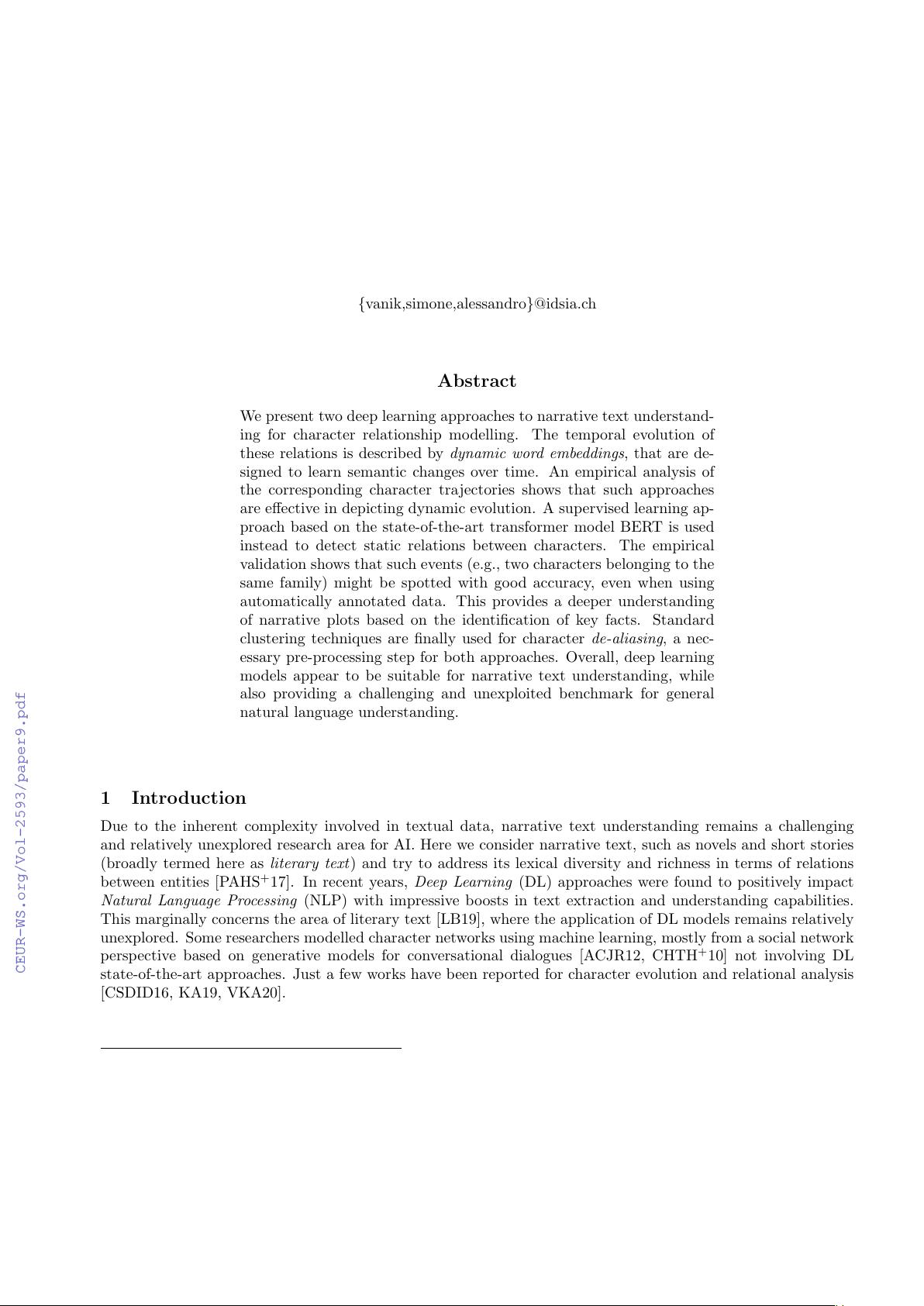
Temporal Embeddings and Transformer Models for
Narrative Text Understanding
Vani K Simone Mellace Alessandro Antonucci
Istituto Dalle Molle di Studi sull’Intell ige nz a Artificiale (IDSIA)
Lugano (Switzerland)
{vanik,simone,alessandro}@idsia.ch
Abstract
We present two deep learning approaches to narrative text understand-
ing for char act er relationship modelling. The temporal evolution of
these relations is described by dynamic word embeddings, that are de-
signed to learn semantic changes over time. An empirical analysis of
the corresponding character trajectories shows that s uch approaches
are e↵ective in depicting dynamic evolution. A super v is ed learning ap-
proach based on the state-of-the-art transformer model BERT is used
instead t o detect static relations between characters. The empi r i cal
validation shows that such events (e. g. , two characters belonging to the
same family) might be spotted with good accuracy, even when using
automatically annotated data. This provi de s a deeper understanding
of narrative plots based on the identification of key facts. Standard
clustering techni q u es are finally used for character de-aliasing,anec-
essary pre-processing step for both approaches. Overall, deep learning
models appear to be suitable for narrative text understanding, while
also providing a chal l en gi ng and unexploited benchmark for general
natural language understanding.
1 Introduction
Due to the inherent complexity involved in textual data, narrative text understanding remains a challenging
and relatively unexplored research area for AI. Here we consider narrative text, such as novels and short stories
(broadly termed here as l iterary text) and try to address its lexical diversity and richness i n terms of relations
between entities [PAHS
+
17]. In recent years, Deep Learning (DL) approaches were found to p osi t ively impact
Natural Language Processing (NLP) wi t h impressive boost s in text extraction and understanding capabilities.
This marginally concerns the area of literary text [LB19], where the application of DL models re mai n s relatively
unexplored. Some researchers modelled character networks using machine le ar ni n g, mostly from a soc i al network
perspective based on ge ne rat i ve models for conversational dialogues [ACJR12, CHTH
+
10] not involving DL
state-of-the-art approaches. Just a few works have been reported for character evolution and relational analysis
[CSDID16, KA19, VKA20].
Here we evaluate the application of DL to literary text understanding. The goal is to describe character
relationships within a novel and their evolution. Moreover, we also want to emphasize t h e potential of literary
Copyright
c
by the paper’s authors. Use permitted under Creative Commons License Attributio n 4.0 International (CC BY 4.0).
In: R. Campos, A. Jorge, A. Jatowt, S. Bhatia (eds.): Proceedings of the Text2Story’20 Workshop, Lisbon, Portugal, 14-April-2020,
published at http://ceur-ws.org
评论0
最新资源