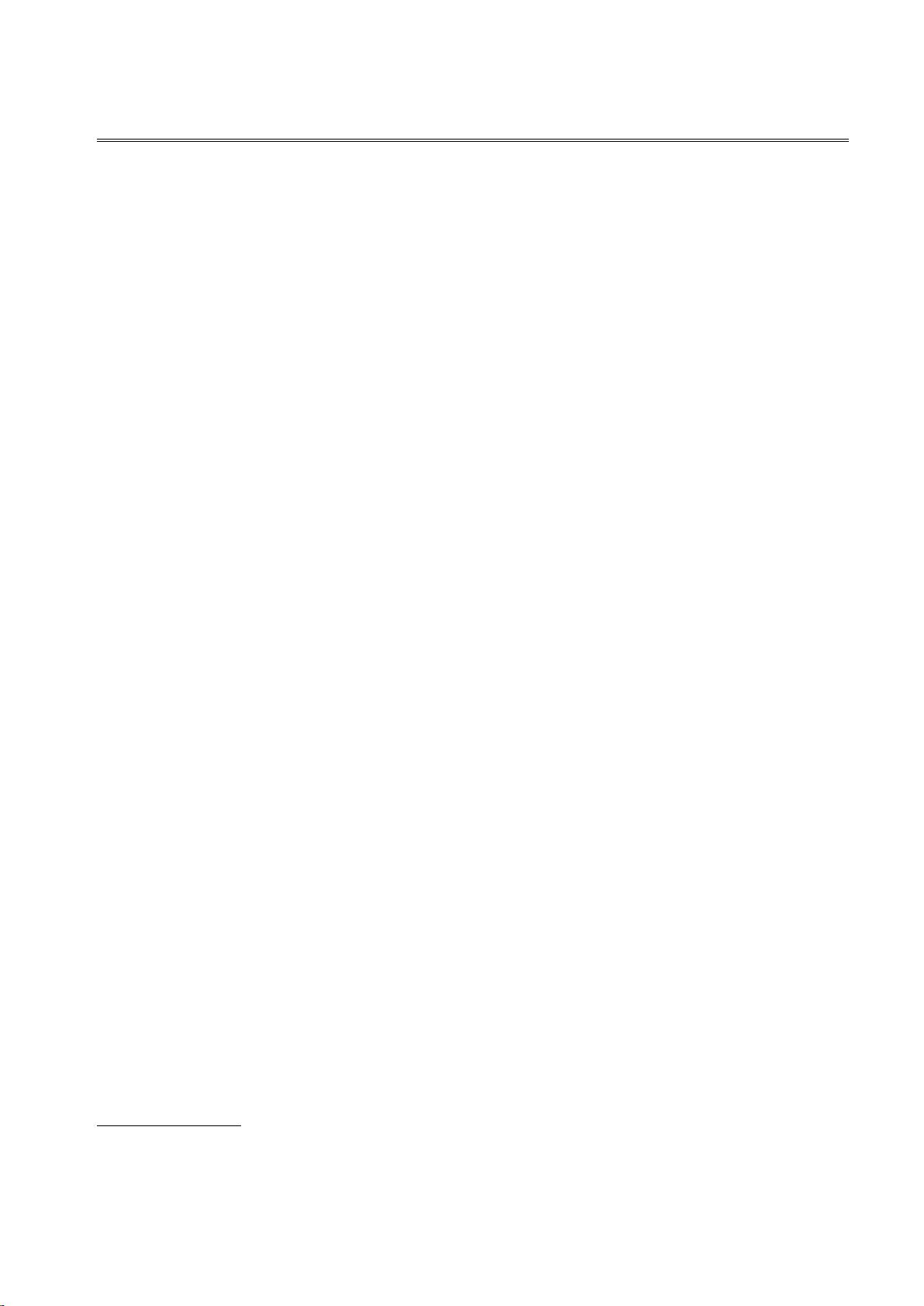
33 10 ᳳ
2016 ᑈ 10 ᳜
ࣅᄥৢူဈ
Control Theory & Applications
Vol. 33 No. 10
Oct. 2016
∖∖∖㾷㾷㾷ᯖᯖᯖ䌉䌉䌉䯈䯈䯈ⳂⳂⳂӬӬӬ࣪࣪࣪䯂䯂䯂乬乬乬ⱘⱘⱘ催催催ᮃᮃᮃҷҷҷ⧚⧚⧚ൟൟൟ
DOI: 10.7641/CTA.2016.50398
䰜ᖫᯎ
1,2
, ⱑ䫠
1†
, ᴼϗ
1
, 咘݈ᯎ
1
, ᴢᔎ
1
(1. ➩ቅᄺ ᎹϮ䅵ㅫᴎࠊᎹ⊇࣫ⳕ䞡⚍ᅲ偠ᅸ, ⊇࣫ ⾺ⱛቯ 066004;
2. ➩ቅᄺ ᆊދ䔻ᵓᏺ㺙ঞᎹ㡎ᎹᡔᴃⷨおЁᖗ, ⊇࣫ ⾺ⱛቯ 066004)
ᨬ㽕: ᴀ᭛ᇚ᭄ᣪᥬ(催ᮃ䖛ಲᔦᓎ)ᱎ㛑䖯࣪ㅫ⊩(GA, NSGA–II)䖯㸠㒧ড়, ⫼Ѣ㾷އӬ࣪ߑ᭄ⶹⱘ
ᯖ䌉䯈ⳂӬ࣪䯂乬. 佪ܜ߽⫼催ᮃ䖛ᇍ䞛⫼Ё⚍ϡ⹂ᅮᑺ㸼⼎ⱘⶹⳂߑ᭄㑺ᴳߑ᭄䖯㸠ᓎ, ⬅
ѢⳌ݇ᗻޚ⹂ᗻᰃ䯈ߑ᭄ൟⱘϸϾᖙᴵӊ, ᬙᦤߎϔ⾡㵡ড়ሲᗻއㄪⱘঠሖ⾡㕸ㄯ䗝ㄪ⬹, ᑊᇚ݊ጠܹ
ࠄ䘫Ӵㅫ⊩∖㾷催ᮃൟখ᭄ⱘ䖛Ё, 1ሖḍⳌ݇ᗻሲᗻᥦ䰸䗝㾷䲚Ё䚼ߚࡷ㾷, 2ሖḍޚ⹂ᗻሲᗻᥦ
䰸䗝㾷䲚Ё݊ԭ䍙ߎ⾡㕸㾘ⱘࡷ㾷, ϸሲᗻⱘᴗ䞡㋏᭄އᅮϸሖᥦ䰸ࡷ㾷ⱘ↨՟. ✊ৢᇚ᠔ᓎൟЎӬ࣪
ᇍ䈵ⱘҷ⧚ൟᓩᇐ䯈NSGA–IIㅫ⊩Ӭ࣪∖㾷, Ң㗠㦋ᕫ᠔䳔ⱘParetoࠡ⊓.
݇䬂䆡: ⳂӬ࣪; 䯈㾘ߦ; 2ҷ䴲ᬃ䜡ᥦᑣ䖯࣪ㅫ⊩(NSGA–II); 催ᮃ䖛; ሲᗻއㄪ; ҷ⧚ൟ
Ёߚ㉏ো: TP301 ᭛⤂䆚ⷕ:A
Gaussian surrogate models for expensive interval multi-objective
optimization problem
CHEN Zhi-wang
1,2
, BAI Xin
1†
, YANG Qi
1
, HUANG Xing-wang
1
, LI Guo-qiang
1
(1. Key Lab of Industrial Computer Control Engineering of Hebei Province, Yanshan University, Qinhuangdao Hebei 066004, China;
2. National Engineering Research Center for Equipment and Technology of Cold Strip Rolling,
Yanshan University, Qinhuangdao Hebei 066004, China)
Abstract: In this paper data mining (Gaussian process regression modeling) and intelligent evolutionary algorithm (GA,
NSGA–II) are combined to solve the expensive interval multi-objective optimization problem with unknown optimization
functions. Firstly, Gaussian process (GP) is used to model the objective functions and constraint functions represented by
the midpoint and uncertainty. Because relevance and accuracy are two essential factors of interval function models, A kind
of double steps screening strategy based on multiple attribute decision making (MADM) is proposed and it is embedded
into the genetic algorithm to identify the parameters of the GP model. In the first step, inferior solutions in candidate
solutions are excluded according to relevance. In the second step, the rest of inferior solutions beyond population quantity
are excluded according to accuracy. And the proportion of inferior solutions excluded in the two steps is decided by the
weight coefficient of two factors. Then, the built GP models for optimization objects are used as surrogate models in the
NSGA--II optimization algorithm, so that Pareto front can be found.
Key words: multi-objective optimization; interval programming; non-dominated sorting genetical agorithm II (NSGA--
II); Gaussian process; multiple attribute decision making; surrogate model
1 ᓩᓩᓩ㿔㿔㿔(Introduction)
䱣ⴔᄺ㗙ӀᇍⳂӬ࣪䯂乬ⱘⷨお, ᯖ䌉䯈
ⳂӬ࣪䯂乬៤ЎᔧҞⳂӬ࣪ⱘⷨお⛁⚍. 䆹
䯂乬ϡҙࣙњ䯈ⳂӬ࣪ᯖ䌉ⳂӬ࣪
ⱘ䲒⚍, 㗠Ϩ⬅Ѣϸ䯂乬ጠড়, Փᕫ∖㾷ᯊ䳔ѦⳌݐ
乒, Ң㗠ࡴ⏅њ䯂乬ⱘӬ࣪䲒ᑺ. ݊Ё䯈ⳂӬ
࣪ᣛⱘᰃӬ࣪䯂乬ЁⳂߑ᭄㑺ᴳߑ᭄᳝ϡ⹂
ᅮ䯈ব䞣
[1]
; 㗠ᯖ䌉ⳂӬ࣪ᣛⱘᰃӬ࣪䯂乬㊒
⹂᭄ᄺൟ䲒ҹ㦋ᕫϨϢӬ࣪ᇍ䈵䖯㸠䆘Ԅᅲ偠ⱘ
៤ᴀ催ᯖ, 䞣ⱘ䆘Ԅᅲ偠ᖙ✊Ӯ䗴៤៤ᴀ♒䲒
[2–3]
.
ᇍѢᯖ䌉ⳂӬ࣪䯂乬, 䖥ᑈᴹᄺ㗙Ӏᇚ᭄
ᣪᥬⱘᗱᛇᓩܹࠄᱎ㛑䖯࣪乚ඳ
[4]
. ݊Џ㽕ᗱ䏃ᰃ߽
⫼ಲᔦㅫ⊩ᇍӬ࣪ᇍ䈵ⱘ㒣偠᭄(㒣偠᭄⬅㾷ঞ
݊᠔ᇍᑨⱘⳳᅲⳂ䞣㒘៤)䖯㸠ᄺд䆁㒗㗠䕼䆚
ᬊ〓᮹ᳳ: 2015−05−13; ᔩ⫼᮹ᳳ: 2016−09−05.
†
䗮ֵ㗙. E-mail: 15233013272@163.com; Tel: +86 15233013272.
ᴀ᭛䋷ӏ㓪ྨ: ߬ϕ.
ᆊ㞾✊⾥ᄺ䞥(61403331, 61573305), ⊇࣫ⳕ㞾✊⾥ᄺ䞥䴦ᑈ䞥(F2014203099), ➩ቅᄺ䴦ᑈᬭᏜ㞾Џⷨお䅵ߦ䇒乬(13LGA006)䌘ࡽ.
Supported by National Natural Science Foundation of China (61403331, 61573305), Natural Science Foundation for Young Scientist of Hebei Pro-
vince, China (F2014203099) and Independent Research Program for Young Teachers of Yanshan University, China (13LGA006).