没有合适的资源?快使用搜索试试~ 我知道了~
温馨提示
The generation of good label placement arrangements is a frequent problem when producing maps. The objective of a good label placement is to display the geographic position of the features with their matching label in a clear and harmonious fashion, following accepted cartographic conventions. In this work, we propose the fast algorithm for label placement (FALP) for generation of real time screen maps. FALP is a cost-effective choice, with good quality performance and excellent runtime performance.
资源推荐
资源详情
资源评论
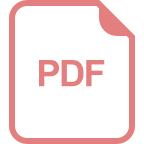
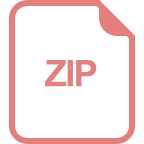
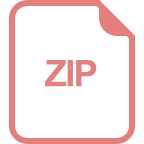
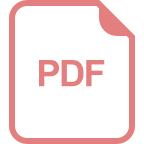
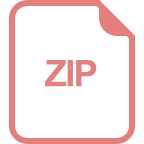
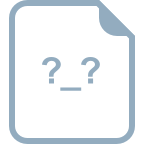
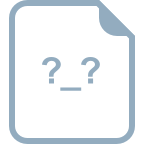
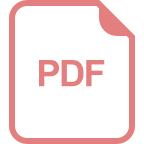
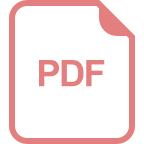
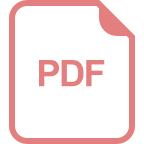
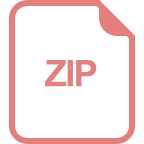
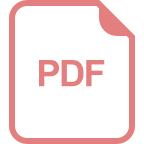
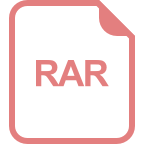
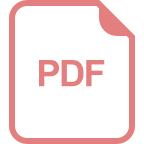
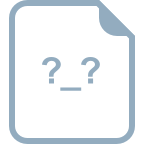
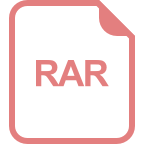
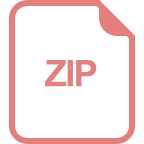
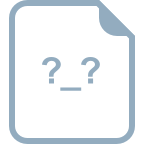
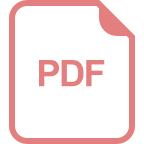
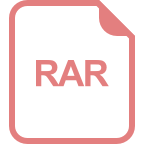
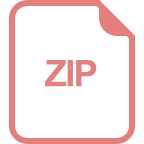
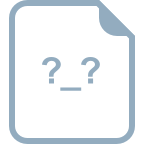
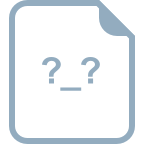
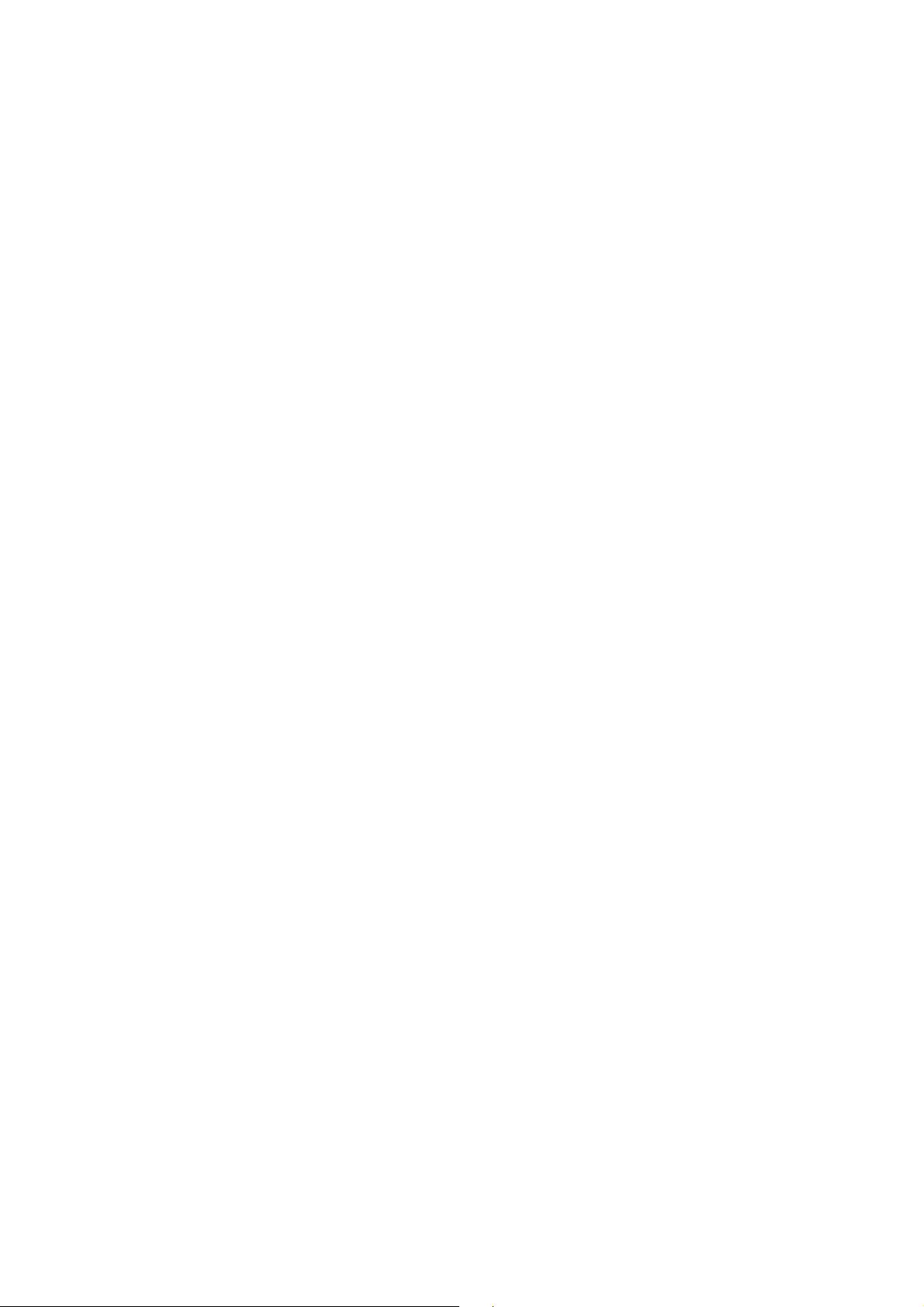
Fast Point-Feature Label Placement Algorithm for Real Time
Screen Maps
Missae Yamamoto, Gilberto Camara, Luiz Antonio Nogueira Lorena
National Institute of Space Research - INPE, São José dos Campos, SP, Brazil
São José dos Campos – SP – Brazil
{missae, gilberto}@dpi.inpe.br, lorena@lac.inpe.br
Abstract. The generation of good label placement arrangements is a frequent
problem when producing maps. The objective of a good label placement is to
display the geographic position of the features with their matching label in a
clear and harmonious fashion, following accepted cartographic conventions.
In this work, we propose the fast algorithm for label placement (FALP) for
generation of real time screen maps. FALP is a cost-effective choice, with
good quality performance and excellent runtime performance.
1. Introduction
Point label placement refers to insertion of text in maps and is a challenging problem in
geoinformatics and automated cartography (Wolff and Strijk 1996). Text positioning
requires avoiding overlaps and adherence to cartographic conventions and preferences.
There should be unambiguous association between each text and its matching feature.
Overall, good labeling needs harmony and quality in the resulting maps. The motivation
for research in automated label placement includes:
• Label placement is a fundamental part of producing good maps and essential
item to communicate spatial information;
• Placing text manually in maps is a laborious procedure;
• A good label placing algorithm brings a substantial increase in map productivity;
Label placement would be much simpler if labels could be pre-computed for
each layer at once and their position stored beforehand. Unfortunately, this is not
possible. Map production frequently requires information from more than one layer. It is
the last step in map production. After the user chooses the output layers, the scale and
the limits of the map, object labels need to be placed effectively.
Screen maps are created as answers to spatial queries on geographical
information systems (GIS), either in desktop or Web applications. To allow for a
smooth uninterrupted response, the maps must be produced “on the stroke of a key”.
Otherwise, the user gets impatient. Screen map production needs to balance the quality
of label placement with the processing time. Label placement techniques that are of
extremely good quality but need much processing time are not useful for screen maps.
The most common label placement problem is the point feature label placement
(PFLP) problem. Given a set of places associated to point locations, the PFLP consists
in placing the names of these places in the map with quality and efficiency. This work
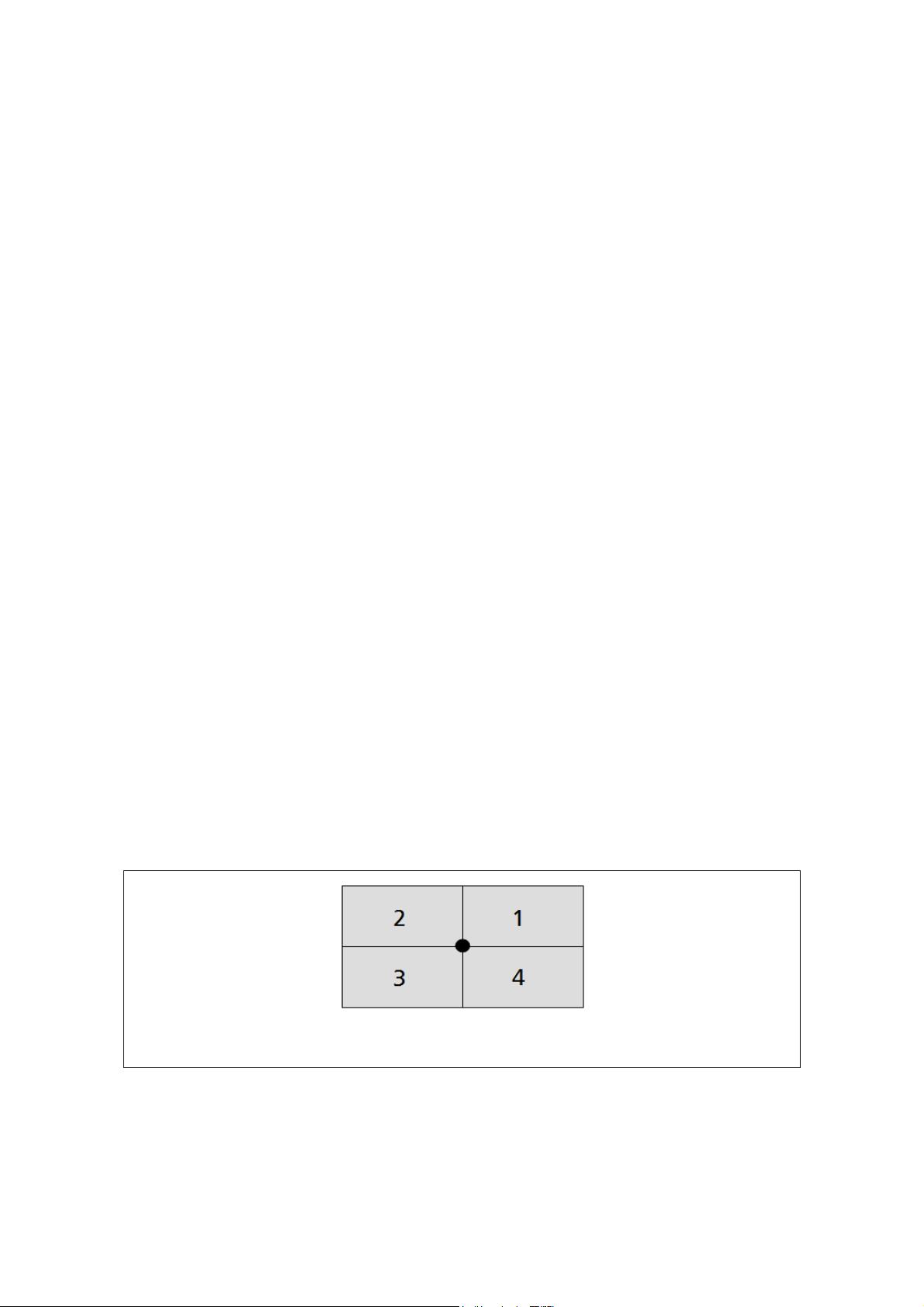
describes the Fast Algorithm for Label-Placement (FALP) to solve the PFLP. Our
results show the FALP has good performance in label placement processing time and
presents a good label placement quality for a screen map. Given its relative simplicity of
implementation and efficient performance, we believe that FALP method is a good
solution to the PFLP problem for screen maps.
In Section 2 of the paper, we review the literature on label placement. In Section
3, we present the conflict graph, a structure that represents all conflicts between
candidate labels. Section 4 we introduce the FALP algorithm. Section 5 shows
computational results using instances formed by standard sets of randomly generated
points suggested in the literature.
2. Review of the point label placement problem
There are three different label-placement problems: labeling of point features (cities,
schools, hospital, mountain peaks …), line features (rivers, roads, …), and area features
(countries, states, oceans, …). In this article we focus on placing labels for point
features using combinatorial optimization. We will use three key notions, defined
below:
• Label positions for each point feature. Given a point, a label can be placed on
one of four positions relative to it (see Figure 1). Therefore, a label position is an
ordered pair (point location, relative position). In Figure 1, each box marks a
label position.
• Cartographic preference. Usually, we prefer to place labels at the upper right
corner of the point, and this preference decreases counterclockwise from this
position. The value inside each box in Figure 1 matches the order of
cartographical preference for placing a label. Lower values mark more desirable
positions.
• Objective function. The objective function (f) measures the quality of the label
placement. The quality of labeling depends on the number of overlaps between
labels and the cartographic preference for label placement.
Figure 1. A set of label positions and their cartographic preference (best = 1;
worse = 4)
We take npos as the number of label positions and take np as the total number of
point features. There are npos
np
possible arrangements, a number which increases
exponentially. Since the set of possible solutions is finite, theoretically we could select
the best solution by enumeration. As the number of points increases this becomes
unfeasible, because of the combinatorial explosion of possible solutions. Marks and
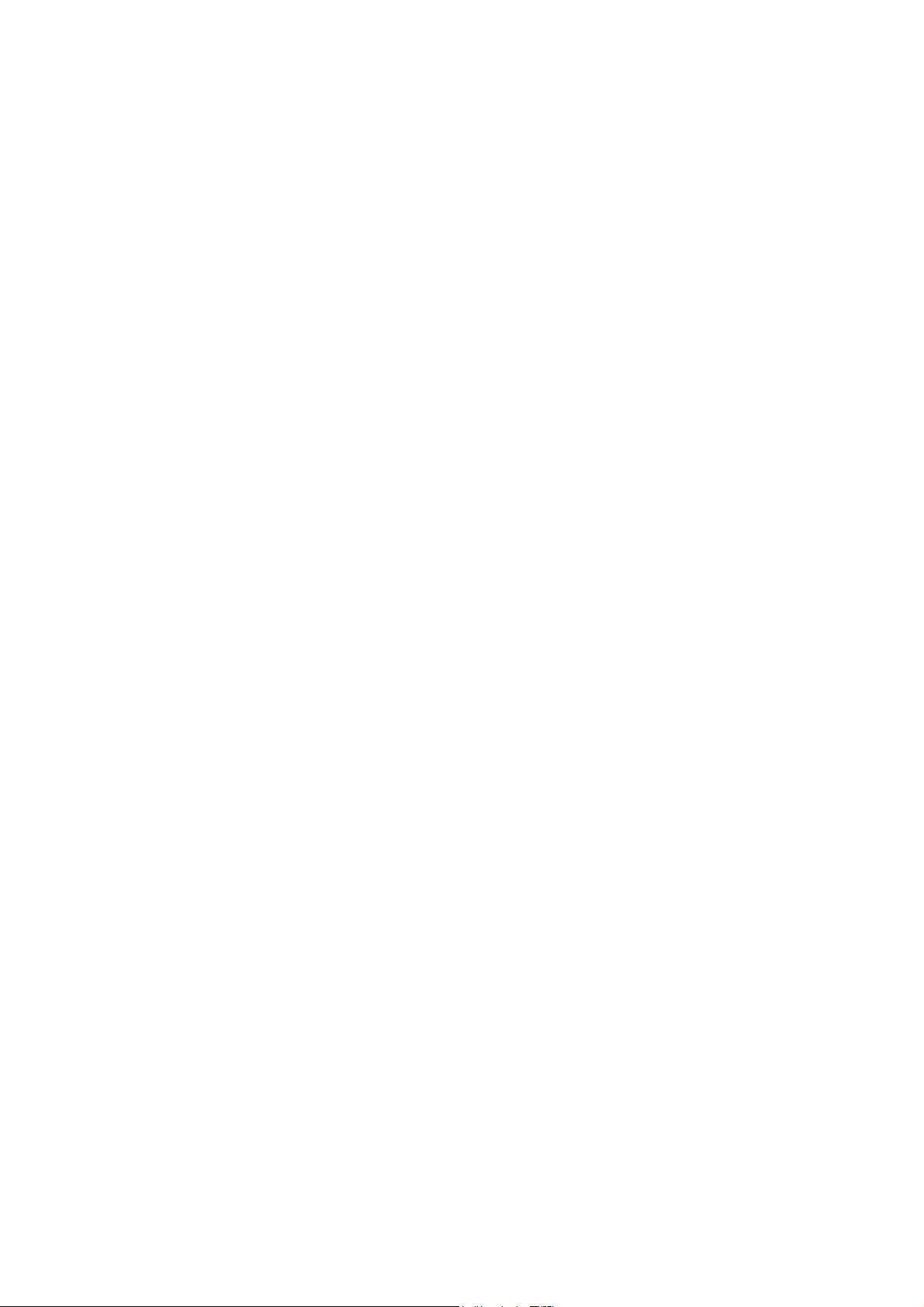
Scheiber (1991) have shown the point feature label placement (PFLP) problem is NP-
hard.
For screen maps, we need algorithms that seek a compromise solution in cost-
benefit, with good quality and a short response time. In our algorithm, we have
considered a limited set of four possible label positions. Our approach can be extended
to account for a larger number of positions. However, a larger set of the label positions
would result in a problem with larger number of possible solutions. In spite of the
apparent better looking results, the growth in problem complexity results in a much
higher computational effort. We consider that a set of four positions to be an acceptable
compromise in terms of cost-benefit analysis.
Several heuristics and metaheuristics have been used for the PFLP problem. For
an early review of methods, see Christensen et al. (1995), which includes Zoraster's
integer programming algorithm (Lagrangean relaxation) (Zoraster 1990) and Hirsch's
continuous gradient-descent algorithm (Hirsch 1982). Relevant recent proposals include
solutions based on simulated annealing (Christensen et al. 1995), genetic algorithms
(GA) (Verner et al. 1997) and tabu search (Yamamoto et al. 2002). The most recent
result is the constructive genetic approach (Yamamoto and Lorena 2005), that has better
results in label placement quality than all previous methods.
In this paper, we will consider the PFLP as the problem of placing labels in all
points. We will try to find out the largest subset of labels with no conflicts with good
quality at acceptable runtime. Our algorithm considers that a typical generation of screen
maps consists of three steps:
• The user requests an overview of the area of interest. The software
displays the area, with a suitable choice of labels depicted at that scale.
• The user requests a zoom in an area for detailed analysis. The software
displays the area, and labels invisible at the largest scale are made visible
at this scale.
• The user may zoom for further detail or pan for visualization of
neighboring areas. In the latter case, more labels will be made visible and
the software will try to display them.
Based on this conception, we consider that label placement on screen maps
needs a preparation stage, where the software will build a list of labels which will be
visible at different scales. This pre-processing phase is outside the scope of this paper.
The proposed algorithm will run at one particular scale, where the visible labels are
known in advance. Therefore, our algorithm should be embedded in a more general
visualization software. In the proposed method, the basic data structure is the conflict
graph, described in the next section.
3. The conflict graph
The conflict graph is a structure that describes conflicts due to label overlap. Each node
of the conflict graph is a label position. Recall from section 2 that we consider a label
position as a pair (point location, relative position). The edges of the graph link
conflicting label positions. Figure 2 shows two points with their label positions and the
剩余14页未读,继续阅读
资源评论
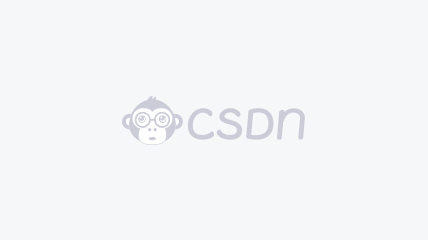

suhebater
- 粉丝: 0
- 资源: 1
上传资源 快速赚钱
我的内容管理 展开
我的资源 快来上传第一个资源
我的收益
登录查看自己的收益我的积分 登录查看自己的积分
我的C币 登录后查看C币余额
我的收藏
我的下载
下载帮助

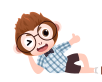
最新资源
- 毕设和企业适用springboot企业安全管理系统类及资源调配管理系统源码+论文+视频.zip
- 毕设和企业适用springboot企业安全管理系统类及智能教育平台源码+论文+视频.zip
- 毕设和企业适用springboot企业财务系统类及3D建模平台源码+论文+视频.zip
- 毕设和企业适用springboot企业财务系统类及仓库管理平台源码+论文+视频.zip
- 毕设和企业适用springboot企业财务系统类及工程管理平台源码+论文+视频.zip
- 毕设和企业适用springboot企业财务系统类及企业健康管理平台源码+论文+视频.zip
- 毕设和企业适用springboot企业财务系统类及全渠道电商平台源码+论文+视频.zip
- 毕设和企业适用springboot企业财务系统类及企业数字化转型平台源码+论文+视频.zip
- 毕设和企业适用springboot企业财务系统类及食品安全追溯平台源码+论文+视频.zip
- 毕设和企业适用springboot企业财务系统类及市场调查平台源码+论文+视频.zip
- 毕设和企业适用springboot企业财务系统类及全生命周期管理平台源码+论文+视频.zip
- 毕设和企业适用springboot企业财务系统类及视频监控系统源码+论文+视频.zip
- 毕设和企业适用springboot企业财务系统类及物流信息平台源码+论文+视频.zip
- 毕设和企业适用springboot企业财务系统类及业务流程自动化平台源码+论文+视频.zip
- 毕设和企业适用springboot企业财务系统类及智慧物流管理平台源码+论文+视频.zip
- 毕设和企业适用springboot企业财务系统类及智能电商平台源码+论文+视频.zip
资源上传下载、课程学习等过程中有任何疑问或建议,欢迎提出宝贵意见哦~我们会及时处理!
点击此处反馈


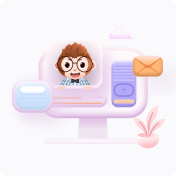
安全验证
文档复制为VIP权益,开通VIP直接复制
