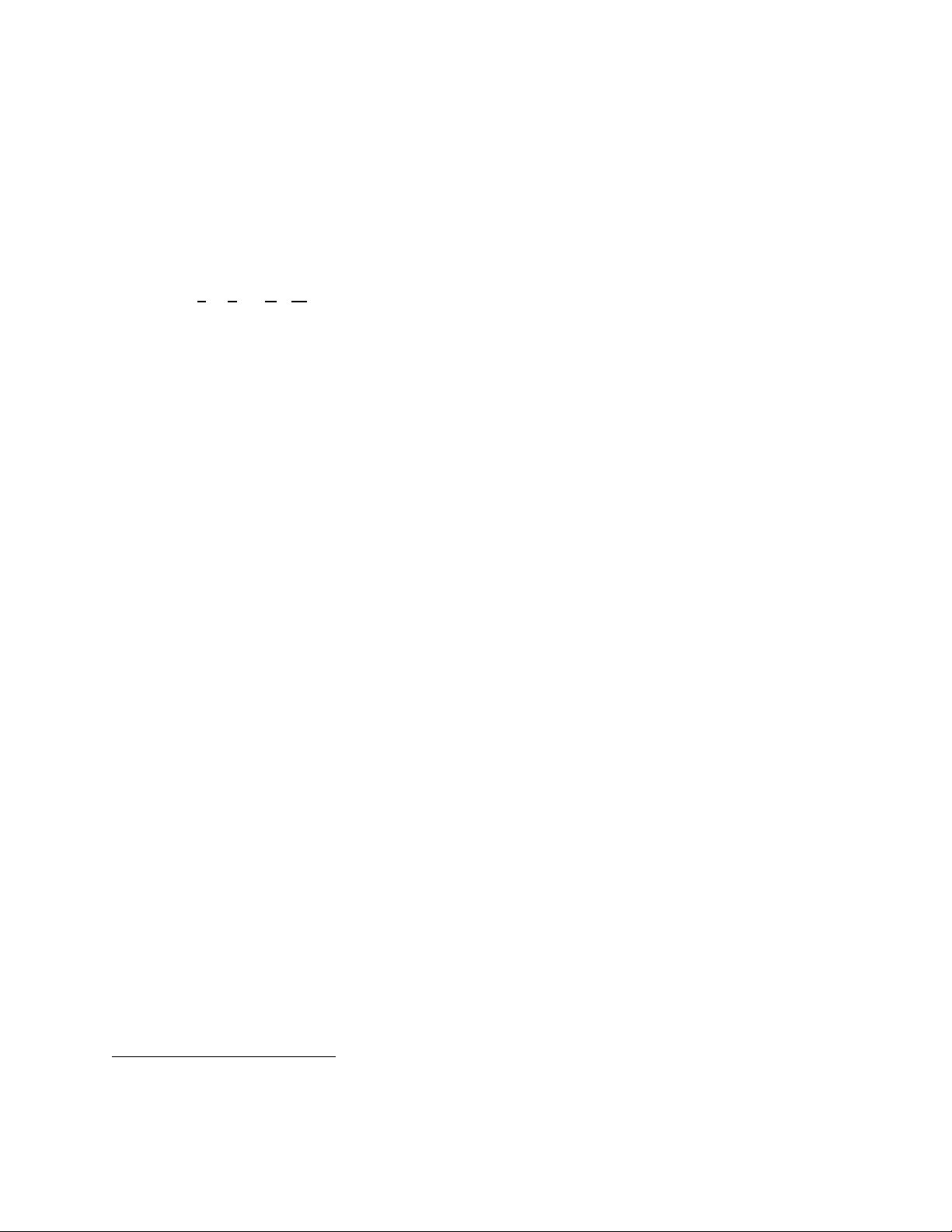
Several estimating methods developed in recent years
have shown that generalized predictions can be made
with usable, but variable, accuracy (Rawls et al., 1982;
Saxton et al., 1986; Williams et al., 1992; Stolte et al.,
1994; Kern, 1995). Nearly all of these methods involve
multiple soil descriptors, some of which are often not
available for practical applications. Most were derived
by statistical correlations, although more recent analyses
have explored neural network analysis (Schaap et al.,
1998) or field descriptions and pedotransfer functions
(Grossman et al., 2001; Rawls and Pachepsky, 2002).
Gijsman et al. (2002) reported an extensive review of
eight modern estimating methods applicable to hydro-
logic and agronomic analyses. They observed significant
discrepancy among the methods due to the regional data
basis or methods of analyses thus creating doubt on the
value of lab-measured water retention data for crop
models. They concluded that…“ an analysis with a set of
field-measured data showed that the method of Saxton
et al. (1986) performed the best….” Thus an enhance-
ment of the Saxton et al. (1986) method is an appropriate
extension to improve the field applications of soil water
characteristic estimates with improved data basis and
supplemented by recently derived relationships of con-
ductivity and including appropriate local adjustments
for OM, density, gravel, and salinity.
METHODOLOGY
An extensive laboratory data set of soil water char-
acteristics was obtained from the USDA/NRCS Na-
tional Soil Characterization database (Soil Survey Staff,
2004) consisting of approximately 2000 A-horizon and
2000 B-C horizon samples (B-C a subset of 6700). The
data for each sample included soil water content at 33-
and 1500-kPa tensions; bulk densities; sand (S), silt and
clay (C) particle sizes; and OM. These data were devel-
oped with standard laboratory procedures (USDA-SCS,
1982; Klute, 1986) with reviews and approval for consis-
tency and accuracy.
The B-C horizon data had much less average OM
content than that of the A horizon, 0.6 vs. 2.8% (w)
1
,
respectively. Preliminary correlations showed that com-
bining B-C horizon samples with those of the A-horizon
significantly masked the effect of OM. Because texture
and OM are primary variables affecting soil water
content, only the A-horizon data were used to develop
regression equations.
Samples with “extreme” values were omitted from the
data. Excluded were those with bulk density , 1.0 and
. 1.8 g cm
23
,OM. 8 % (w) and clay . 60% (w). This
reduced the A-horizon data set from 2149 to 1722
samples. Samples outside the density range may have
been the result of tillage or compaction causing them to
be unlike natural soils. The high OM samples were con-
sidered from an “organic” soil whose water character-
istics would not be representative of typical mineral
soils. Soils of very high clay content often have pore
structure and mineralogical effects different than those
containing higher portions of S or silt fractions.
The soil water retention data were correlated with
variables of S, C, and OM and their interactions (Hahn,
1982, p. 218). Density was not included as a correla-
tion variable because it was highly variable within the
A-horizon data set and is not commonly available for
applications. Regression equations were developed for
moisture held at tensions of 1500, 33, 0 to 33 kPa, and
air-entry tensions. Air-entry values were estimated from
the sample data by the exponential form of the Campbell
equation (Rawls et al., 1992, Table 5.1.1). Saturation
moisture (u
s
) values were estimated from the reported
sample bulk densities assuming particle density of
2.65 g cm
23
.
Standard regression methods minimize the statistical
error about a model equation. However, the best equa-
tion form is often unknown and may not adequately
represent the data, thus does not provide a satisfactory
predictive equation. Multi-variable linear analyses are
particularly suspect in this regard because one or more
of the variables may not be linearly correlated with
the dependent variables. This “lack-of-fit” was partially
compensated for by applying a second correlation to the
prediction deviations by the first correlation resulting in
two combined dependent equations, the second being
linear or nonlinear.
Finally, the new moisture tension equations were com-
bined with the conductivity equations of Rawls et al.
(1998) and additional equations for density, gravel, and
salinity effects. The results of the derived correlation
equations were compared with three independent data
sets representative of a wide range of soils to verify their
capability for field applications. A companion com-
puter model and graphical interface of the equations
provides rapid computations and displays for hydro-
logic applications.
PREDICTION EQUATIONS
New predictive equations to estimate soil water con-
tent at selected tensions of 1500, 33, 0 to 33, and c
e
kPa
are summarized in Table 1 (Eq. [1]–[4])
2
. Variable de-
fini tions are shown i n Table 2. The coefficient of
determination (R
2
) and standard error of estimate (S
e
)
define the data representation and expected predic-
tive accuracy.
Moisture at the selected tensions was correlated with
S, C, and OM plus interactions while air-entry tension
(bubbling pressure), c
e
, was correlated with S, C, and
u
S-33
plus interactions. Supplemental analyses of the
initial predictive errors provided “lack of fit” second-
ary adjustment equations for each equation as defined
in methodology.
Graphical results of the correlations are shown in
Fig. 1 for soil moisture and air entry. The best moisture
correlation was obtained for u
1500
(R
2
5 0.86) with
progressively more variability for u
33
(R
2
5 0.63) and
1
%w indicates decimal percent by weight basis, and %v indicates
decimal percent by volume basis.
2
Equations throughout the text are referenced to those in Table 1.
Reproduced from Soil Science Society of America Journal. Published by Soil Science Society of America. All copyrights reserved.
1570 SOIL SCI. SOC. AM. J., VOL. 70, SEPTEMBER–OCTOBER 2006
- 1
- 2
前往页