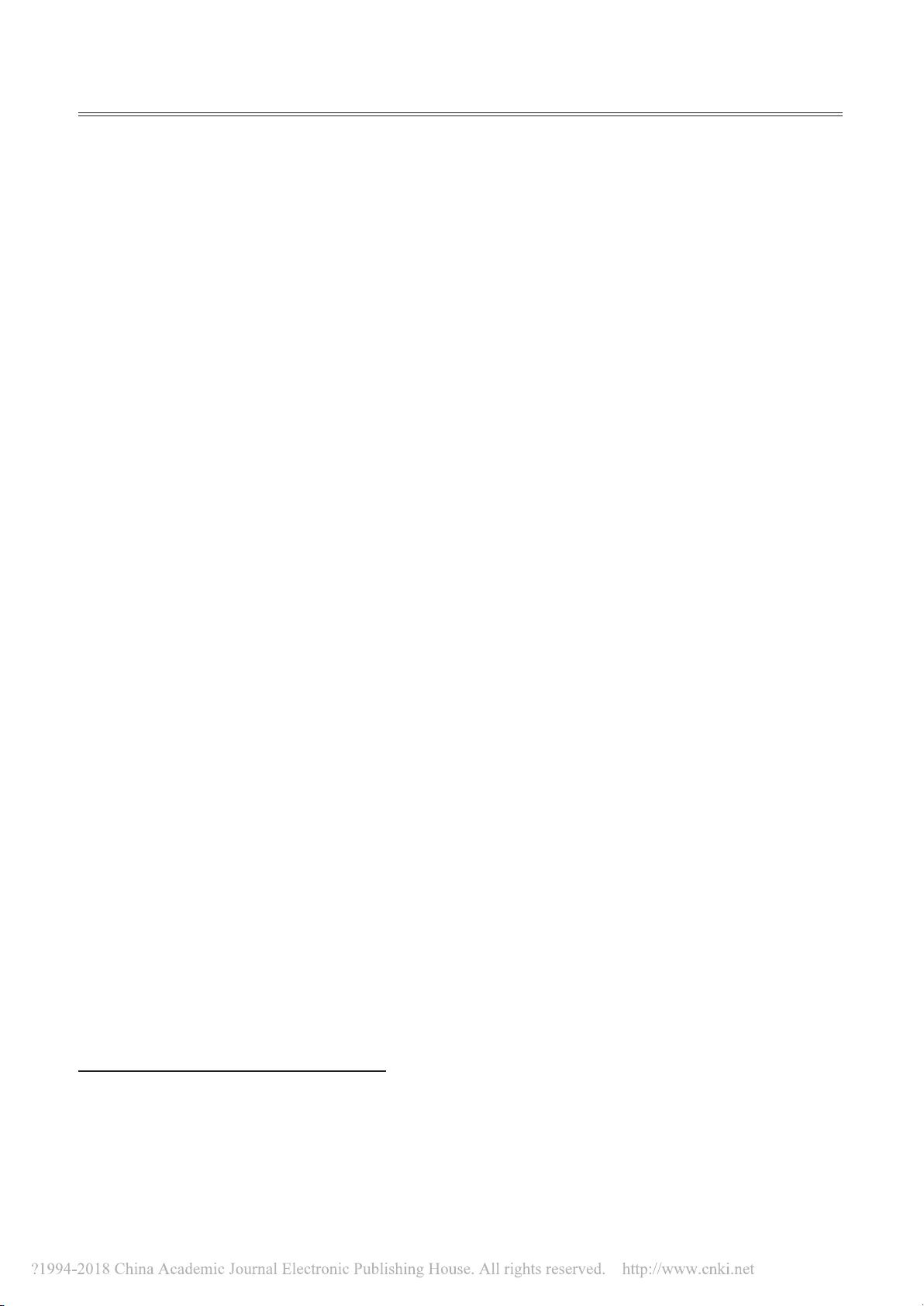
2018,54(12)
1 引言
癌症是威胁人类健康和生命的主要杀手之一,其中
乳 腺 癌 是 女 性 中 最 常 见 的 癌 症 ,据 世 界 卫 生 组 织
(WHO)下属的国际癌症研究中心(IARC)2012 年公布
的数据显示
[1]
,乳腺癌是造成女性死亡的第二大病症,且
其发病率呈逐年上升和年轻化的趋势。临床上,相对于
X-ray、钼靶、核磁共振等图像,病理图像是医生最后确
诊乳腺癌的重要标准,而对病理图像进行准确地分类是
医生制订最佳治疗方案的重要依据。目前基于人工的
病理图像分类,不仅耗时、费力,而且诊断结果容易受到
诸 多 主 观 人 为 因 素 的 影 响 ,借 助 于 计 算 机 辅 助 诊 断
(Computer-Aided Diagnosis ,CAD),自动将病理图像进
行良、恶性分类,不仅可以提高诊断效率,还能为医生提
供更加客观、准确的诊断结果,因而具有重要的临床应
用价值。
乳腺癌病理图像自动分类是一项非常有挑战性的
工作。一是由于病理图像自身的特点:图像之间细微的
差异性、细胞重叠现象、颜色分布不均匀等,给图像分类
工作带来了很大的困难。二是由于缺乏大型公开的、已
标记的数据集,给算法研究带来一定的困难。尽管如
基于深度学习的乳腺癌病理图像自动分类
何雪英,韩忠义,魏本征
HE Xueyi ng, HA N Zhongyi, WEI Benzheng
山东中医药大学 理工学院,济南 250355
College of Sci ence and Technology, Shando ng Unive rsity of Traditional Ch inese Medicine, Jinan 250355, China
HE Xu eying, HAN Zhongyi, WEI Benzheng. Breast cancer histopathological image auto- classification using deep
learning. Computer Engineering and Ap plications, 2 018, 54(12):121-125.
Ab stract:The auto-classific atio n has a significant clinical value for breast canc er histopathol ogical image. The conven-
tional image classification method based on hand-crafted features is professional knowledge demanding and time-consuming.
Beside s, t he conventional met hod can not extract discrimi nati ve features in most cases. To this end, this paper proposes an
improved deep conv olutional neur al network model to realize automatic histopathol ogical ima ge classification with higher
accuracy. Data augmentation and transfer l earning ar e also adopted to avoid the overfitting by the limitation of training
samples. The experimental results show that the recognition r ate rises up to 91% and the proposed method has good
robustness a nd generalization.
Key words:breast cancer histopathological image classification; deep leaning; convolutional neural network; transfer learning;
data augmentation
摘 要:乳腺癌病理图像的自动分类具有重要的临床应用价值。基于人工提取特征的分类算法,存在需要专业领域
知识、耗时费力、提取高质量特征困难等问题。为此,采用一种改进的深度卷积神经网络模型,实现了乳腺癌病理图
像的自动分类 ;同时,利用数据增强和迁移学习方法,有效避免了深度学习模型受样本量限制时易出现的过拟合问
题。实验结果表明,该方法的识别率可达到 91%,且具有较好的鲁棒性和泛化性。
关键词:乳腺癌病理图像分类 ;深度学习;卷积神经网络;迁移学习 ;数据增强
文献标志码:A 中图分类号:T P391.4 doi:10.3778/j.issn.1002-8331.1701-0392
基金项目:山东省自然科学基金(No.ZR2015FM010);山东省高等学校科技计划项目(No.J15LN20);山东省医药卫生科技发展计
划项目(No.201 6WS0577);山东省中医药科技发展计划项目(No.2015-026)。
作者简介:何雪英(1979—),女,讲师,研究领域为机器学习、医学图像处理,E-mail:hxy0104@163.com;韩忠义(1994—),男,硕士
研究生,研究领域为医学图像处理、机器学习;魏本征(1976—),男,博士,副教授,硕士生导师,研究领域为医学图像处
理、机器学习、医学信息工程。
收稿日期:2017-02-06 修回日期:2017-04-01 文章编号:1002-8331(2018)12-0121-05
CN KI 网络出版:2017-06-27, http://kns.cnki.net/kcms/deta il/11.2127.TP.20170627.1712.008.html
Computer Engineering and Applications 计算机工程与应用
121
评论0