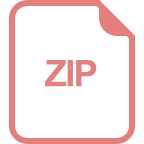
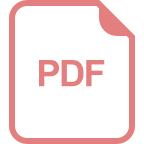
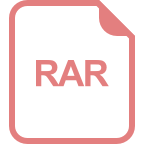
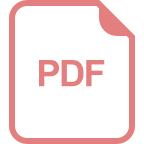
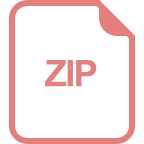
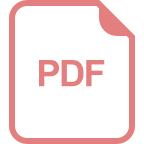
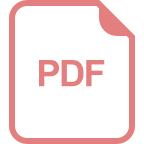
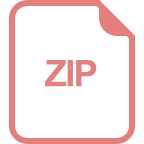
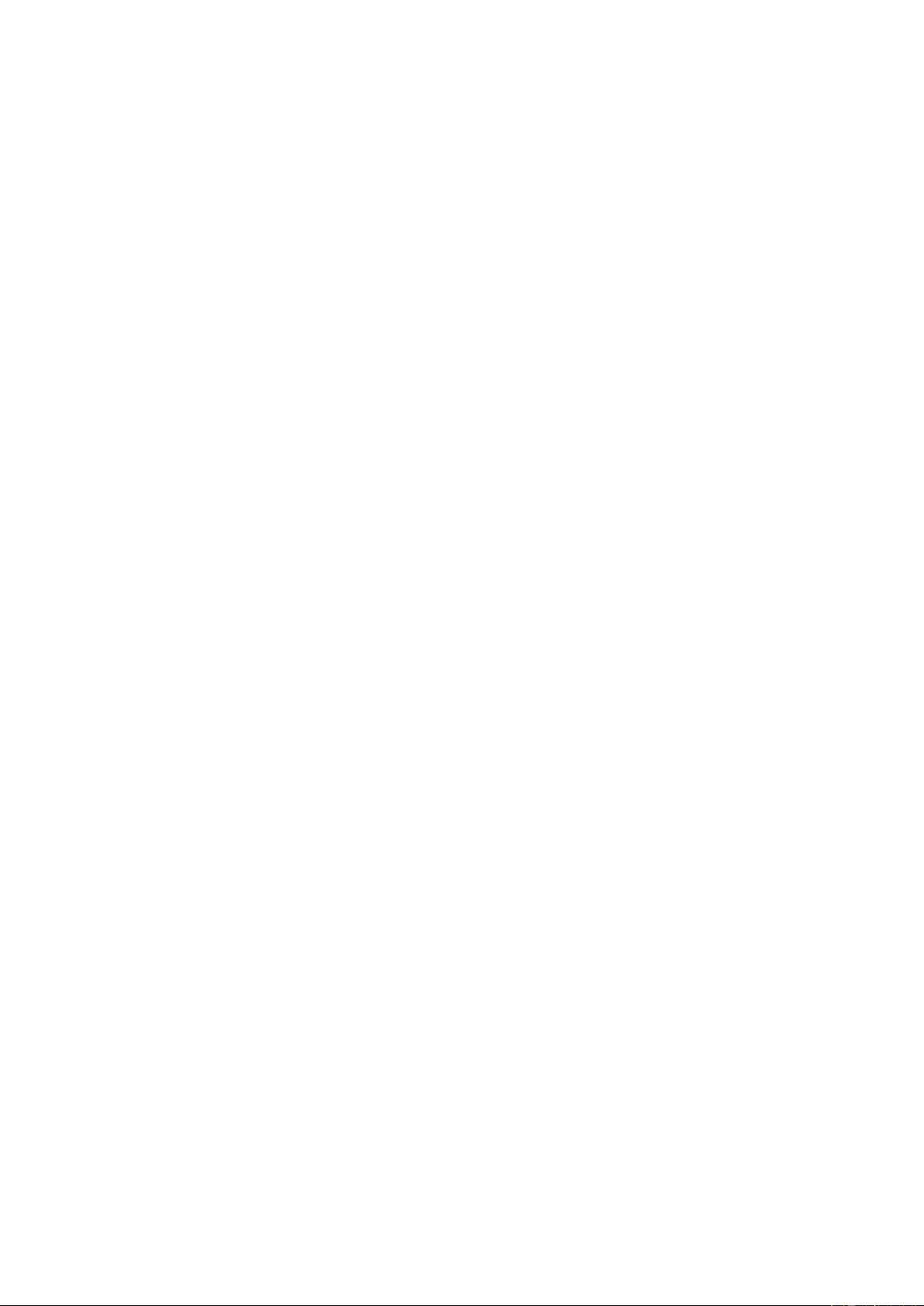
adfa, p. 1, 2011.
© Springer-Verlag Berlin Heidelberg 2011
Null Space Diversity Fisher Discriminant Analysis for
Face Recognition
Xingzhu LIANG
a
, Yu’e LIN
b
, Gaoming YANG
c
, Guangyu XU
d
School of Computer Science and Engineering, Anhui University of Science and
Technology
Huainan, 232001, China
a
lxz9117@126.com,
b
linyu_e@126.com,
c
gmyang@aust.edu.cn,
d
gyxu@aust.edu.cn
Abstract.
The feature extraction algorithms, which attempt to project the original data in-
to a lower dimensional feature space , have drawn much attention. In this paper,
based on enhanced fisher discriminant criterion (EFDC), a new feature extrac-
tion method called null space diversity fisher discriminant analysis (NSDFDA)
is proposed for face recognition. NSDFDA based on a new optimization criteri-
on is presented, which means that all the discriminant vectors can be calculated
in the null space of the within-class scatter. Moreover, the proposed algorithm is
able to extract the orthogonal discriminant vectors in the feature space and sim-
ultaneously does not suffer from the small sample size problem, which is desir-
able for many pattern analysis applications. Experimental results on the Yale da-
tabase show the effectiveness of the proposed method.
Keywords: feature extraction, enhanced fisher discriminant criterion, null
space, the within-class scatter, the small sample size problems
1 Introduction
The feature extraction is a critical issue in face recognition tasks. The goal of fea-
ture extraction is to map high dimensional data samples to a lower dimensional space
such that certain properties are preserved. Among all the dimensionality reduction
methods, Fisher linear discriminant analysis (FLDA)[1] is the most popular method
and has been widely used in many classification applications. FLDA seeks to find
directions on which the ratio of the trace of the between-class matrix and the trace of
the within-class matrix is maximized. However, some recent research shows that the
samples may reside on a nonlinear submanifold. FLDA fails to discover the underly-
ing submanifold structure, due to the fact that it aims only to preserve the global
structures of the samples. Another technique called Locality Preserving Projections
(LPP) [2] has been proposed for dimensionality reduction, which can preserve the
intrinsic geometry of data. However, LPP has no direct relationship to classification.
资源评论
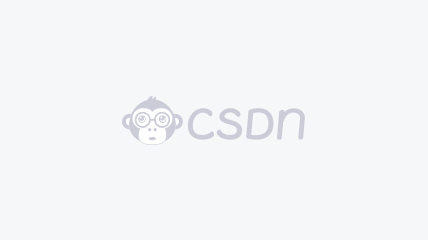

weixin_38609571
- 粉丝: 8
- 资源: 908
上传资源 快速赚钱
我的内容管理 展开
我的资源 快来上传第一个资源
我的收益
登录查看自己的收益我的积分 登录查看自己的积分
我的C币 登录后查看C币余额
我的收藏
我的下载
下载帮助

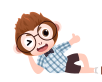
最新资源
资源上传下载、课程学习等过程中有任何疑问或建议,欢迎提出宝贵意见哦~我们会及时处理!
点击此处反馈


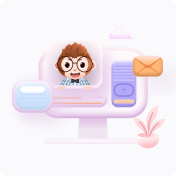
安全验证
文档复制为VIP权益,开通VIP直接复制
