"""
Copyright (C) 2011-2012 Brandon L. Reiss
Permission is hereby granted, free of charge, to any person obtaining a copy of
this software and associated documentation files (the "Software"), to deal in
the Software without restriction, including without limitation the rights to
use, copy, modify, merge, publish, distribute, sublicense, and/or sell copies
of the Software, and to permit persons to whom the Software is furnished to do
so, subject to the following conditions:
The above copyright notice and this permission notice shall be included in all
copies or substantial portions of the Software.
THE SOFTWARE IS PROVIDED "AS IS", WITHOUT WARRANTY OF ANY KIND, EXPRESS OR
IMPLIED, INCLUDING BUT NOT LIMITED TO THE WARRANTIES OF MERCHANTABILITY,
FITNESS FOR A PARTICULAR PURPOSE AND NONINFRINGEMENT. IN NO EVENT SHALL THE
AUTHORS OR COPYRIGHT HOLDERS BE LIABLE FOR ANY CLAIM, DAMAGES OR OTHER
LIABILITY, WHETHER IN AN ACTION OF CONTRACT, TORT OR OTHERWISE, ARISING FROM,
OUT OF OR IN CONNECTION WITH THE SOFTWARE OR THE USE OR OTHER DEALINGS IN THE
SOFTWARE.
Processes a sequence of nighttime traffic images and extracts the bright
objects.
"""
import sys
import pylab as plab
import scikits.image.color
import scipy
import scipy.ndimage as ndimg
import numpy as np
import pyopencl as cl
import PIL
import pymorph
import os
import fnmatch
import datetime
import fractions
# GMM parameters for script-run.
GMM_K = 3
GMM_NUM_FRAMES = 25
GMM_W_INIT = 0.1
GMM_VAR_INIT = 20
GMM_MAHA_THRESH = 3
def rgb2ycbcr(image):
"""
Convert a unit8 RGB image to full-range Y'CbCr image. This is not intended
for use directly on broadcast video systems.
"""
assert(image.dtype == 'uint8')
# Matrix for RGB to full-range YCbCr conversion.
# http://www.equasys.de/colorconversion.html
A = np.array([[ 0.299, 0.587, 0.114],
[-0.169, -0.331, 0.500],
[ 0.500, -0.419, -0.081]], dtype='f4')
# Convert.
yuv = np.dot(image.astype('f4'), A.T) + \
np.array([0., 128.5, 128.5], dtype='f4')
return np.clip(yuv, 0, 255).astype('uint8')
# GMM:
# * Use u_t in expression for s_t. Update mean first.
# * w_K^N+1 = ((1 - a) * w_k^N) + (a * p(k|x_N+1, theta_t-1))
# where a = (1 / (N + 1))
# * u_K^N+1 = ((1 - p_k) * u_k^N) + (p_k * x_N+1)
# where p_k = (a * p(k|x_t, theta^old)) / w_k^new
# * s_k^N+1 = ((1 - p_k) * s_k^N) + (p_k * (x_N+1 - u_k^N+1) *
# (x_N+1 - u_k^N+1)^T)
# where p_k = (a * p(k|x_t, theta^old)) / w_k^new
class GaussianMixtureModelUV(object):
"""
A Gaussian Mixture Model (GMM) is an online statistical background model
invented by Stauffer and Grimson. It attempts to learn a k-modal
mixure of gaussian distributions corresponding to k surfaces observed
at pixel [i,j] of the image. For pixels exibiting unimodal through
k-modal behavior, the GMM will adapt the appropriate number of modes
evidenced by the allocation of k weights among its k mixture components.
"""
# Number of parameters required to model one gaussian component.
GAUSS_COMPONENT_SIZE = 5
# Mean used during model initialization.
MU_INIT = 32.
# Minimum variance.
VAR_MIN = 2.
# Total density included in the model.
DENSITY_THRESH = 0.8
def __init__(self, k, size, num_frames, w_init, var_init, maha_thresh):
# Collect CPU style params.
self._k = k
self._size = tuple(size[:2])
self._num_frames = num_frames
self._alpha = 1. / (num_frames + 1)
self._w_init = w_init
self._var_init = var_init
self._maha_thresh = maha_thresh
# Collect GPU style params.
self._params = np.zeros((1,), dtype=[('size','2i4'),
('k','i4'),
('maha_thresh','f4'),
('alpha','f4'),
('w_init','f4'),
('var_init','f4'),
('var_min','f4'),
('density_thresh','f4')])
self._params['size'] = np.array(self._size)
self._params['k'] = self._k
self._params['maha_thresh'] = self._maha_thresh
self._params['alpha'] = self._alpha
self._params['w_init'] = self._w_init
self._params['var_init'] = self._var_init
self._params['var_min'] = self.VAR_MIN
self._params['density_thresh'] = self.DENSITY_THRESH
# Create the model.
model_shape = tuple(size[:2]) + (k, self.GAUSS_COMPONENT_SIZE,)
self._model = np.zeros(model_shape, dtype='f4')
# Initialize model.
self._model[:,:,:,:] = np.array([1. / k,
self.MU_INIT, self.MU_INIT,
var_init, var_init])
# Setup OpenCL.
self._setup_opencl()
self._setup_opencl_buffers()
def _setup_opencl(self):
""" Setup OpenCL elements for GMM. """
# Setup OpenCL device and queue.
os.environ['PYOPENCL_CTX'] = '1'
self._context = cl.create_some_context()
self._queue = cl.CommandQueue(self._context)
# Load OpenCL program.
program_text = open('./gmm.cl').read();
self._program = cl.Program(self._context, program_text).build()
# Determine work sizes.
local_mem_size = cl.device_info.LOCAL_MEM_SIZE
sizeof_gaussians = self._model[0][0].nbytes
max_local_work = int(local_mem_size / sizeof_gaussians)
local_work_dim = int(np.sqrt(max_local_work))
self._local_work_size = (local_work_dim, local_work_dim)
self._local_gauss_buf_size = local_work_dim * local_work_dim * \
sizeof_gaussians
assert(self._local_gauss_buf_size <= local_mem_size)
global_work_blocks_x = int((float(self._size[0]) / local_work_dim) +
0.5)
global_work_blocks_y = int((float(self._size[1]) / local_work_dim) +
0.5)
self._global_work_size = (local_work_dim * global_work_blocks_x,
local_work_dim * global_work_blocks_y)
max_work_group_dim = int(np.sqrt(cl.device_info.MAX_WORK_GROUP_SIZE))
rgb2ycbcr_local_work_dim = fractions.gcd(
fractions.gcd(self._size[0], max_work_group_dim),
fractions.gcd(self._size[1], max_work_group_dim))
self._rgb2ycbcr_local_work_size = (rgb2ycbcr_local_work_dim,
rgb2ycbcr_local_work_dim)
def _setup_opencl_buffers(self):
""" Setup OpenCL buffers for GMM. """
# Setup buffers for kernels.
self._params_buf = cl.Buffer(self._context,
cl.mem_flags.READ_ONLY |
cl.mem_flags.COPY_HOST_PTR,
hostbuf=self._params)
self._gauss_buf = cl.LocalMemory(self._local_gauss_buf_size)
self._model_buf = cl.Buffer(self._context,
cl.mem_flags.READ_WRITE |
cl.mem_flags.COPY_HOST_PTR,
hostbuf=self._model)
image_size = self._size[0] * self._size[1] * 3
self._img_read_buf = cl.Buffer(self._context,
cl.mem_flags.READ_ONLY,
size=image_size)
self._img_write_buf = cl.Buffer(self._context,
cl.mem_flags.WRITE_ONLY,
si
没有合适的资源?快使用搜索试试~ 我知道了~
资源推荐
资源详情
资源评论
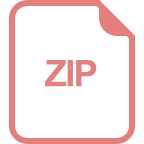
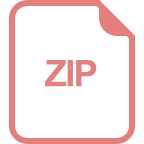
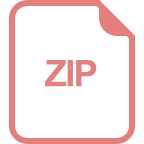
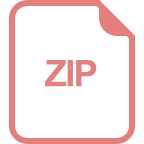
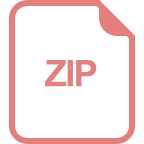
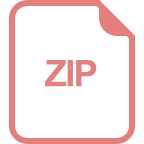
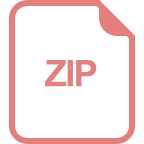
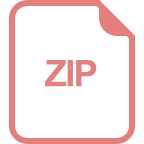
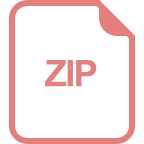
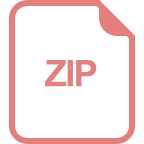
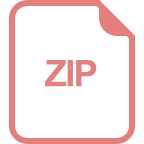
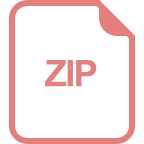
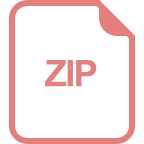
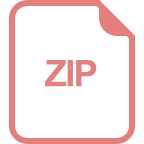
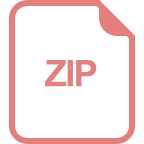
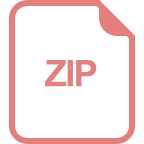
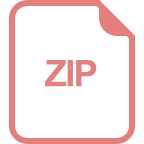
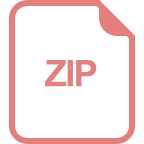
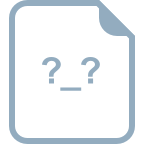
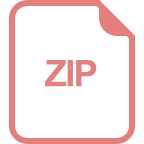
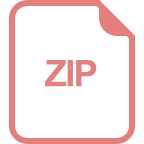
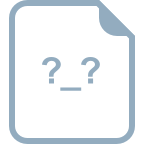
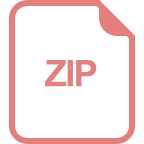
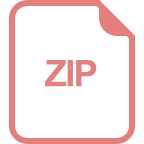
收起资源包目录


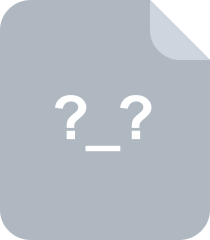
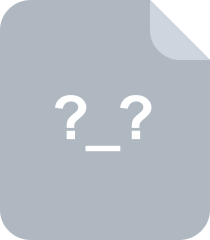
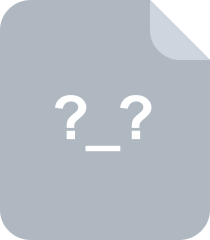
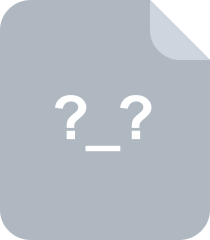
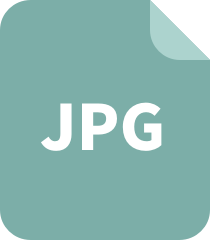
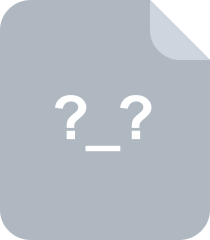
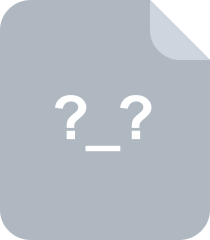
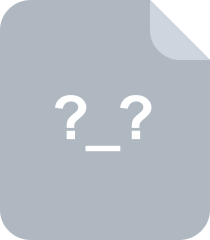
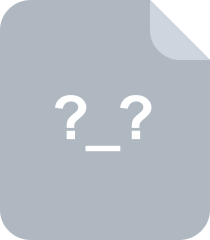
共 9 条
- 1
资源评论
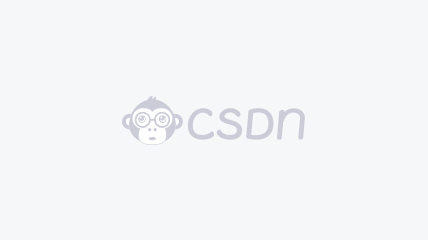

小码蚁.
- 粉丝: 2676
- 资源: 4542
上传资源 快速赚钱
我的内容管理 展开
我的资源 快来上传第一个资源
我的收益
登录查看自己的收益我的积分 登录查看自己的积分
我的C币 登录后查看C币余额
我的收藏
我的下载
下载帮助

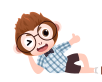
安全验证
文档复制为VIP权益,开通VIP直接复制
