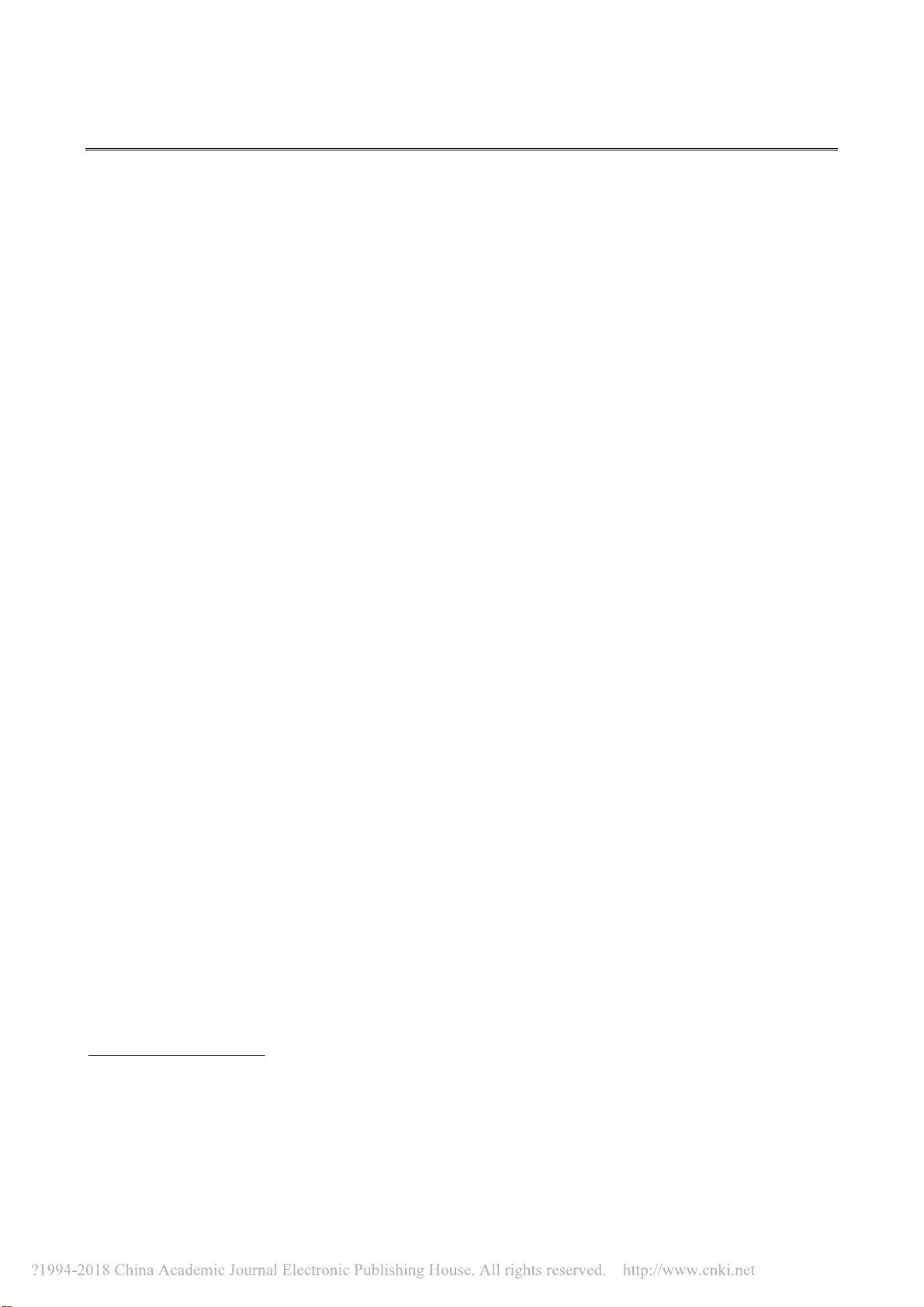
第 31 卷 第 31 期 中 国 电 机 工 程 学 报 Vol.31 No.31 Nov.5, 2011
102 2011 年 11 月 5 日 Proceedings of the CSEE ©2011 Chin.Soc.for Elec.Eng.
文章编号:
0258-8013 (2011) 31-0102-07
中图分类号:
TM 71
文献标志码:
A
学科分类号:
470
⋅
40
基于经验模态分解和支持向量机的
短期风电功率组合预测模型
叶林,刘鹏
(中国农业大学信息与电气工程学院,北京市 海淀区 100083)
Combined Model Based on EMD-SVM for Short-term Wind Power Prediction
YE Lin, LIU Peng
(College of Information and Electrical Engineering, China Agricultural University, Haidian District, Beijing 100083, China)
ABSTRACT: A wind power prediction method based on
empirical mode decomposition (EMD) and support vector
machine (SVM) is proposed to treat with the nonlinearity and
nonstationarity of wind speed data. Firstly, the wind speed data
is decomposed into a series of components with stationarity by
using EMD to reduce the influence between different feature
information. Then, different models were built and different
kernel functions and parameters were chosen to deal with each
group of data by using SVM in order to improve the
forecasting accuracy. Finally, short term wind power
forecasting was made based on wind speed data through a
practical wind power curve. Case study was carried out to
investigate the validity of the novel algorithm and the results
illustrated that the forecasting error of EMD-SVM combined
model decreased by 5%~10% compared to single statistics
model. The proposed combined model can improve the short
term forecasting accuracy of wind power effectively by
tracking the change of wind power.
KEY WORDS: empirical mode decomposition (EMD);
support vector machine (SVM); wind speed; short-term wind
power prediction; combined forecasting model
摘要:针对风速序列随时间、空间呈现非平稳性变化的特征,
提出一种基于经验模态分解(empirical mode decomposition,
EMD)和支持向量机(support vector machine,SVM)的
EMD-SVM 短期风电功率组合预测方法。该方法首先利用
基金项目:国家自然科学基金项目(51077126,51174290);教育部
科学技术重点研究项目(109017);教育部新世纪优秀人才支持计划
(NCET-08-0543);北京市自然科学基金项目(3113029)。
Project Supported by National Natural Science Foundation of China
(51077126, 51174290); Key Project of Chinese Ministry of Education
(109017); Program for New Century Excellent Talents in University
(NCET-08-0543); Beijing Natural Science Foundation (3113029).
EMD 将风速序列分解为一系列相对平稳的分量,以减少不
同特征信息间的相互影响;然后利用 SVM 法对各分量建立
预测模型,针对各序列自身特点选择不同的核函数和相关参
数来处理各组不同数据,以提高单个模型预测精度。最后将
风速预测结果叠加并输入功率转化曲线以得到风电功率预
测结果。研究结果表明,EMD-SVM 组合预测模型能更好地
跟踪风电功率的变化,其预测误差比单一统计模型降低了
5%~10%,有效地提高了短期风电功率预测的精度。
关键词:经验模态分解
;支持向量机;风速;短期风电功
率预测;组合预测模型
0 引言
随着风电技术的不断发展和风电场的规模不
断增大,为了保证电力系统的稳定运行和供电可靠
性,必须对风电系统进行有效的规划和调度
[1-2]
。由
于风电本身所特有的间歇性和不确定性,增加了电
网调度的难度,为了解决风电场的发电量不确定问
题,电网必须提供足够的旋转备用容量,而旋转备
用容量的增加间接地增加了风力发电的整体运营
成本,所以需要对风电场的输出功率进行预测,通
过对风电场发电量进行准确的预测,可以大幅降低
电网旋转备用容量,从而有效降低风力发电系统的
运行成本,为电网调度运行提供可靠依据
[3-5]
。
目前常用的风电功率预测方法包括持续法、时
间序列法、卡尔曼滤波法、人工神经网络法、模糊
逻辑法、支持向量机法、空间相关性法、物理预测
法等
[6-8]
。其中持续法认为风速预测值等于最近几个
风速历史值的滑动平均值。通常,只是简单地把最
近一点的风速观测值作为下一点的风速预测值。该
模型的预测误差较大,且预测结果不稳定。随机时
DOI:10.13334/j.0258-8013.pcsee.2011.31.014
评论0
最新资源