# 卷积神经网络结构可视化
- 简介
- 本文介绍的工具是针对卷积神经网络示意图可视化的,不包括算图。(示意图一般出现在论文中)
- 常见的卷积神经网络示意图绘制工具不少,常用的主要有 NN SVG、ConvNetDraw、PlotNeuralNet 等。
- 写这篇重点介绍 PlotNeuralNet 的教程的原因是国内关于它的教程很少并且大都只是列举了官方 demo。
- 常见工具
- NN SVG
- [官方地址](http://alexlenail.me/NN-SVG/)
- 
- 过去一段时间内比较喜欢用的,特点是很方便,提供给用户的是个交互式的 Web 页面。
- 特点
- 方便,各层直接界面控制增减及变化。
- 支持三种风格,选择空间大。
- 支持 SVG 格式下载。
- 缺点
- 可视化界面的最大问题就是很多用户期待的功能为考虑全面,定制程度低。
- 各层连接不是很好看。
- ConvNetDraw
- [官方地址](https://cbovar.github.io/ConvNetDraw/)
- 
- 从未使用过,很多博主推荐,但是观感劝退了我。
- 特点
- 脚本化控制
- 尺度自定义
- 直观
- 缺点
- 既没有做到脚本化的自由度,又没有做到交互界面的观感。
- 不好看。
- PlotNeuralNet
- 官方地址
- [https://github.com/HarisIqbal88/PlotNeuralNet.git](https://github.com/HarisIqbal88/PlotNeuralNet.git)
- 
- 这是我极力推荐的工具,尽管它的上手难度略高于之前两个,但学会之后很好用,不少论文就是使用这个工具可视化的。
- 特点
- 脚本化,使用 LaTex 编写或者使用 Python 脚本编写结构模型,自由度高。
- 缺点
- 无交互界面,上手略有难度。
- 使用教程
- 说明
- 基于 Linux 或者有 bash 的环境,我只在 Ubuntu 系统下测试成功。(事实上,深度学习首选的环境之一就是 Linux 的 Ubuntu)
- 只介绍 Python 脚本绘制的方式,不介绍 LaTex 方式。
- 需要安装前置软件,如 LaTex 解析器。
- 前置准备
- 安装 textlive
- 调用 LaTex 解析生成 PDF,需要安装 LaTex,这里使用 TextLive。
- `wget https://mirrors.tuna.tsinghua.edu.cn/CTAN/systems/texlive/Images/texlive2019.iso`
- 上面命令为下载镜像到当前目录,失败则可能换源,去掉上述链接的最后文件,在 Web 中查看合适文件下载即可。
- `sudo apt-get install perl-tk`
- 安装图形界面
- `sudo mount -o loop texlive.iso /mnt`
- 挂载镜像
- `cd /mnt`
- 切换到挂载目录
- `sudo ./install-tl -gui`
- 使用图形界面安装
- `sudo apt-get install texlive-latex-extra`
- 安装扩展包
- 下载源码
- `git clone https://github.com/HarisIqbal88/PlotNeuralNet.git`
- 源码目录结构
- 
- 其中 pycore 下的 tikzeng.py 是核心文件,定义了绘图过程(所有 to 开头的函数),可以绘制的层,py 脚本向 LaTex 的转换。
- 代码比较易懂,这里不做解析了。
- 绘制
- 一般将自己写的 py 脚本放在 clone 的项目的 pyexamples 目录下。
- 源目录下有两个 py 脚本,对其进行详细注释,包含了常用的语法。
- 代码 1-test_simple.py
- cd 到 pyexamples 目录执行 `bash ../tikzmake.sh test_simple`(**注意不加 py 后缀,且有些错误正常,观察是否生成 pdf 文件即可**)
- ```python
import sys
sys.path.append('../') # 添加自定义库的目录
from pycore.tikzeng import * # 导入自定义库
# defined your arch
arch = [
# 添加头
to_head( '..' ),
to_cor(),
to_begin(),
# 添加卷积层conv1
to_Conv("conv1", 512, 64, offset="(0,0,0)", to="(0,0,0)", height=64, depth=64, width=2 ),
# 卷积层conv1东侧添加池化层pool1
to_Pool("pool1", offset="(0,0,0)", to="(conv1-east)"),
# 池化层pool1东侧添加卷积层conv2
to_Conv("conv2", 128, 64, offset="(1,0,0)", to="(pool1-east)", height=32, depth=32, width=2 ),
# 建立pool1到conv2的连接箭头
to_connection( "pool1", "conv2"),
# conv2东侧添加pool2
to_Pool("pool2", offset="(0,0,0)", to="(conv2-east)", height=28, depth=28, width=1),
# pool1东侧添加softmax层但是偏移3单位
to_SoftMax("soft1", 10 ,"(3,0,0)", "(pool1-east)", caption="SOFT" ),
# 建立pool2到soft1的连接箭头
to_connection("pool2", "soft1"),
# 结束
to_end()
]
def main():
namefile = str(sys.argv[0]).split('.')[0]
to_generate(arch, namefile + '.tex' )
if __name__ == '__main__':
main()
```
- 执行结果
- 
- 代码 2-unet.py
- ```python
import sys
sys.path.append('../')
from pycore.tikzeng import *
from pycore.blocks import *
arch = [
# 开头
to_head('..'),
to_cor(),
to_begin(),
# 添加输入层
to_input( '../examples/fcn8s/cats.jpg' ),
# 添加block1包含一个二重卷积接relu
to_ConvConvRelu( name='ccr_b1', s_filer=500, n_filer=(64,64), offset="(0,0,0)", to="(0,0,0)", width=(2,2), height=40, depth=40 ),
to_Pool(name="pool_b1", offset="(0,0,0)", to="(ccr_b1-east)", width=1, height=32, depth=32, opacity=0.5),
# 添加三个block,每个包含三个二卷积加一池化
*block_2ConvPool( name='b2', botton='pool_b1', top='pool_b2', s_filer=256, n_filer=128, offset="(1,0,0)", size=(32,32,3.5), opacity=0.5 ),
*block_2ConvPool( name='b3', botton='pool_b2', top='pool_b3', s_filer=128, n_filer=256, offset="(1,0,0)", size=(25,25,4.5), opacity=0.5 ),
*block_2ConvPool( name='b4', botton='pool_b3', top='pool_b4', s_filer=64, n_filer=512, offset="(1,0,0)", size=(16,16,5.5), opacity=0.5 ),
# 瓶颈,为block5
to_ConvConvRelu( name='ccr_b5', s_filer=32, n_filer=(1024,1024), offset="(2,0,0)", to="(pool_b4-east)", width=(8,8), height=8, depth=8, caption="Bottleneck" ),
to_connection( "pool_b4", "ccr_b5"),
# 解码器
# 多个block,每个为unconv
*block_Unconv( name="b6", botton="ccr_b5", top='end_b6', s_filer=64, n_filer=512, offset="(2.1,0,0)", size=(16,16,5.0), opacity=0.5 ),
to_skip( of='ccr_b4', to='ccr_res_b6', pos=1.25),
*block_Unconv( name="b7", botton="end_b6", top='end_b7', s_filer=128, n_filer=256, offset="(2.1,0,0)", size=(25,25,4.5), opacity=0.5 ),
to_skip( of='ccr_b3', to='ccr_res_b7', pos=1.25),
*block_Unconv( name="b8", botton="end_b7", top='end_b8', s_filer=256, n_filer=128, offset="(2.1,0,0)", size=(32,32,3.5), opacity=0.5 ),
to_skip( of='ccr_b2', to='ccr_res_b8', pos=1.25),
*block_Unconv( name="b9", botton="end_b8", top='end_b9', s_filer=512, n_filer=64, offset="(2.1,0,0)", size=(40,40,2.5),
没有合适的资源?快使用搜索试试~ 我知道了~
温馨提示
本文介绍的工具是针对卷积神经网络示意图可视化的,不包括算图。(示意图一般出现在论文中) 常见的卷积神经网络示意图绘制工具不少,常用的主要有 NN SVG、ConvNetDraw、PlotNeuralNet 等。 写这篇重点介绍 PlotNeuralNet 的教程的原因是国内关于它的教程很少并且大都只是列举了官方 demo。 详细介绍参考:https://blog.csdn.net/newlw/article/details/132507741
资源推荐
资源详情
资源评论
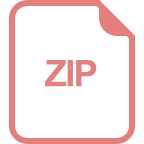
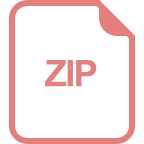
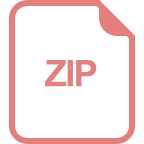
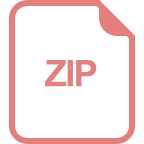
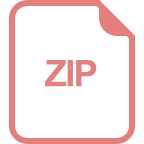
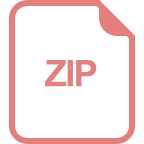
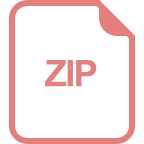
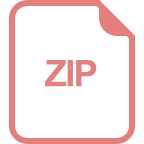
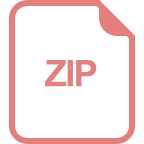
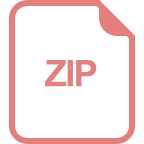
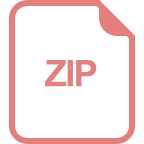
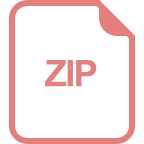
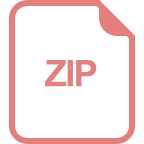
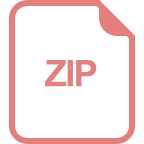
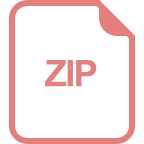
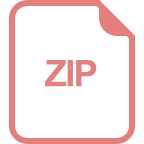
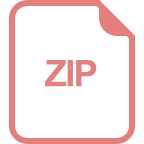
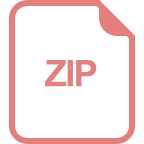
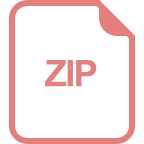
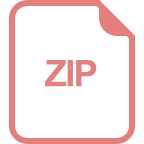
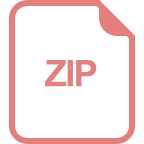
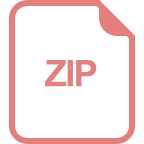
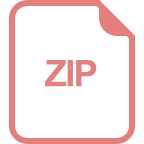
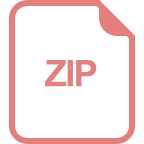
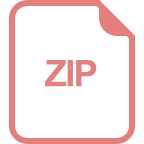
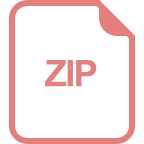
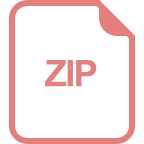
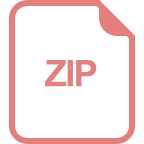
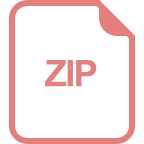
收起资源包目录



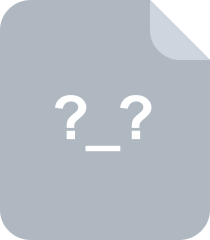


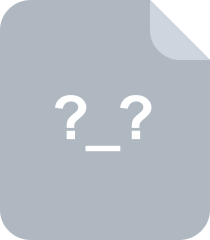
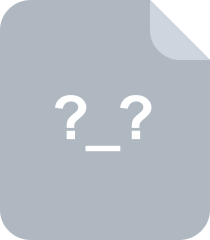
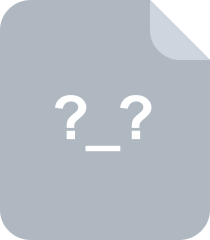
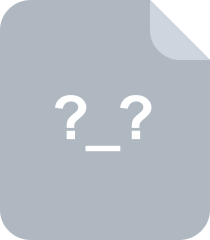


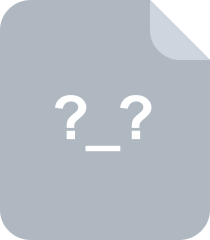
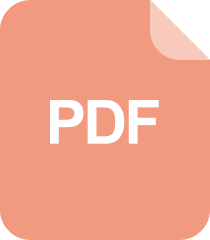
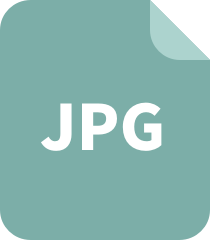

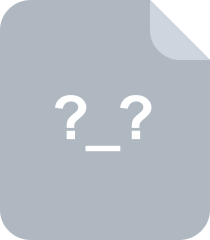
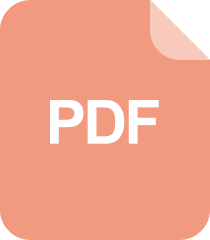

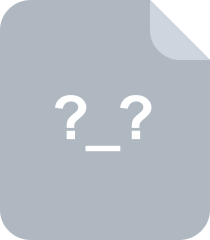
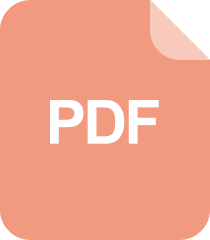

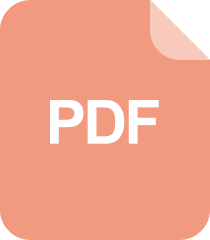
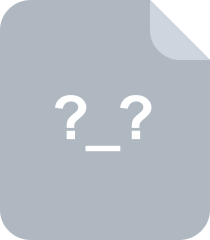

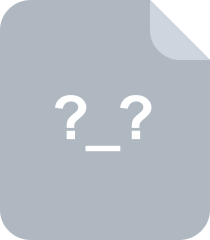
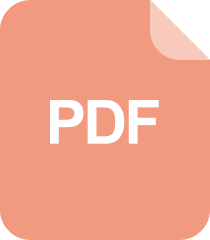

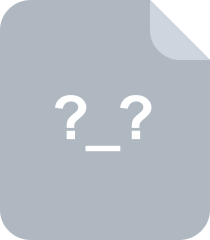
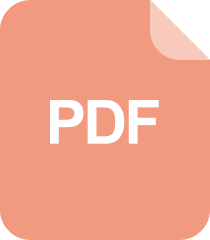

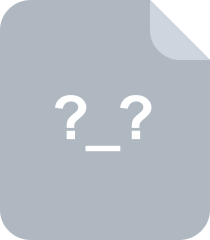
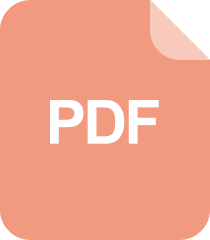

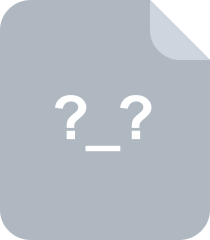
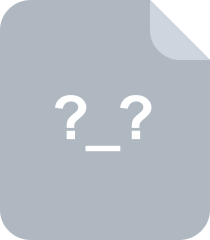
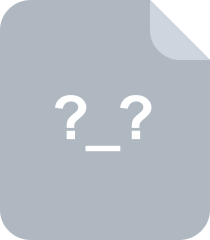
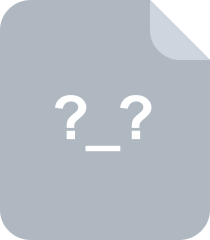
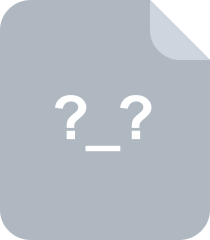

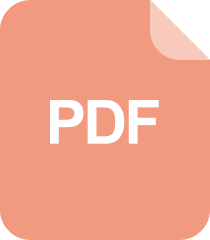
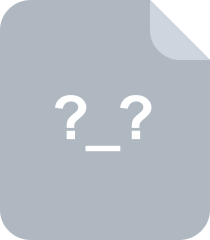
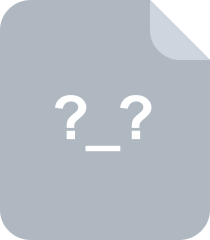
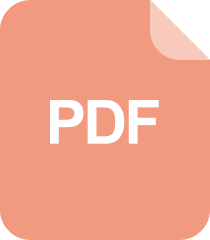
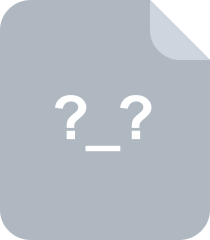
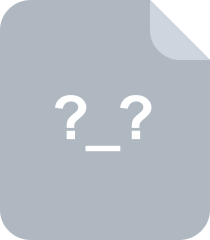
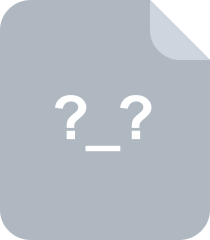
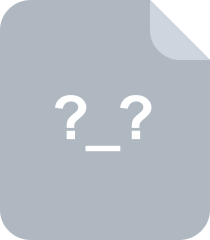
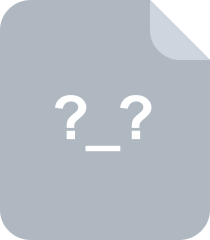
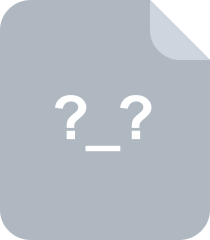
共 35 条
- 1
资源评论
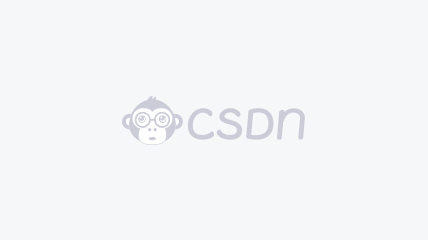

shejizuopin
- 粉丝: 1w+
- 资源: 1300
上传资源 快速赚钱
我的内容管理 展开
我的资源 快来上传第一个资源
我的收益
登录查看自己的收益我的积分 登录查看自己的积分
我的C币 登录后查看C币余额
我的收藏
我的下载
下载帮助

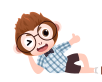
最新资源
资源上传下载、课程学习等过程中有任何疑问或建议,欢迎提出宝贵意见哦~我们会及时处理!
点击此处反馈


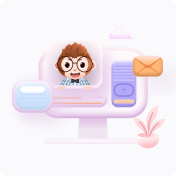
安全验证
文档复制为VIP权益,开通VIP直接复制
