%%%%%%%%%%%%%%%%%%%%%%%%%%%%%%%%%%%%%%%%%%%%%
%功能:演示蚁群算法在计算机视觉中的应用
%基于蚁群算法实现路径规划;
%环境:Win7,Matlab2018a
%Modi: C.S
%时间:2022-04-05
%%%%%%%%%%%%%%%%%%%%%%%%%%%%%%%%%%%%%%%%%%%%%
function [Alocation,Newbest,traceInfo]=aca_ant_colony_system %(InitOps);
%%%%%%%%%%%%%%%%%%%%%%%%%%%%%%%%%%%%%%%%%%%%%%%%%%%%%%%%%%%%%%
%% 蚁群算法初始化程序开始 %%
%%%%%%%%%%%%%%%%%%%%%%%%%%%%%%%%%%%%%%%%%%%%%%%%%%%%%%%%%%%%%%
%%%%%%%%%% 问题定义:获取城市位置坐标、计算距离矩阵 %%%%%%%%%
InitOps=[];
[Location,DistMatrix,Ncities,Bestx,Lengx] = pr76init(InitOps);
close all;
figure (1);
hold on;
minx=min(DistMatrix(:,1));
maxx=max(DistMatrix(:,1));
miny=min(DistMatrix(:,2));
maxy=max(DistMatrix(:,2));
minm=min(minx,miny);
maxm=max(maxx,maxy);
l=(maxm-minm)/10;
for i=1:Ncities
plot(Location(i,1),Location(i,2),'*b');
text (Location(i,1)+l,Location(i,2)+l,num2str(i));
end
for i=1:Ncities-1
line([Location(Bestx(i),1),Location(Bestx(i+1),1)] , [Location(Bestx(i),2),Location(Bestx(i+1),2)]) ;
end
line([Location(Bestx(1),1),Location(Bestx(Ncities),1)] , [Location(Bestx(1),2),Location(Bestx(Ncities),2)]) ;
grid on,title(['初始路线图-',num2str(Lengx)]),xlabel('横坐标'),ylabel('纵坐标');
legend('城市位置');
hold off ;
% 初始化随机发生器状态
rand('state',sum(100*clock));
% ================================================
% 使用最近邻法构造一个初始游历,并据此计算信息系初值
p=zeros(1,Ncities+1);
p(1)=round(Ncities*rand+0.5);% p存储目前找到的所有城市的编号
i=p(1);
count=2;
while count <= Ncities
NNdist= inf ;%NNdist存储目前找到的和当前城市距离最短的城市的距离
pp= i ;% i存储当前城市的编号 pp存储目前找到的城市编号
for j= 1: Ncities
if (DistMatrix(i, j) < NNdist) & (j~=i) & ((j~=p) == ones(1,length(p)))
% 目标城市的要求为--距离短、且不能是当前城市,也不能是以前已经走过的城市
NNdist= DistMatrix(i, j) ;
pp= j ;
end
end
p(count)=pp;
i= pp ;
count= count + 1 ;
end
p=AcatspEval(p,DistMatrix,Ncities);
len=p(1,Ncities+1);
Q0=1/(Ncities*len);
%%%%%%%%%% 设定系统有关参数 %%%%%%%%%%
MaxNc=5000;% 最大代数
A=1;% 信息素因子
B=2;% 启发信息因子
P1=0.1;% 局部挥发系数初值
P2=0.1;% 全局挥发系数初值
R0=0.9; %选择概率
M=10;% 蚂蚁数量
%%%%%%%%%% 初始化信息素、启发信息矩阵、确定蚂蚁最初位置及允许矩阵 %%%%%%%%%%
Pheromone=Q0*ones(Ncities,Ncities);% 信息素初始矩阵;
Heuristic=1./DistMatrix;% 启发信息初始矩阵
Temp=ones(1,Ncities);
Heuristic=1./(1./Heuristic+diag(Temp));
RandL=round(rand(M,1)*Ncities+0.5);%蚂蚁最初位置
Alocation0=zeros(M,Ncities+1);% 存放M+1个蚂蚁游历的路径及长度矩阵初始化
Alocation0(:,1)=RandL;
Allow0=repmat(1:Ncities,M,1);% 允许访问的城市矩阵初始化
for Ak=1:M
Allow0(Ak,RandL(Ak))=0;
end
%%%%%%%%%% 运行参数初始化 %%%%%%%%%%
Nc=1;% 第一代
Lbestdis=inf;
Cbestdis=inf;
Fnewbest=0;
Alocation=Alocation0;% 存放个蚂蚁游历的路径及长度矩阵初始化
Allow=Allow0; % 允许矩阵赋初值
t1=clock;
%%%%%%%%%%%%%%%%%%%%%%%%%%%%%%%%%%%%%%%%%%%%%%%%%%%%%%%%%%%%%%
%% 蚁群算法初始化程序结束 %%
%%%%%%%%%%%%%%%%%%%%%%%%%%%%%%%%%%%%%%%%%%%%%%%%%%%%%%%%%%%%%%
%%%%%%%%%%%%%%%%%%%%%%%%%%%%%%%%%%%%%%%%%%%%%%%%%%%%%%%%%%%%%%
%% 蚁群算法主循环开始 %%
%%%%%%%%%%%%%%%%%%%%%%%%%%%%%%%%%%%%%%%%%%%%%%%%%%%%%%%%%%%%%%
while(Nc<=MaxNc)
% M个蚂蚁选择Ncities个城市
for Cityi=2:Ncities+1
if Cityi<Ncities+1
for Ak=1:M
i=Alocation(Ak,Cityi-1);% 当前城市
j=Select_for_aca(R0,Ak,i,Allow,A,B,Pheromone,Heuristic);% 依据Pij选择下一个城市j
Alocation(Ak,Cityi)=j;
Allow(Ak,j)=0;% 更新允许矩阵
Pheromone(i,j)=(1-P1)*Pheromone(i,j)+P1*Q0; % 信息素在线单步更新
Pheromone(j,i)= Pheromone(i,j);
end
else % 返回出发城市
for Ak=1:M
i=Alocation(Ak,Cityi-1);% 当前城市
j=Alocation(Ak,1);
Pheromone(i,j)=(1-P1)*Pheromone(i,j)+P1*Q0; % 信息素在线单步更新
Pheromone(j,i)=Pheromone(i,j);
end
end
end
% 计算每个蚂蚁找到的路径的长度
for Ak=1:M
Alocation(Ak,:)=AcatspEval(Alocation(Ak,:),DistMatrix,Ncities);% 计算适应值即每个蚂蚁找到的路径长度
end
% 保存最优、最差解;
t2=clock;
t=etime(t2,t1);
[Cbestdis,Am]=min(Alocation(1:M,Ncities+1));
Cbest=Alocation(Am,:);
% 给输出变量赋值
traceInfo(Nc,1)=Nc; %current generation
traceInfo(Nc,2)= Cbest(Ncities+1); %Best fittness
traceInfo(Nc,3)=mean(Alocation(:,Ncities+1)); %Avg fittness
traceInfo(Nc,4)=std(Alocation(:,Ncities+1)); %计算标准方差
if (Cbestdis<Lbestdis)||(Nc==1)
Fnewbest=Fnewbest+1;
Newbest(Fnewbest,1)=Nc;
Newbest(Fnewbest,2)=t;
Newbest(Fnewbest,3:Ncities+3)=Cbest;
Lbest=Cbest;
Lbestdis=Cbest(Ncities+1);
end
% 信息素离线全局更新
Iindex=Lbest(1:Ncities);
Jindex=[Lbest(2:Ncities) Lbest(1)];
for k=1:Ncities
Pheromone(Iindex(k),Jindex(k))=(1-P1)*Pheromone(Iindex(k),Jindex(k))+P2*(1./Lbest(Ncities+1));%对最优路径上的信息素增加
Pheromone(Jindex(k),Iindex(k))=Pheromone(Iindex(k),Jindex(k));
end
RandL=Alocation(:,Ncities);
Alocation=zeros(M,Ncities+1);% 存放M+1个蚂蚁游历的路径及长度矩阵初始化
Alocation(:,1)=RandL;
Allow=repmat(1:Ncities,M,1);% 允许访问的城市矩阵初始化
for Ak=1:M
Allow(Ak,RandL(Ak))=0;
end
Nc=Nc+1;
end
%%%%%%%%%%%%%%%%%%%%%%%%%%%%%%%%%%%%%%%%%%%%%%%%%%%%%%%%%%%%%%
%% 蚁群算法主循环结束 %%
%%%%%%%%%%%%%%%%%%%%%%%%%%%%%%%%%%%%%%%%%%%%%%%%%%%%%%%%%%%%%%
%%%%%%%%%%%%%%%%%%%%%%%%%%%%%%%%%%%%%%%%%%%%%%%%%%%%%%%%%%%%%
%% 蚁群算法输出模块开始 %%
%%%%%%%%%%%%%%%%%%%%%%%%%%%%%%%%%%%%%%%%%%%%%%%%%%%%%%%%%%%%%%
%%%%%%%%%%%%%%%%%%%%%%%%%%%%%%%%
%% 以图形方式显示运行结果开始 %%
%figure(1);
%hold on;
%plot ( traceInfo (: , 1) , traceInfo (: , 2) ) ;
%grid on,title('离线性能'),xlabel('代数'),ylabel('最优个体适应值');
%legend('最优个体适应值');
%hold off;
%figure(2);
%hold on;
%plot( traceInfo (: , 1) , traceInfo (: , 3) ) ;
%grid on,title('在线性能'),xlabel('代数'),ylabel('平均适应值');
%legend('平均适应值');
%hold off;
figure(2);
hold on;
plot( Newbest (: , 1) , Newbest (: , Ncities+3) ) ;
grid on,title('全局最优解变化'),xlabel('代数'),ylabel('全局最优解适应度');
legend('全局最优解适应度');
hold off;
figure (3);
Gbest=Newbest(Fnewbest,:);
hold on;
minx=min(DistMatrix(:,1));
maxx=max(DistMatrix(:,1));
miny=min(DistMatrix(:,2));
maxy=max(DistMatrix(:,2));
minn=min(minx,miny);
maxx=max(maxx,maxy);
l=(maxx-minn)/100;
for i=1:Ncities
plot(Location(i,1),Location(i,2),'*b');
text (Location(i,1)+l,Location(i,2)+l,num2str(i));
end
for i=3:Ncities+1
line([Location(Gbest(i),1),Location(Gbest(i+1),1)] , [Location(Gbest(i),2),Location(Gbest(i+1),2)]) ;
end
line([Location(Gbest(3),1),Location(Gbest(Ncities+2),1)] , [Location(Gbest(3),2),Location(Gbest(Ncities+2),2)]) ;
grid on,title(['全局最优解路线图-',num2str(Lbestdis)]),xlabel('横坐标'),ylabel('纵坐标');
legend('城市位置');
hold off ;
%% 以图形方式显示运行结果开始 %%
%%%%%%%%%%%%%%%%%%%%%%%%%%%%%%%%
没有合适的资源?快使用搜索试试~ 我知道了~
温馨提示
计算机视觉-蚁群算法MATLAB源码 蚁群算法是一种用来寻找优化路径的概率型算法。它由Marco Dorigo于1992年在他的博士论文中提出,其灵感来源于蚂蚁在寻找食物过程中发现路径的行为。 这种算法具有分布计算、信息正反馈和启发式搜索的特征,本质上是进化算法中的一种启发式全局优化算法。 蚁群系统(Ant System或Ant Colony System)是由意大利学者Dorigo、Maniezzo等人于20世纪90年代首先提出来的。他们在研究蚂蚁觅食的过程中,发现单个蚂蚁的行为比较简单,但是蚁群整体却可以体现一些智能的行为。例如蚁群可以在不同的环境下,寻找最短到达食物源的路径。这是因为蚁群内的蚂蚁可以通过某种信息机制实现信息的传递。后又经进一步研究发现,蚂蚁会在其经过的路径上释放一种可以称之为“信息素”的物质,蚁群内的蚂蚁对“信息素”具有感知能力,它们会沿着“信息素”浓度较高路径行走,而每只路过的蚂蚁都会在路上留下“信息素”,这就形成一种类似正反馈的机制,这样经过一段时间后,整个蚁群就会沿着最短路径到达食物源了。
资源详情
资源评论
资源推荐
收起资源包目录


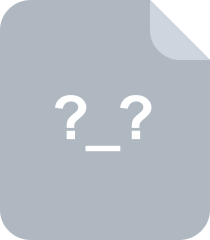
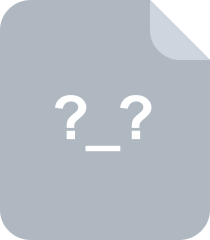
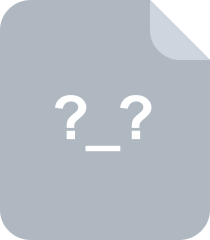
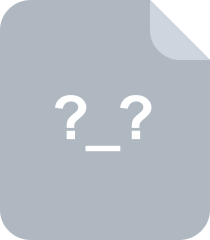
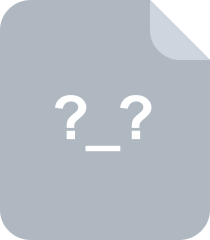
共 5 条
- 1
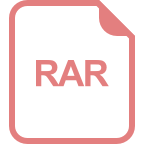
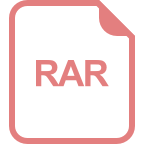
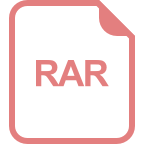
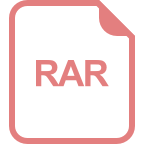
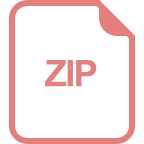
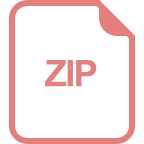
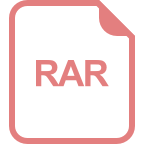
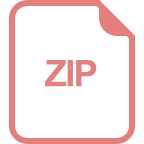
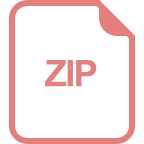
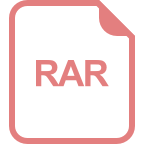
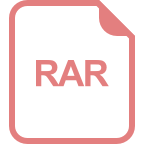
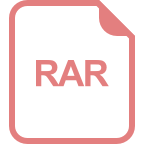
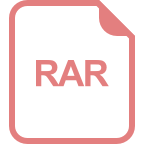
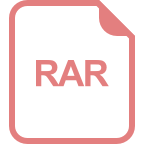
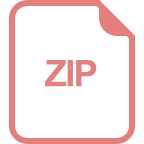
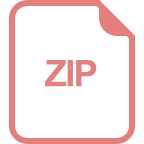
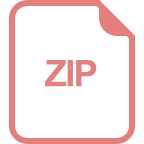
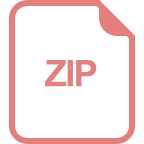

mozun2020
- 粉丝: 1w+
- 资源: 131
上传资源 快速赚钱
我的内容管理 展开
我的资源 快来上传第一个资源
我的收益
登录查看自己的收益我的积分 登录查看自己的积分
我的C币 登录后查看C币余额
我的收藏
我的下载
下载帮助

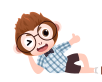
最新资源
- 探索高维数据可视化:技术、实践与代码示例
- 基于java swing+jdbc+mysql实现的超市购物管理系统实习报告.docx
- 控制ppt图案填充透明度,极大增加ppt的显示效果
- 递推平均滤波法是一种简单而有效的滤波方法,通过计算一段时间内的数据平均值来平滑数据,达到滤波的目的
- 关闭浏览器跨域启动脚本chrome.bat
- JDK Development Kit 17.0.13 downloads官方下载
- TIA PORTAL V19硬件支持包HSP(2024.10最新).txt
- 卡西欧手表GA-100(5081)中文使用手册
- WINCC(虚拟机)PC1与博途(虚拟机)PC2通讯(虚拟PLC装在PC1主机上)
- 【源码+数据库】基于ssm框架+mysql实现的学生选课信息管理系统
资源上传下载、课程学习等过程中有任何疑问或建议,欢迎提出宝贵意见哦~我们会及时处理!
点击此处反馈


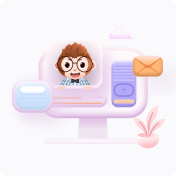
安全验证
文档复制为VIP权益,开通VIP直接复制

评论0