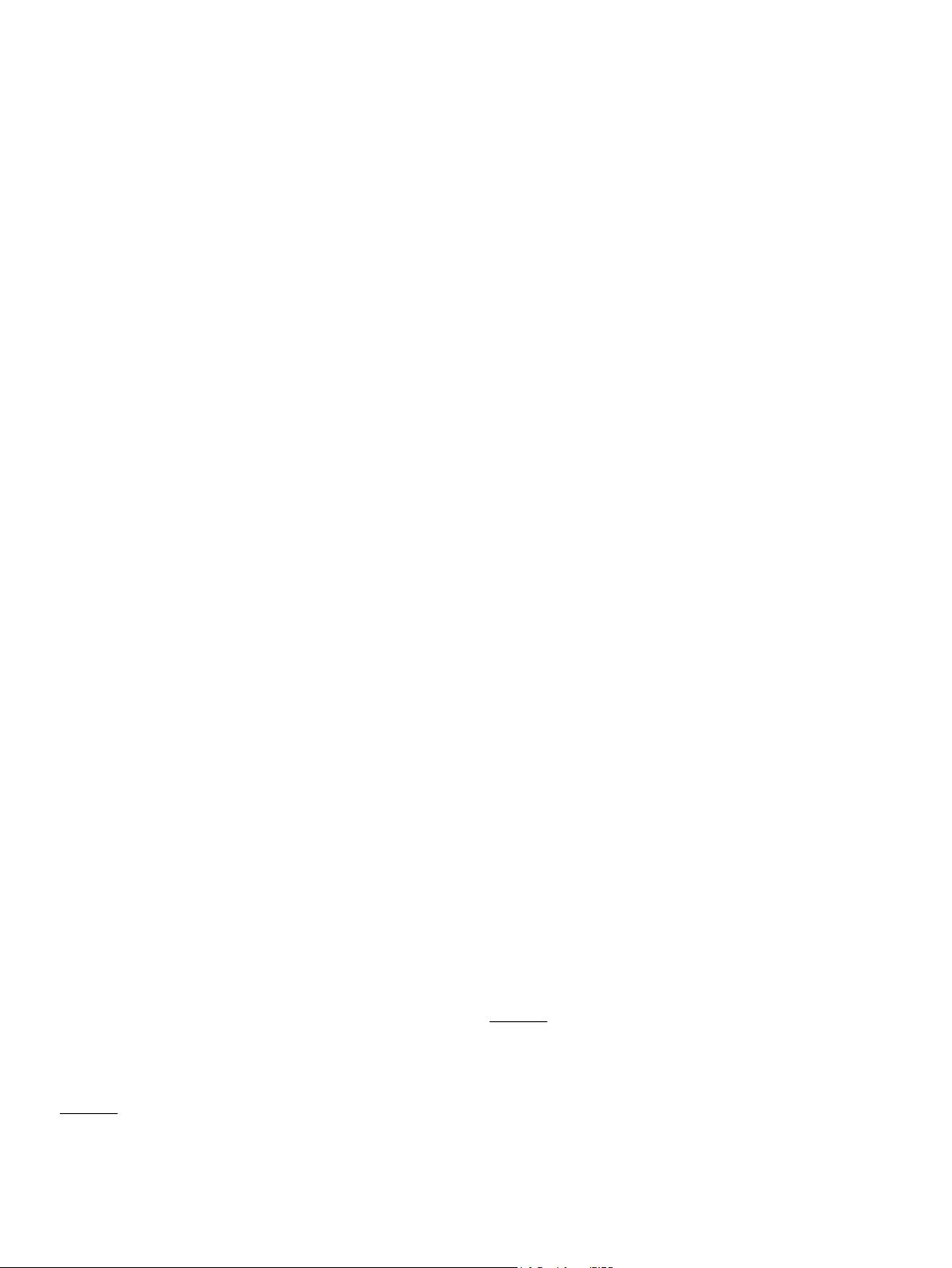
concept embeddings are classified into three types depending on the
concept (code, CUI or patient) they map. (Section 5)
•
We discuss various methods like intrinsic and extrinsic to evaluate
embeddings and present summary of evaluation tasks in various
clinical embeddings. (Section 6)
•
We discuss various challenges like small size of clinical corpus, multi
sense embeddings, domain adaptation, sub-word information, OOV
issue, temporal information and suggest possible solutions from the
surveyed research articles. (Section 7)
•
Finally, we conclude with some of future directions of research in
embeddings like interpretability, knowledge distillation, bias and eva-
luation of embeddings. (Section 8)
2. Medical corpora
In this section, we classify medical corpora into four types as shown
in Fig. 1 and then discuss each type followed by a comparison (see
Table 1).
Embeddings are inferred using any of the embeddings models over a
large unlabeled corpus. Quality of embeddings inferred, depends on
two properties of corpus like size and whether it is general or domain
specific. A large corpus provides better vocabulary coverage while a
domain related corpus provides better semantic representation of
terms. Medical corpora can be classified into four categories.
2.1. Electronic Health Record (EHR)
In recent times, Electronic Health Records have become first option
to store patient details in most of the hospitals [14]. EHRs include both
structured data like diagnostic codes, procedure codes, medication
codes, laboratory results etc. as well as unstructured data like clinical
notes written by health professionals [15]. EHRs containing rich clin-
ical information have become an invaluable source of data for many
clinical informatics applications [16,17]. Some of the research studies
have used publicly available EHR data while others have used private
EHR data. MIMIC Dataset [18,19] is the largest publicly available EHR
dataset and is described below.
2.1.1. MIMIC Dataset
Multiparameter Intelligent Monitoring in Intensive Care (MIMIC)
[18,19] is a publicly available ICU dataset developed by MIT Lab. It
includes demographics, vital signs, laboratory tests, medications, and
more. MIMIC-II [18] contains data collected from Intensive Care Units of
Beth Israel Deaconess Medical Center from 2001 to 2008 while MIMIC III
[19] consists of data collected in between 2001 and 2012 from the same
medical center. The data in MIMIC datasets is deidentified and can be
used for research purpose. But prior to access, agreement to data use and
completion of a training course is mandatory.
2.2. Medical related Social Media Corpus
In recent times, social media evolved as a medium of expression for
internet users. Medical related social media corpus includes tweets
posted by individuals, questions and answers in online discussion
forums related to health issues. In Twitter
1
, users express health related
concerns in short text of 140 characters while health discussion forums
consists of health related questions raised and the corresponding an-
swers. Some of the popular health discussion forums are MedHelp
2
,
DailyStrength
3
, AskAPatient
4
and WebMD
5
. Social media text is highly
informal and conversational in nature with lot of misspelled words,
irregular grammar, non-standard abbreviations and slang words.
Moreover, users describe their experiences in non-standard and de-
scriptive words. Analysis of medical social media text which contains
rich medical information can provide new medical insights and im-
proved health care.
2.3. Online medical knowledge sources
Online medical knowledge sources contain medicine and health
related information which is created and maintained by medical pro-
fessionals. Merriam-Webster Medical Thesaurus
6
, Merriam-Webster
Medical Dictionary
7
and Merck Manual
8
are some of the online medical
knowledge sources. Merriam-Webster Medical Thesaurus consists of
word definition along with example sentence, synonyms, related words
and antonyms while MerriamWebster Medical Dictionary consists of
word definition along with multiple example sentences and synonyms.
Merck Manual is a medical text book having articles related to various
topics including disorders, drugs and tests. From these sources, corpus
can be built and adopted by any embedding model to generate em-
beddings. eMedicine
9
is an online website which consists of almost
6,800 (by December 2018) articles related to various topics in medicine
like Emergency medicine, Internal medicine etc. Each article is au-
thored by a certified specialist in the concerned area which undergoes
four levels of peer view which includes review by Doctor of Pharmacy.
Medical Subject Headings (MeSH)
10
is created and maintained by
United States National Library of Medicine
11
. It is a controlled voca-
bulary used for indexing articles in PubMed and classifying diseases in
clinicaltrials.gov
MedlinePlus
12
maintained by United States National Library of
Medicine offers reliable and updated information on various topics
related to health in an easy to understand language. It is a medical
encyclopedia that has information over 1000 diseases and conditions.
Sciencedaily
13
and Medscape
14
are two other online sources that pro-
vides latest news related to medicine.
2.4. Scientific literature
PubMed
15
maintained by United States National Library of Medi-
cine, is a search engine for citations and abstracts of research articles
published in the areas of life sciences and biomedicine. As of December
2018, PubMed has 14.2 million articles with links to full-text. Apart
from this, it provides access to books with full text available. PubMed
Central (PMC)
16
is a digital repository of research papers published in
the areas of biomedicine and life sciences and it provides free access. As
of December 2018, it has over 5.2 million articles. Table 1 gives a
comparison of various medical corpora.
3. Medical codes
The primary motive behind EHR [20] is to record the patient in-
formation right from admission to discharge in a systematic way. Sev-
eral classification schemes are available for recording relevant clinical
information. For example, ICD (International Statistical Classification of
https://twitter.com
2
https://www.medhelp.org
3
https://www.dailystrength.org/
4
https://www.askapatient.com/
5
https://www.webmd.com/
https://www.merriam-webster.com/thesaurus
7
https://www.merriam-webster.com/medical
8
https://www.msdmanuals.com/
9
https://emedicine.medscape.com/
10
https://www.ncbi.nlm.nih.gov/mesh
11
https://www.nlm.nih.gov/
12
https://medlineplus.gov/
13
https://www.sciencedaily.com/
14
https://www.medscape.com/
15
https://www.ncbi.nlm.nih.gov/pubmed/
16
https://www.ncbi.nlm.nih.gov/pmc/
K.S. Kalyan and S. Sangeetha
Journal of Biomedical Informatics 101 (2020) 103323
2