没有合适的资源?快使用搜索试试~ 我知道了~
节点流行度和相似度对社交网络持久性的耦合效应
需积分: 10 1 下载量 63 浏览量
2021-05-11
09:19:29
上传
评论
收藏 1.28MB PDF 举报
温馨提示
网络健壮性代表网络承受故障和干扰的能力。 在社交网络中,维持个体活动(也称为持久性)对于理解健壮性非常重要。 先前的工作通常考虑对预先生成的网络结构的持久性; 而在社交网络中,网络结构随着现有个人的级联不活动而增长。 在这里,我们通过分析协同进化模型下的节点来应对这一挑战,该模型描述了三种网络增长模式下个体活动的变化:遵循节点受欢迎程度,相似性或均匀随机性的降序排列。 我们表明,当节点具有较高的自发活动时,流行度优先的增长模式会获得高度持久的网络;否则,在自发活动性较低的情况下,相似度优先的模式会更好。 此外,在短期内连续加入相似节点并混合几个高人气节点的复合增长模式,可获得最高的持久性。 因此,节点相似性对于持久性社交网络必不可少,而将流行度与相似性适当地结合起来则进一步优化了持久性。 这表明节点活动的发展不仅取决于网络拓扑,还取决于它们的连接类型。
资源推荐
资源详情
资源评论
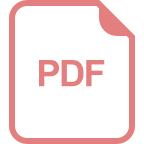
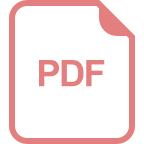
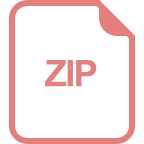
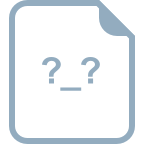
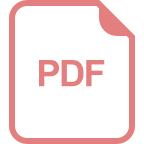
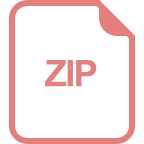
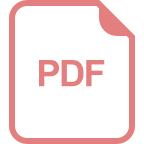
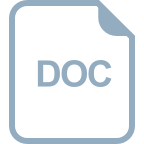
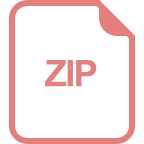
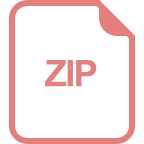
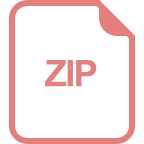
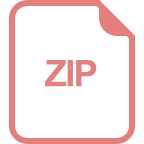
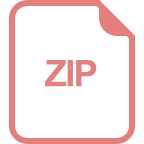
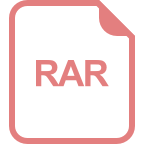
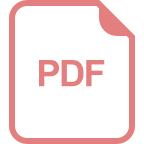
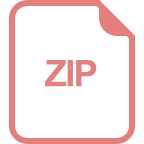
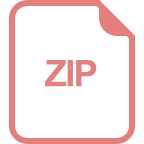
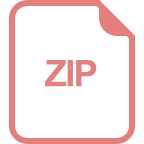
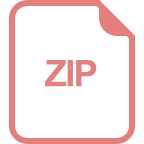
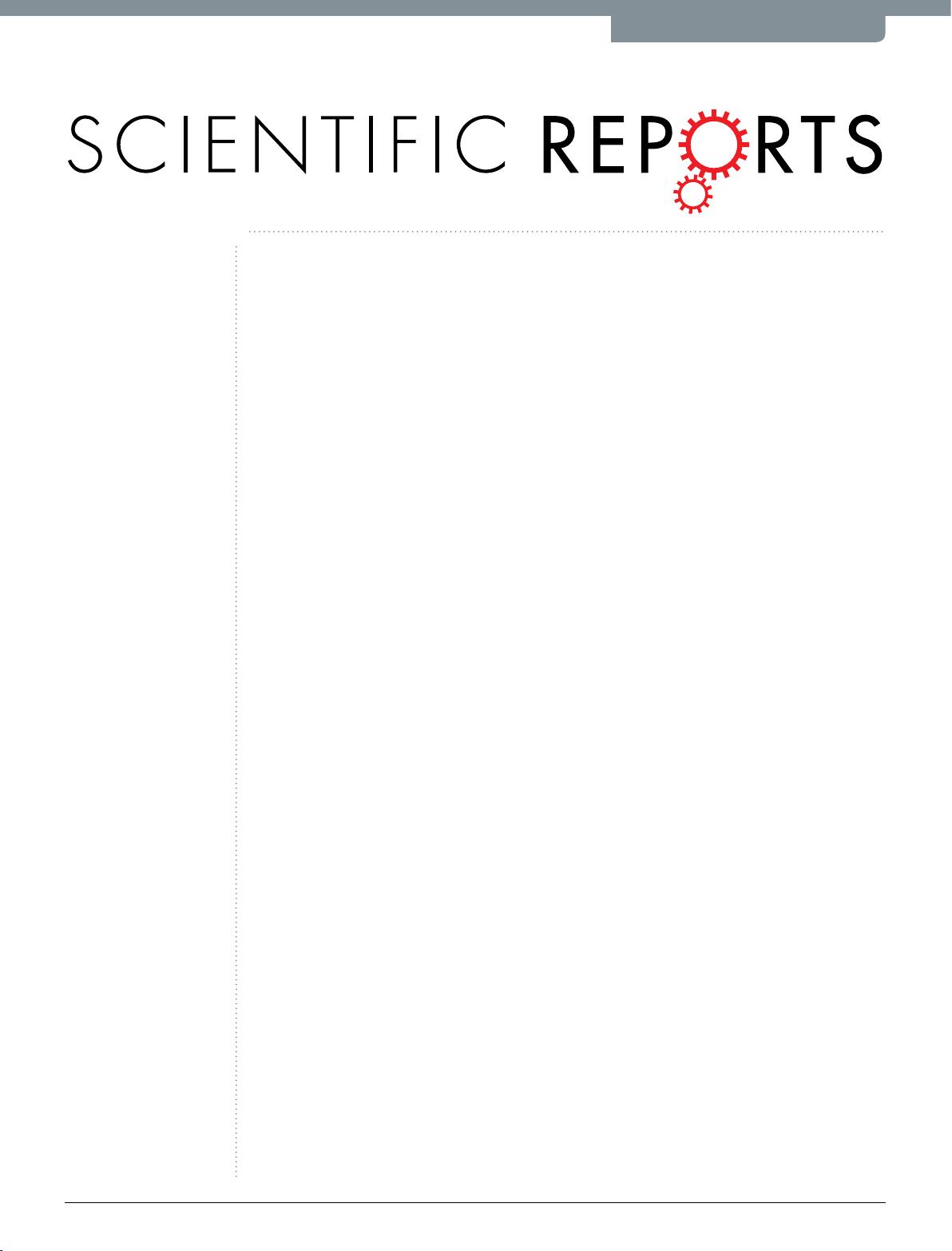
1
Scientific RepoRts | 7:42956 | DOI: 10.1038/srep42956
www.nature.com/scientificreports
Coupling eect of nodes popularity
and similarity on social network
persistence
Xiaogang Jin
1
, Cheng Jin
1
, Jiaxuan Huang
1
& Yong Min
2
Network robustness represents the ability of networks to withstand failures and perturbations. In
social networks, maintenance of individual activities, also called persistence, is signicant towards
understanding robustness. Previous works usually consider persistence on pre-generated network
structures; while in social networks, the network structure is growing with the cascading inactivity of
existed individuals. Here, we address this challenge through analysis for nodes under a coevolution
model, which characterizes individual activity changes under three network growth modes: following
the descending order of nodes’ popularity, similarity or uniform random. We show that when nodes
possess high spontaneous activities, a popularity-rst growth mode obtains highly persistent networks;
otherwise, with low spontaneous activities, a similarity-rst mode does better. Moreover, a compound
growth mode, with the consecutive joining of similar nodes in a short period and mixing a few high
popularity nodes, obtains the highest persistence. Therefore, nodes similarity is essential for persistent
social networks, while properly coupling popularity with similarity further optimizes the persistence.
This demonstrates the evolution of nodes activity not only depends on network topology, but also their
connective typology.
Network robustness is one of the core issues in network science
1–4
. Early research mainly focuses on static robust-
ness, i.e. the resilience of network connectivity to random errors or targeted attacks of components like nodes
or edges
5–7
. Furthermore, dynamics robustness or network persistence, which concerns about the ability of a
network to maintain certain states or functions, received major attention
8
. For example, in high voltage networks,
cascading failure is a common eect, where a single point of failure on a fully loaded or slightly overloaded system
results in a sudden spike across nearly all nodes of the system
9,10
. In food-webs, extinction of a certain tropic spe-
cies may cause a threat to the balance of the food-web, and the persistence of food-webs can be measured as the
fraction of initial species remaining at the end of a perturbation
11–14
. Similarly, in social networks, maintaining
individual activity is a key issue towards the overall welfare of the community
15
.
e real social networks possess three particular features, each of which may aect persistence of individual
activity. Firstly, diverse network growth modes. Social networks may grow under various modes like following
celebrities
16,17
, sharing the same hobbies
18,19
or uniformly random ways. Attraction comes from nodes popular-
ity or similarity
20
, and the heterogeneous node and link types may change network persistence even under the
same network structure
21,22
. Secondly, the cascading eects, i.e. inactivation of a node may cause its neighbors
turn inactive. Concepts like k-core and k-core decomposition are adopted to understand the function of network
structure on cascading eects in social networks
23–25
; besides, models initially describing cascading failures
26
in
power grids
7,9,27
or epidemic spreading
28,29
are reformed to understand dynamic cascading processes in social
networks, including rumor cascades
30,31
, inuence maximization
32–34
, viral marketing
35,36
, etc. irdly, unlike
power grids or stable food webs, the total number of nodes in social networks is in growth and the node state is
also changing
37,38
; however, the eect of the coevolution process brings to network persistence remains unclear.
erefore, social network persistence is an interesting and open issue.
Here, based on the three features in social networks, we integrate Papadopoulos’s
20
network growth model and
k-core
39
based cascading processes, which can characterize the coevolution of network growth with diverse
modes and the cascading process of node states. To display multiple growth modes of social networks, the
extended model contains four steps (See Fig.1). (1) generate n nodes under polar coordinates each with two
1
Institute of Articial Intelligence, College of Computer Science, Zhejiang University, Hangzhou, 310027, China.
2
College of Computer Science, Zhejiang University of Technology, Hangzhou, 310023, China. Correspondence and
requests for materials should be addressed to Y.M. (email: myong@zjut.edu.cn)
received: 04 October 2016
accepted: 17 January 2017
Published: 21 February 2017
OPEN
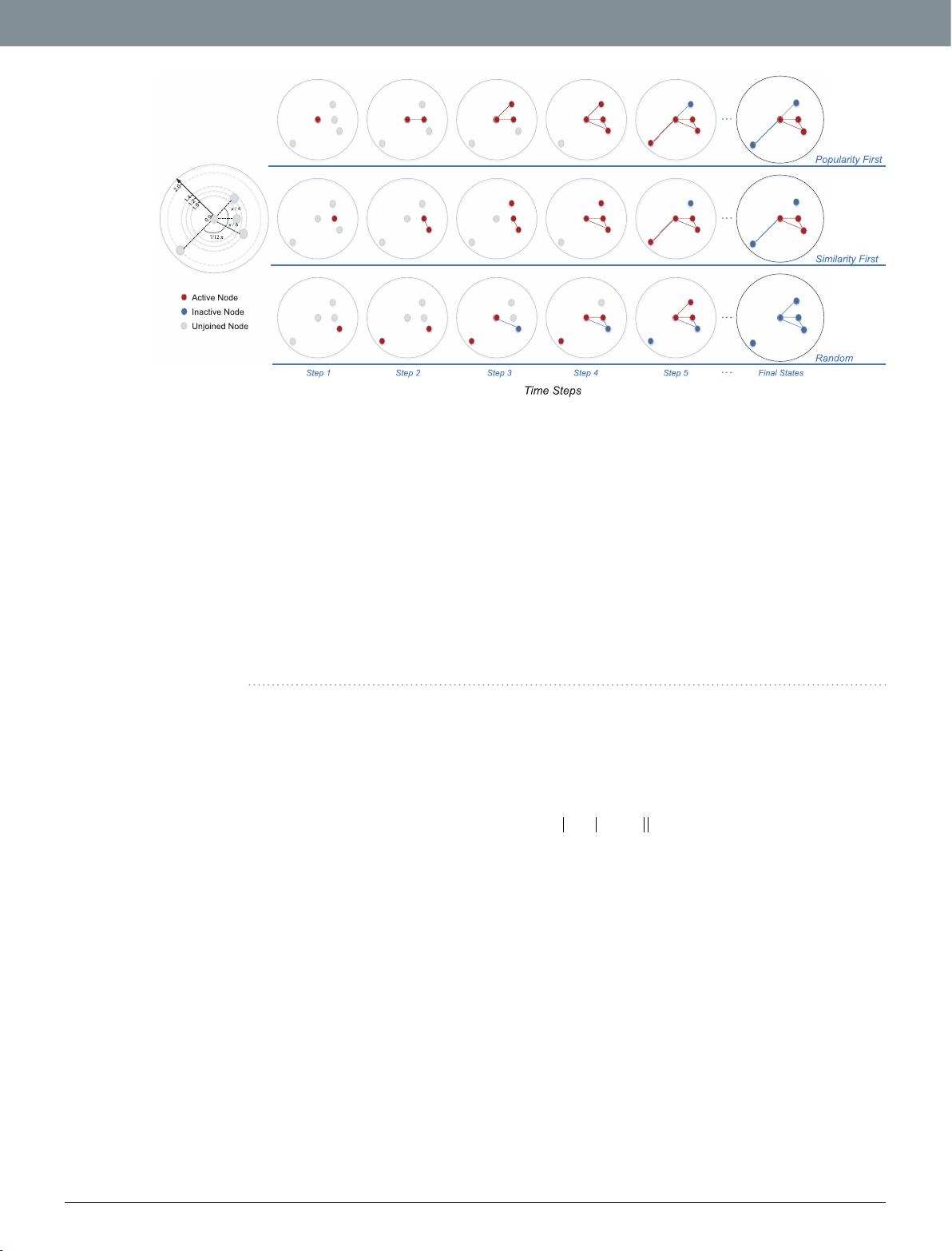
www.nature.com/scientificreports/
2
Scientific RepoRts | 7:42956 | DOI: 10.1038/srep42956
properties, the popularity of nodes (polar radius
==…riinln ,1,2,,
) and the similarity with other nodes
(random polar angle
θπ∈ [0,2 )
). (2) Initially, the network is empty and the adding order of nodes is dened,
which corresponds to the descending order of nodes’ popularity or nodes’ similarity, or is uniformly random (in
the following, we call them popularity-rst, similarity-rst and random network growth modes). (3) at time t, a
node v in order t is picked up and joins the network. (4) Node v connects to a subset of existing nodes S, consist-
ing of nodes with hyperbolic distance
θ=++≤Xrrrln(/2)
vs vs vs v
, which co-consider the pair nodes’ pop-
ularity and similarity with the central angle
θ ππθθ=− −−
vs vs
, and v should connect to no more than m
existing nodes that obtain the smallest X
vs
. Here, high popularity stands for small r and high similarity means a
small angle to
θ =
δ
0
. In this way, we inherit the connection rule introduced by Papadopoulos
20
, that is connec-
tions link to nodes with small hyperbolic distance, but we split the binding between node popularity and node
join time. e generated network still follows the preferential attachment mechanism, but the network growth
modes can be very exible.
To describe the coevolution of state cascading with the network growth, we introduce a cascading process
inspired from the denition of k-core. When joining in the network, each node has an initial setup of spontaneous
activity, say h, which is node’s ability to maintain active unconditionally; aer the spontaneous time, for each time
step, nodes with at least c active neighbors could keep active. Otherwise, they would turn inactive permanently.
For the demonstration in Fig.1, h is the length of time steps for nodes to remain unconditional active. For the
following results, for simplicity, h is a relative value of a total number of nodes and h ∈ (0%, 100%]. e idea of
active neighbor threshold c dates back to k-core or k-degenerate graph in graph theory. e network evolves until
all nodes are added, and states for each node never changes, and the persistence of complex social networks can
be quantied by the nal active ratio of nodes (FAR). Since active nodes satisfy active neighbor threshold c, FAR
can be seen as a variant measure of k-core in dynamic processes in which k = c.
We analyze the response of the proportion of durable, active nodes with dierent network growth modes, and
further study the coupling eects of popularity and similarity on the promotion of network persistence. e cou-
pling eect in this work means by a combined consideration of nodes popularity and similarity; the network may
obtain an enhanced or dierent persistence. Since the single growth modes (popularity-rst or similarity-rst)
based on one dimension of attraction reveal unique characters, we introduce a weighted score S
i
for nodes popu-
larity and similarity to combine the two preferential growth modes together to nd whether an optimized mixing
Figure 1. Geometric interpretation of network formation by nodes popularity and similarity, and
evolution of individual activity. e process is shown in polar coordinates. At each time step t, a node
v
i
= (r
i
,θ
i
) with the active state (red point) is added to the network and can connect to at most m existing nodes
(here m = 2) whose hyperbolic distance from v
i
is less than r
i
(i.e. within the green areas). If there are m′ > m
existing nodes within the green area of node v
t
, then v
t
preferentially links to the m nearest nodes regarding
hyperbolic distance. e hyperbolic distance between current node v
i
and an existing node v
j
is
θ++
()
rrln /2
ij ij
. An active node can remain in the active state unconditionally within h time steps (we call
this the spontaneous activity) since it was added. Aer the spontaneous activity, the node remains in the active
state on the condition that it has at least c active neighbours (we call this active neighbour thresholds, e.g. node 1
at time steps t = 3 and 4); otherwise, it changes to the inactive state (e.g. blue node 2 at time step t = 4) and
remains in this state indenitely thereaer. e order in which nodes are added can be freely chosen. In this
illustration, we use a demonstration of ve nodes to show the network growths and activity changes under
popularity-rst, similarity-rst and random growth modes. We set spontaneous activity h = 2 and active
neighbour threshold c = 2 both as absolute values here. But the following discussion adopts the relative value
within range [0%, 100%] for simplication, and h is a relative value of total number of nodes, c is a relative value
of m.
剩余7页未读,继续阅读
资源评论
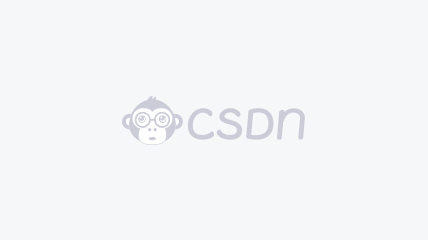

weixin_38595690
- 粉丝: 6
- 资源: 942
上传资源 快速赚钱
我的内容管理 展开
我的资源 快来上传第一个资源
我的收益
登录查看自己的收益我的积分 登录查看自己的积分
我的C币 登录后查看C币余额
我的收藏
我的下载
下载帮助

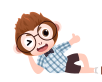
最新资源
- 毕设和企业适用springboot企业数据智能分析平台类及智能农场管理系统源码+论文+视频.zip
- 毕设和企业适用springboot企业数据智能分析平台类及智能农业解决方案源码+论文+视频.zip
- 毕设和企业适用springboot企业协作平台类及数字图书馆平台源码+论文+视频.zip
- 毕设和企业适用springboot企业协作平台类及网络营销平台源码+论文+视频.zip
- 毕设和企业适用springboot企业协作平台类及个性化广告平台源码+论文+视频.zip
- 毕设和企业适用springboot企业协作平台类及产品溯源系统源码+论文+视频.zip
- 毕设和企业适用springboot企业数据智能分析平台类及资源调度平台源码+论文+视频.zip
- 毕设和企业适用springboot企业协作平台类及团队协作平台源码+论文+视频.zip
- 毕设和企业适用springboot企业协作平台类及无人驾驶系统源码+论文+视频.zip
- 毕设和企业适用springboot企业协作平台类及业务流程自动化平台源码+论文+视频.zip
- 毕设和企业适用springboot企业协作平台类及销售管理平台源码+论文+视频.zip
- 毕设和企业适用springboot企业协作平台类及客户关系管理平台源码+论文+视频.zip
- 毕设和企业适用springboot企业协作平台类及共享经济平台源码+论文+视频.zip
- 毕设和企业适用springboot企业协作平台类及客户服务平台源码+论文+视频.zip
- 毕设和企业适用springboot企业协作平台类及跨平台销售系统源码+论文+视频.zip
- 毕设和企业适用springboot企业协作平台类及平台生态系统源码+论文+视频.zip
资源上传下载、课程学习等过程中有任何疑问或建议,欢迎提出宝贵意见哦~我们会及时处理!
点击此处反馈


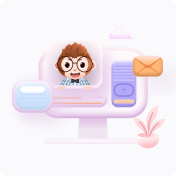
安全验证
文档复制为VIP权益,开通VIP直接复制
