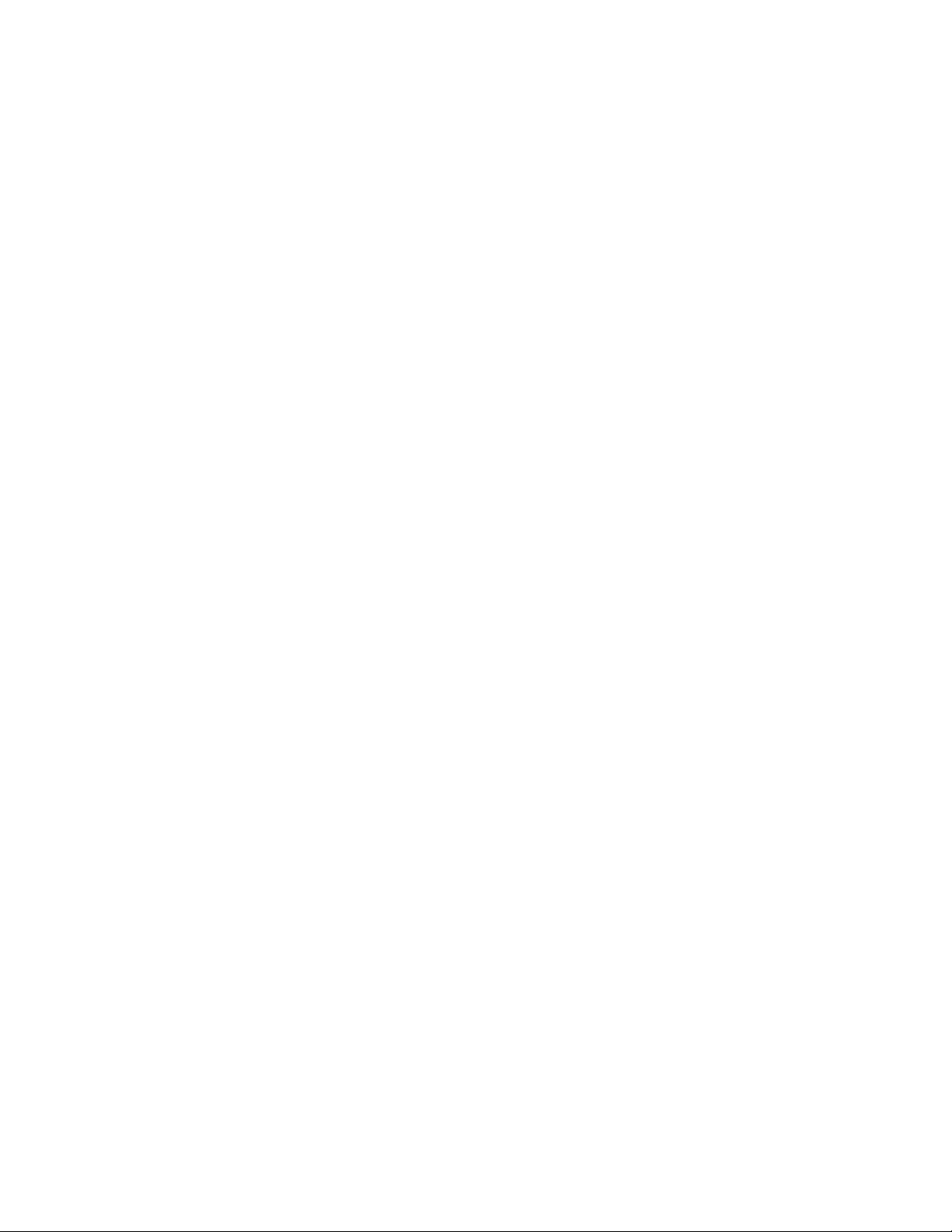
HAMA AND OCHIAI: PERFORMANCE ANALYSIS OF MF DETECTOR FOR MIMO SPATIAL MULTIPLEXING 3221
lowest computational complexity detector among all practical
MIMO detectors studied in the literature [11], but at the
cost of severe performance degradation. Since the matched
filter operates such that the output SNR is maximized for
each transmit antenna and thus does not take into account
the interference caused by the symbols transmitted from the
other antennas, its performance without channel coding is
prohibitively poor in high SNR. Nevertheless, in the case
of low SNR, recent studies demonstrate that near optimal
performance can be achieved with the assistance of powerful
channel coding, and this is especially the case when the
number of antennas is large [22]. This fact has motivated
us to study the fundamental performance limit of the MF
detector in MIMO spatial multiplexing systems. Due to the
central limit theorem, the performance of the MF detector
can be easily analyzed in the framework of massive MIMO.
However, invoking such an approximation generally requires
the assumption of large antennas.
We note that the exact performance analyses for MIMO
and massive MIMO systems are still challenging, even for
simple cases such as those over uncorrelated Rayleigh fading
channels. The performances of linear detectors depend on
the signal-to-interference-plus-noise ratio (SINR) [23], and the
closed-form bit error rate (BER) expressions of the ZF detector
are discussed in [24], where the error rate performance and
outage probabilities of MMSE detector are also examined
based on the high SNR approximation. In [25], based on
the Gamma distribution approximation of the MMSE receiver
output, the approximate BER expression is derived. In [26],
on the other hand, the exact instantaneous BER (conditioned
on a channel realization) is derived in a closed form for a
general quadrature amplitude modulation (QAM) signaling
under the framework of MMSE detection, but in practice,
the average BER expression for a typical Rayleigh fading
scenario would be desirable if it is expressed by a simple
closed-form equation. Very recently, based on the assumption
of large number of receive antennas, the distribution of the
output of ZF detector and the resulting coded performance
have been derived in [27].
Since the ZF detector is able to remove all the interfering
symbols, its output contains only the two terms, i.e., signal
and noise. On the other hand, the MF detector should take
into account the correlation between the desired and interfering
symbols. Therefore, the exact analysis of MF detector would
be more complicated than that of ZF detectors. In [28],
the outage probability of the MF precoder is derived under
the framework of downlink multi-user massive MIMO, which
is shown to become accurate with a large number of anten-
nas. The exact expression of the outage probability for MF
precoder is also derived in the very recent work [29], but it
involves an expression with an infinite summation. Therefore,
the asymptotic forms are developed under the framework of
massive MIMO systems.
To the best of the authors’ knowledge, the exact and closed-
form expressions for uncoded BER under the framework of
MIMO spatial multiplexing with arbitrary numbers of transmit
and receive antennas, even with simple uncorrelated Rayleigh
fading channels, has not been well developed in the literature.
Therefore, in this work, we study the exact performance of
the MF detector without any restrictions on the numbers of
transmit and receive antennas. To this end, we first derive
the exact and closed-form probability distributions of the
MF detector output. We then derive the closed-form BER
expressions of uncoded MIMO systems with BPSK, QPSK,
and square-type M-ary QAM signaling with arbitrary numbers
of transmit and receive antennas. Our results can be seen as
the MIMO generalization of the MRC detector over Rayleigh
fading channels whose closed-form BER expressions are well
known in the case of single-input multiple-output (SIMO)
scenarios [30]. Furthermore, we derive an approximate cumu-
lative distribution function (cdf) when the number of antennas
becomes large. Using this result, we also derive the asymptotic
BER expression for QAM. The developed result can also be
used for the derivation of the optimal and closed-form log-
likelihood ratio (LLR) expression with the MF detector in
the case of coded MIMO systems. Furthermore, it enables
us to calculate the mutual information which serves as the
performance limit of the coded MIMO systems over ideally
interleaved channels. The results are confirmed by Monte-
Carlo simulations using a low-density parity-check (LDPC)
code [31], justifying our findings in this work. We will also
find that the optimal LLR expression based on the exact
metric developed here matches with that based on the simple
Gaussian distribution modeling, which may justify the use of
simple Gaussian-based metric for coded MIMO systems with
MF detector. Furthermore, considering the application of MF
detector to a more practical scenario, we extend our exact
analysis to the systems with imperfect channel estimation.
Our fundamental analytical framework will be built on
single-user MIMO systems where both the transmitter and
receiver can have an arbitrary number of antennas, but the
derived analytical results as well as the insight gained may be
applicable to the uplink of multi-user MIMO systems where
hundreds of antennas are placed at the base station (BS).
For such systems, the use of MF detector may substantially
simplify the complexity overhead of the signal processing
imposed for the BS. Our main objective is, through rigorous
theoretical analysis, to elucidate the conditions where the
MF detector may be beneficial in terms of the number of
antennas and channel SNR, for both uncoded and coded
cases.
This paper is organized as follows. In Section II, we
describe the system model adopted throughout this work.
The exact analysis of the MF detector output is performed
in Section III. The closed-form BER expressions in the
cases of uncoded BPSK, QPSK, and M-QAM are derived
in Section IV. Section V is devoted to the development of the
optimal metric for coded cases as well as the corresponding
mutual information, where the simulation results that support
the theoretical results are also provided. Our analysis is
extended to the practical scenario with channel estimation
error in Section VI. Finally, Section VII concludes this work.
Note that some very preliminary result focusing only on BPSK
signaling is presented in [32]. This work is its rigorous and
substantial extension including the exact analysis of QPSK and