没有合适的资源?快使用搜索试试~ 我知道了~
Convergence of gradient method with penalty for Ridge Polynomial...
0 下载量 156 浏览量
2021-02-21
04:57:40
上传
评论
收藏 504KB PDF 举报
温馨提示
In this paper, a penalty term is added to the conventional error function to improve the generalization of the Ridge Polynomial neural network. In order to choose appropriate learning parameters, we propose a monotonicity theorem and two convergence theorems including a weak convergence and a strong convergence for the synchronous gradient method with penalty for the neural network. The experimental results of the function approximation problem illustrate the above theoretical results are valid.
资源推荐
资源详情
资源评论
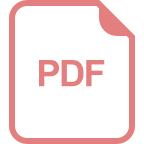
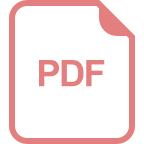
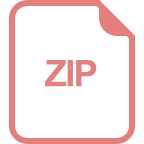
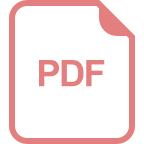
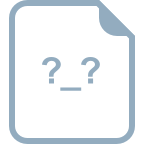
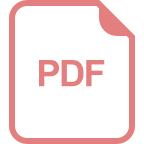
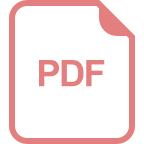
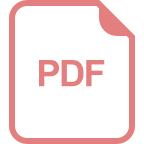
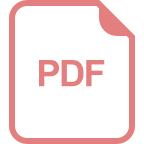
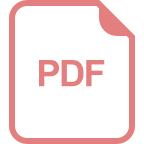
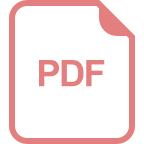
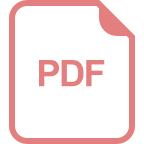
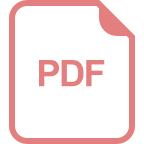
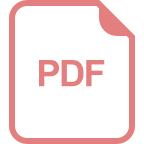
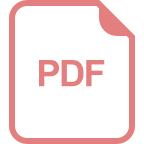
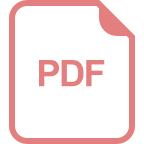
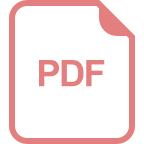
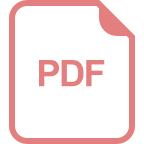
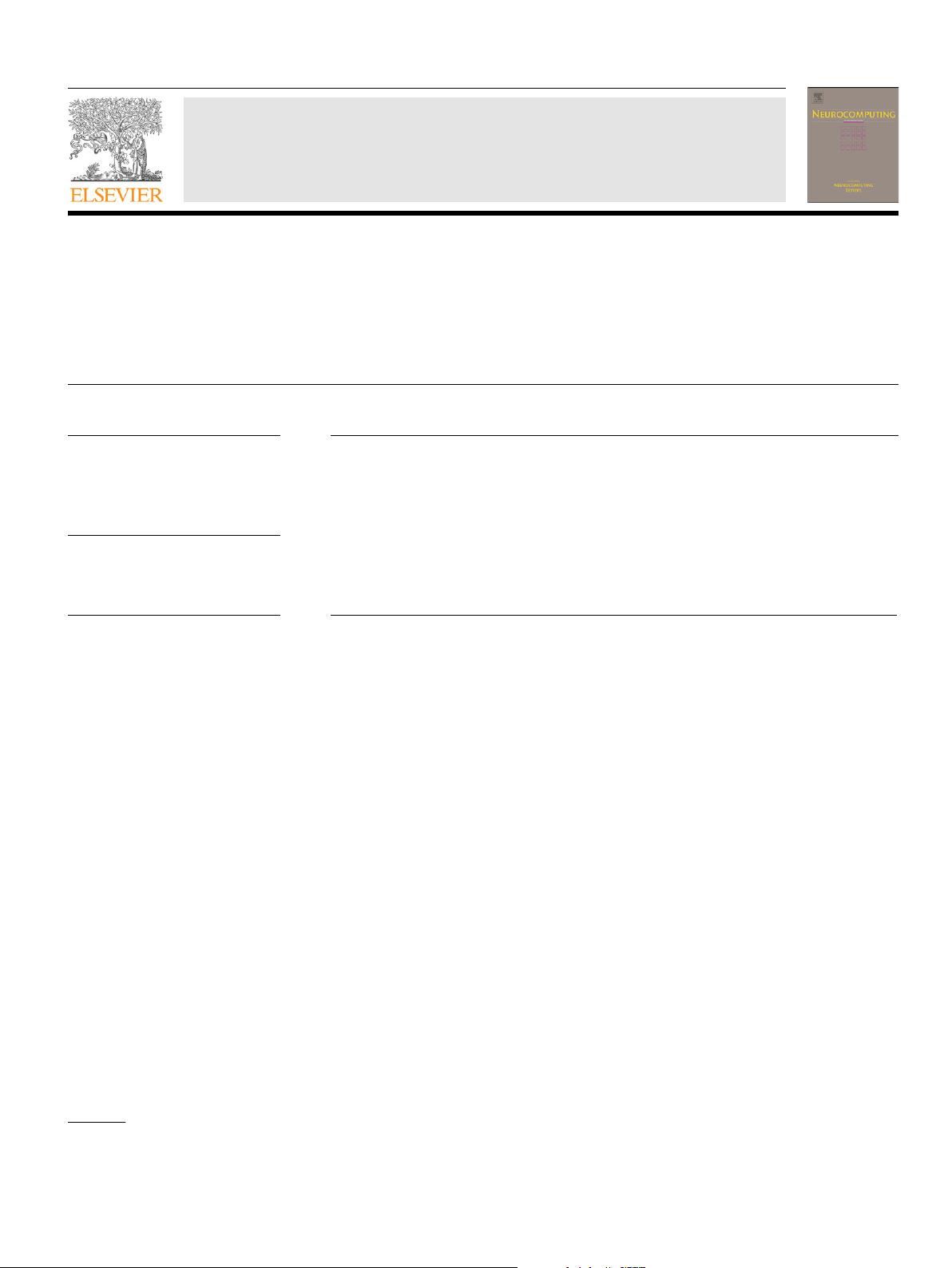
Letters
Convergence of gradient method with penalty for Ridge Polynomial
neural network
Xin Yu
n
, Qingfeng Chen
School of Computer, Electronics and Information, Guangxi University, Nanning 53004, China
article info
Article history:
Received 2 December 2011
Received in revised form
24 April 2012
Accepted 28 May 2012
Communicated by R.W. Newcomb
Available online 3 July 2012
Keywords:
Ridge Polynomial neural network
Gradient algorithm
Monotonicity
Convergence
abstract
In this paper, a penalty term is added to the conventional error function to improve the generalization
of the Ridge Polynomial neural network. In order to choose appropriate learning parameters, we
propose a monotonicity theorem and two convergence theorems including a weak convergence and a
strong convergence for the synchronous gradient method with penalty for the neural network. The
experimental results of the function approximation problem illustrate the above theoretical results
are valid.
& 2012 Elsevier B.V. All rights reserved.
1. Introduction
The pi–sigma(
Q
–
P
) neural network (PSNN) [1] isatypeof
high-order feedforword neural network, which uses t he product of
the sums of the input units. This network has a powerful function
approximation ability while avoiding the combinatorial explosion of
the higher terms. However, it is not an universal approximator. The
Ridge Polynomial neural network (RPNN) [2] is a generalization of
PSNN and uses a number of PSNNs as its basic building blocks. It
maintains the fast learning ability of the PSNN and can uniformly
approximate any continuous function on a compact set in R
d
[3].
Thus, the RPNN has been widely used in various fields [4–6].
The synchronous gradient method is a commonly used train-
ing algorithm for higher-order neural networks, and some con-
vergence theorems of gradient methods for various neural
networks have been proposed [7–13]. The study on the conver-
gence of training method is important for choosing appropriate
training parameters, e.g., initial weights and learning rate, to
perform an effective network training. Moreover, the general-
ization of neural networks is a critical issue in designing a training
method for neural networks. To improve the generalization of
neural networks, one widely used scheme is adding a penalty
term proportional to the magnitude of network weights to the
conventional error function [10–13]. Thus, this article aims to
study the convergence of synchronous gradient method with
penalty for the RPNN. We first prove that the error sequence will
be uniformly monotonous and the algorithm also will be weakly
convergent during the training procedure on condition that
certain assumptions (cf. Assumptions A1–A3) are satisfied, then
prove that if the error function has finite stable points, the
algorithm with the weak convergence will be strongly conver-
gent. In the end, the training method is applied to a function
approximation problem to illustrate our theoretical finding.
2. Preliminary
Since the Ridge Polynomial neural network mainly consists of
some Pi–Sigma neural networks, the structure of the Pi–Sigma
neural network is introduced below.
2.1. Pi–sigma neural network
Fig. 1 shows a Pi–Sigma neural network with a single output.
Let nþ1, k and 1 be the number of the input units, summing units
and output units of the PSNN, respectively. Denote by w
j
¼ðw
j0
,
w
j1
, ..., w
jn
Þ
T
A R
n þ1
the weight vector from the summing unit j to
the input units, and write w ¼ðw
1
, w
2
, ..., w
k
Þ
T
A R
ðn þ1Þk
. Note that
the weights from summing units to product unit are fixed to 1. Let
x ¼ðx
0
, x
1
, ..., x
n
Þ
T
A R
n þ1
be nþ1 dimensional input vector where
x
0
¼1 is an adjustable threshold. Further, let y denote the output
of the product unit, given by
y ¼
s
Y
k
j ¼ 1
X
n
i ¼ 0
w
ji
x
i
!
0
@
1
A
¼
s
Y
k
j ¼ 1
w
j
, x
0
@
1
A
ð1Þ
Contents lists available at SciVerse ScienceDirect
journal homepage: www.elsevier.com/locate/neucom
Neurocomputing
0925-2312/$ - see front matter & 2012 Elsevier B.V. All rights reserved.
http://dx.doi.org/10.1016/j.neucom.2012.05.022
n
Corresponding author.
E-mail addresses: yuxin21@126.com (X. Yu), qingfeng@gxu.edu.cn (Q. Chen).
Neurocomputing 97 (2012) 405–409
资源评论
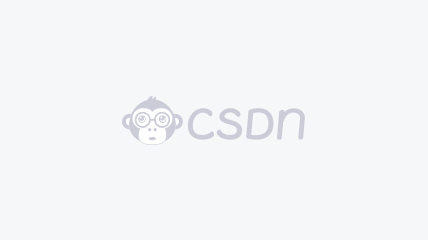

weixin_38565631
- 粉丝: 2
- 资源: 913
上传资源 快速赚钱
我的内容管理 展开
我的资源 快来上传第一个资源
我的收益
登录查看自己的收益我的积分 登录查看自己的积分
我的C币 登录后查看C币余额
我的收藏
我的下载
下载帮助

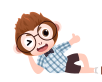
最新资源
- 使用漂移和扩散模型模拟pn二极管中的电流和电压的小型MATLAB脚本.rar
- 使用混合模仿强化学习架构的自主赛车Matlab代码.rar
- 使用漂移扩散解算器求解有机器件中的一维静电方程 matlab代码.rar
- 探索在星座上方的高度使用全球导航卫星系统的可行性Matlab代码.rar
- 图像融合评估的仓库,、Qabf、CC、SCD、Nabf、Qcv.rar
- 通过稀疏有界平方和优化可证明的全局最优单位四元数旋转平均 matlab代码.rar
- Matlab基于LSTM长短期记忆神经网络的锂电池寿命预测(含完整的程序,GUI设计和代码详解)
- 特定任务的 DBF(Design Build Fly)竞赛制作的无人机附matlab代码.rar
- 无人机飞行动力学和控制相关Matlab代码 matlab代码.rar
- python线程、队列等应用示例
- 无人机地面站和模拟器附matlab代码.rar
- 无人机道路裂缝检测附matlab代码 matlab代码.rar
- 无人机飞行控制系统模型SIMULINK代码 matlab代码.rar
- 无人机辅助边缘计算python代码.rar
- 无人机浮标系统的MATLAB Simulink实现.rar
- 无人机辅助移动边缘计算的计算卸载优化:一种深度确定性策略梯度方法python代码.rar
资源上传下载、课程学习等过程中有任何疑问或建议,欢迎提出宝贵意见哦~我们会及时处理!
点击此处反馈


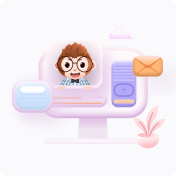
安全验证
文档复制为VIP权益,开通VIP直接复制
