+------------+
| Kernel ICA |
+------------+
Version 1.1 - May 13th, 2002
------------------------------
Description
-----------
The kernel-ica package is a Matlab program that implements the Kernel
ICA algorithm for independent component analysis (ICA). The Kernel ICA
algorithm is based on the minimization of a contrast function based on
kernel ideas. A contrast function measures the statistical dependence
between components, thus when applied to estimated components and
minimized over possible demixing matrices, components that are as
independent as possible are found. For more information, please read the
following paper:
Francis R. Bach, Michael I. Jordan (2001). Kernel Independent Component
Analysis, Technical Report UCB//CSD-01-1166, University of California,
Berkeley.
The kernel-ica package is Copyright (c) 2002 by Francis Bach. If you
have any questions or comments regarding this package, or if you want to
report any bugs, please send me an e-mail to fbach@cs.berkeley.edu. The
current version 1.0 has been released on March, 19th 2002. It has been
tested on both matlab 5 and matlab 6. Check regularly the following for
newer versions: http://www.cs.berkeley.edu/~fbach
Installation
------------
1. Unzip all the .m files in the same directory
2. (Optional) if you want a faster implementation which uses pieces of C
code: at the matlab prompt, in the directory where the package is
installed, type:
>> mex chol_gauss.c
and
>> mex chol_hermite.c
It should create compiled files whose extensions depends on the platform
you are using:
Windows: chol_gauss.dll and chol_hermite.dll
Solaris: chol_gauss.mexsol and chol_hermite.dll
Linux : chol_gauss.mexglx and chol_hermite.dll
To check if the file was correcly compiled, type
>> which chol_gauss
>> which chol_hermite
and the name of the compiled versions should appear. If you have any
problems with the C file of if you are using a platform i did not
mention, please e-mail me.
How to use the kernel-ica package
---------------------------------
The function that you should use to run the ICA algorithm is
'kernel_ica'. a detailed description of its options are described inside
the file and can be reached by simply typing 'help kernel_ica' at the
matlab prompt. A simple demonstration script is provided :
'demo_kernel_ica'.
NB: all the data should be given in columns, that is, if you have m
components and N samples, the matrix should be m x N.
If you wish to investigate the tools and methods we used for this
algorithms, you will find the following files useful:
-contrast_ica.m : computation of the contrast functions based on
Kernel canonical correlations
-chol_gauss.c/.m : incomplete cholesky decomposition with Gaussian
kernel in one or higher dimensions
-chol_hermite.c/.m : incomplete cholesky decomposition with Hermite
polynomial kernel in (currently) only one dimension
Package file list
-----------------
amari_distance.m : Amari distance between two square matrices
chol_gauss.c : incomplete cholesky (Gaussian kernel) - C source
chol_gauss.m : incomplete cholesky (Gaussian kernel) - M file
chol_hermite.c : incomplete cholesky (Hermite kernel) - C source
chol_hermite.m : incomplete cholesky (Hermite kernel) - M file
chol_poly.c : incomplete cholesky (Polyn. kernel) - C source
chol_poly.m : incomplete cholesky (Polyn. kernel) - M file
contrast_emp_grad.m : derivative of m-way contrast functions
contrast_emp_grad_oneunit.m : derivative of the one-unit contrast functions
contrast_ica.m : m-way contrast functions
contrast_update_oneunit.m : one-unit contrast function
demo_kernel_ica.m : demonstration script
empder_search.m : local search (reaches a local minimum) - full contrast
empder_search_oneunit.m : local search (reaches a local minimum) - one-unit contrast
global_mini.m : global minimization with random restarts - full contrast
global_mini_oneunit.m : global minimization with random restarts - one-unit contrast
global_mini_sequential.m : global minimization with random restarts
one-unit contrast + deflation scheme
kernel_ica.m : performs ICA using the kernel ICA algorithm
rand_orth.m : generates random matrix with orthogonal columns
update_contrast.m : used for efficient computation of empirical gradient

sundaoda
- 粉丝: 1
- 资源: 10
最新资源
- ncnn 手机端极致优化的高性能神经网络前向计算框架
- 鞋、帽、头发、头检测54-YOLO(v5至v9)、COCO、CreateML、Paligemma、TFRecord、VOC数据集合集.rar
- 蓝屏信息速查bluescreenview-1.5.5.0(电脑蓝屏分析工具)
- VID_20241204_173621760.mp4
- IATF16949-2016 汽车生产件及相关服务件组织的质量管理体系要求
- C++实现手动开平方根
- rufus-3.19(PE制作工具)
- 基于keras集成常见图像分类模型含VGG16-19、InceptionV3、Xception、MobileNet、AlexNet、LeNet、ZF-Net、ResNet、DenseNet.zip
- Vue vs Angular:2024 年选择哪个框架
- 宽带账户密码生成器(获取上网帐号密码并自动保存到D盘)
资源上传下载、课程学习等过程中有任何疑问或建议,欢迎提出宝贵意见哦~我们会及时处理!
点击此处反馈


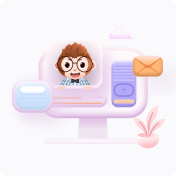