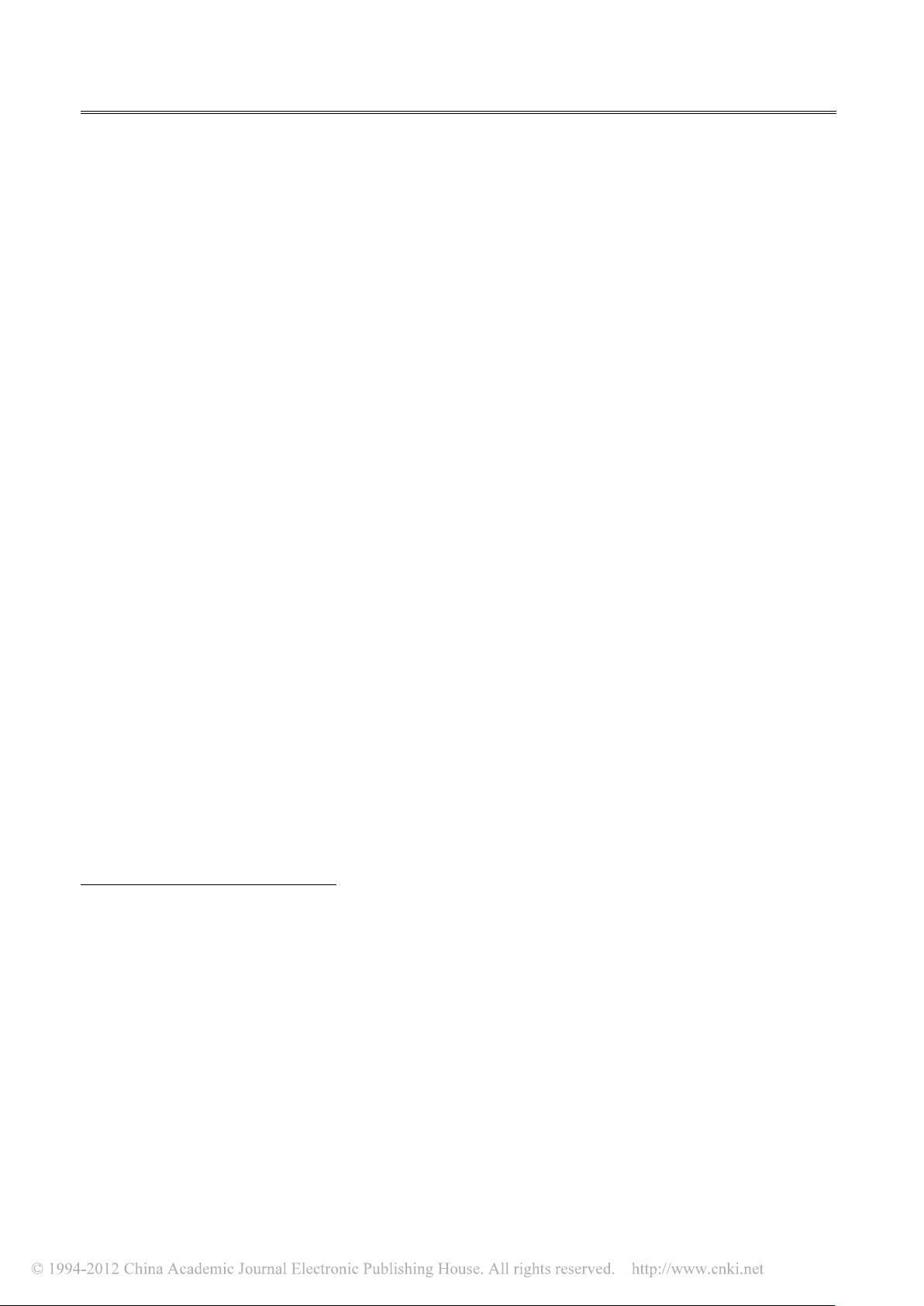
第 35 卷 第 6 期 自 动 化 学 报 Vol. 35, No. 6
2009 年 6 月 ACTA AUTOMATICA SINICA June, 2009
复杂高炉炼铁过程的数据驱动建模及预测算法
郜传厚
1
渐 令
2
陈积明
3
孙优贤
3
摘 要 高炉炼铁过程的控制意味着控制高炉铁水温度及成份在指定的范围. 本文以高炉炉内热状态的重要指示剂 — 高炉
铁水硅含量为研究对象, 针对机理建模难以准确预测、控制高炉铁水硅含量的发展变化, 利用数据驱动建模的思想, 建立了基
于多元时间序列的高炉铁水硅含量数据驱动预测模型. 实例分析表明, 建立的数据驱动预测模型能够很好地预测高炉铁水硅
含量, 连续预测 167 炉高炉铁水硅含量, 命中率高达 83.23 %, 预测均方根误差为 0.07260. 这些指标均优于基于单一硅时间序
列所建立的数据驱动模型, 对实际生产具有很好的指导作用.
关键词 高炉炼铁过程, 数据驱动, 预测模型, 铁水硅含量, 时间序列
中图分类号 TP27
Data-driven Modeling and Predictive Algorithm for
Complex Blast Furnace Ironmaking Process
GAO Chuan-Hou
1
JIAN Ling
2
CHEN Ji-Ming
3
SUN You-Xian
3
Abstract Control of blast furnace ironmaking process means to control the temperature and compositions of blast
furnace hot metal within specific bounds. For the silicon content in blast furnace hot metal as a targeted indicator of
in-furnace thermal state, a data-driven based predictive model is constructed in this paper utilizing the information of
multivariate time series measured from the blast finance system. Through an example, it is indicated that the constructed
data-driven based predictive model has a good performance in predicting the silicon content in hot metal, with 83.23 %
p ercentage of target hitting and 0.07260 of the root mean square error of prediction when the size of predicted sample
set is 167. These criteria are better than those achieved by data-driven predictive model based on univariate time series,
implying that the constructed data-driven predictive model based on multivariate time series can act as an important tool
to serve the production process.
Key words Blast furnace ironmaking process, data-driven, predictive model, silicon content in hot metal, time series
高炉炼铁过程是一个高度复杂的非线性过程,
其实质是将铁从铁矿石等含铁化合物中还原出来.
冶炼期间, 炉内将发生复杂的气 – 固、固 – 固、固 –
液相反应, 并伴随有高温、高压、多相共存、化学反
应与传递现象同时发生等特点. 高炉炼铁过程的复
杂性造成了对其进行有效控制十分困难, 时至今日,
炼铁过程仍要求高炉工长凭经验进行调节, 不能实
现自动控制. 目前, 高炉炼铁过程的建模与控制研究
已引起控制理论界和工业界的高度重视, 成为当今
收稿日期 2008-12-08 收修改稿日期 2009-03-04
Received December 8, 2008; in revised form March 4, 2009
国家自然科学基金 (10826100), 高等学校博士点科研基金 (2007033
5161), 浙江省自然科学基金 (Y107110), 浙江大学工业控制技术国家重
点实验室开放课题 (ICT0904) 资助
Supported by National Natural Science Foundation of China
(10826100), Research Fund for Doctoral Program of Higher Ed-
ucation of China (20070335161), Natural Science Foundation of
Zhejiang Provincial (Y107110), and Open Project of State Key
Laboratory of Industrial Control Technology, Zhejiang Univer-
sity (ICT0904)
1. 浙江大学数学系 杭州 310027 2. 中国石油大学 (华东) 数学与计
算科学学院 东营 257061 3. 浙江大学控制系 杭州 310027
1. Department of Mathematics, Zhejiang University, Hangzhou
310027 2. School of Mathematica and Computational Science,
China University of Petroleum, Dongying 257061 3. Depart-
ment of Control, Zhejiang University, Hangzhou 310027
DOI: 10.3724/SP.J.1004.2009.00725
冶金科技发展的前沿课题
[1]
.
长期以来, 国内外研究人员根据高炉炼铁内部
所发生的化学反应和传递现象建立了多种机理数学
模型
[2−4]
. 这些模型在理论上对于揭示高炉内部现
象和反映高炉炼铁机理起了一定的积极作用, 但亦
存在着准确性低及计算耗时多等缺点. 鉴于此, 数据
驱动建模技术正被广泛地研究, 以试图实现对高炉
炼铁过程的实时模拟和控制. 数据驱动建模技术是
以描述样本数据的特征作为建模的主要准则, 在 “数
据为自身说话” 的信念之下分析系统变量间的相互
关系, 其实质是一种 “黑箱” 建模技术. 高炉在炼铁
过程中, 具有丰富的在线和离线测量数据, 如原料参
数, 包括铁矿石成份、焦炭负荷、喷煤速率、焦炭比
等; 鼓风参数, 包括风量、风温、风压、富氧程度、鼓
风湿度等; 铁水成份参数, 包括铁水硅含量、铁水硫
含量等; 炉渣成份、炉渣碱度以及透气性指数等, 这
为实现高炉炼铁过程数据驱动建模提供了可能. 目
前, 利用数据驱动的思想所建立的高炉预测及控制
模型主要有自回归模型
[5]
、神经网络模型
[6−7]
、非线
性时间序列分析模型
[8]
、模糊模型
[9]
、贝叶斯网络模
型
[10]
、偏最小二乘模型
[11]
、支持向量机模型
[12]
等.
评论0
最新资源