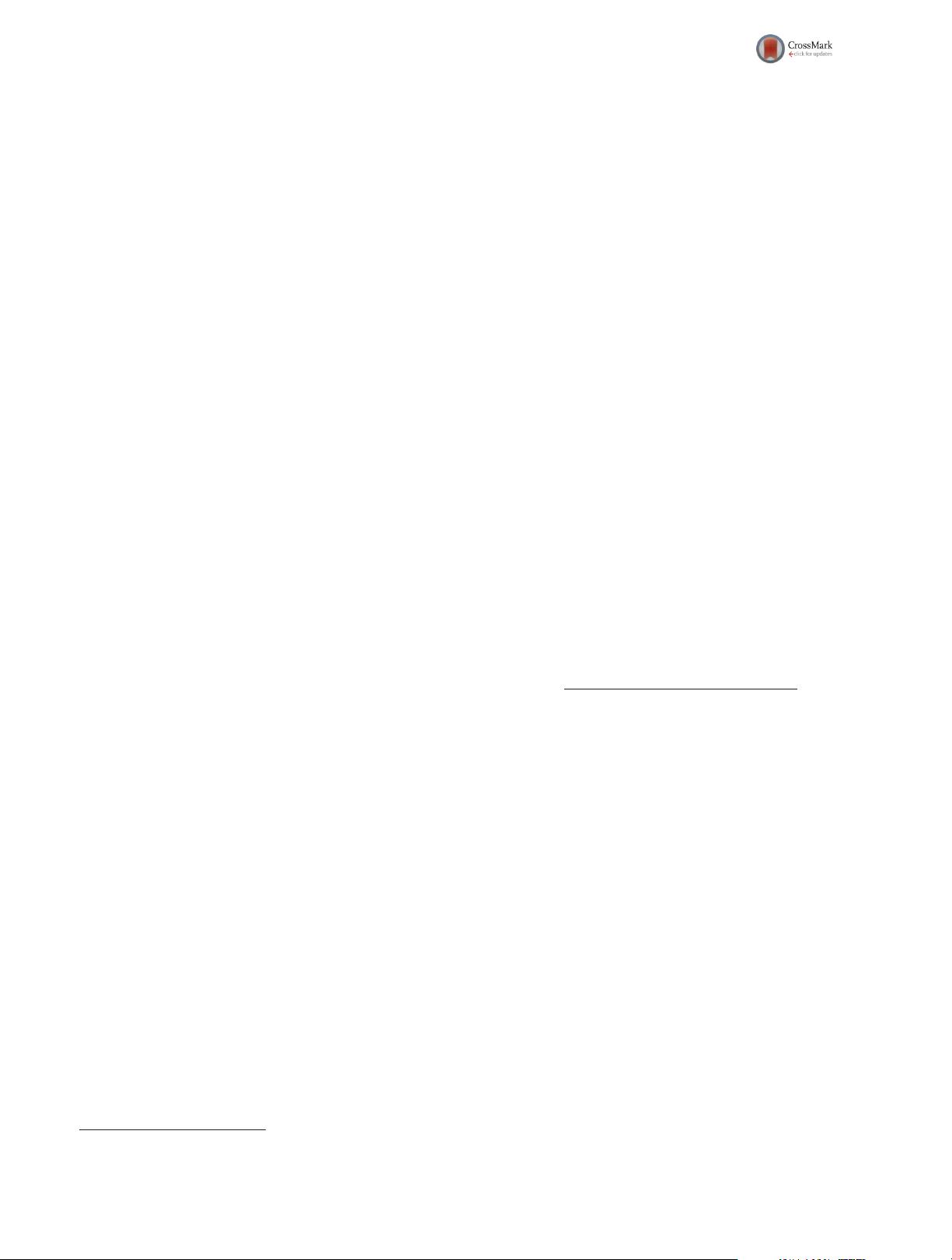
Effects of bursting dynamic features on the generation of multi-clustered
structure of neural network with symmetric spike-timing-dependent
plasticity learning rule
Hui Liu,
1,2
Yongduan Song,
1,2
Fangzheng Xue,
1,2
and Xiumin Li
1,2,a)
1
Key Laboratory of Dependable Service Computing in Cyber Physical Society of Ministry of Education,
Chongqing University, Chongqing 400044, China
2
College of Automation, Chongqing University, Chongqing 400044, China
(Received 8 May 2015; accepted 21 October 2015; published online 13 November 2015)
In this paper, the generation of multi-clustered structure of self-organized neural network with
different neuronal firing patterns, i.e., bursting or spiking, has been investigated. The initially all-
to-all-connected spiking neural network or bursting neural network can be self-organized into clus-
tered structure through the symmetric spike-timing-dependent plasticity learning for both bursting
and spiking neurons. However, the time consumption of this clustering procedure of the burst-
based self-organized neural network (BSON) is much shorter than the spike-based self-organized
neural network (SSON). Our results show that the BSON network has more obvious small-world
properties, i.e., higher clustering coefficient and smaller s hortest path length than the SSON net-
work. Also, the results of larger structure entropy and activity entropy of the BSON network dem-
onstrate that this network has higher topological complexity and dynamical diversity, which
benefits for enhancing information transmission of neural circuits. Hence, we conclude that the
burst firing can significantly enhance the efficiency of clustering procedure and the emergent clus-
tered structure renders the whole network more synchronous and therefore more sensitive to weak
input. This result is further confirmed from its improved performance on stochastic resonance.
Therefore, we believe that the multi-clustered neural network which self-organized from the burst-
ing dynamics has high efficiency in information processing.
V
C
2015 AIP Publishing LLC.
[http://dx.doi.org/10.1063/1.4935281]
Many neurophysiological experiments have investigated
that most of neurons in our brain are always connected
in multi-clustered structures with small-world-like prop-
erty. Thus, it is advised to consider the generation of
multi-clustered structure of neural network based on
neurophysiologically observed learning rules rather than
artificially defined algorithms, as neuronal connectivity
in the brain is flexible and perpetually changing.
Meanwhile, the evolution of network structure is essen-
tially affected by the dynamical state of the network.
However, how different firing dynami cs affect the emer-
gence of multi-clustered neural network has not been
fully investigated. In this paper, we investigate the gener-
ation of multi-clustered neural network with symmetric
spike-timing-dependent plasticity based on two firing pat-
terns: spiking or bursting. We find that burst-based self-
organized neural network (BSON) has more obvious
small-word prop erties than spike-based self-organized
neural network (SSON) by analyzing the clustering coef-
ficient and shortest path length. Moreover, structure en-
tropy and activity entropy is discussed to confirm that
BSON network has higher topological complexity and dy-
namical diversity, which benefits for enhancing informa-
tion transmission of neural circuits. This result may have
important implications on understanding how the brain
network is able to work with high efficiency in
information processing, i.e., by choosing neuronal firing
patterns and potential functions of network learning.
I. INTRODUCTION
Anatomists have found that most connections among
neurons are local in most brain structures, and the majority
of local connections are supplemented by long-range con-
nections. That is, cortical networks are multi-clustered
groups of linked areas where areas within a group form more
connections among each other than with areas in other parts
of the network.
1
For the huge populations of neurons in the
human cortex, two organization principles local clustering
and global connectivity between distant parts of the brain are
necessary for the efficient large-scale communication with a
minimum amount of synaptic connections.
2,3
The existence
of multi-clustered structure with small-world-like property in
the brain has been strongly supported by many neurophysio-
logical experiments.
4
The long-range connections in multi-
clustered neural networks occupy quite a s mall portion of
neural connectivity but have much contribution to the short
synaptic path length, i.e., minimize the cost of connectivity.
Basically, two methods for the generation of multi-
clustered neural network have been put forward. One is a
spatial growth algorithm where the probability for the edge
formation depends on the spatial-distance between nodes,
5
and another is a cortex-like network generation method
a)
Electronic mail: xmli@cqu.edu.cn
1054-1500/2015/25(11)/113108/7/$30.00
V
C
2015 AIP Publishing LLC25, 113108-1
CHAOS 25, 113108 (2015)